Identifying Heart Failure from Electronic Health Records: A Systematic Evidence Review
medRxiv(2022)
摘要
Background Heart failure (HF) is a complex syndrome associated with significant morbidity, mortality, and healthcare costs. Electronic health records (EHRs) are widely used to identify patients with HF. Despite the development of many HF phenotyping algorithms, it is unclear if the characteristics of identified populations reflect the known spectrum of disease in HF patients.
Methods We performed a systematic evidence review to assess the methods used for HF algorithm development from US based data sources and the relevant information facilitating their application to research and clinical care. We queried PubMed for articles published up to November 2021. Out of 428 studies screened, 35 articles were included for primary analysis and 23 studies using only International Classification of Diseases (ICD) codes were evaluated for secondary analysis. Results are reported descriptively.
Results HF algorithms were most often developed at academic medical centers, though these were not evenly distributed across the US. HF and congestive HF were the most frequent labels for observed phenotypes. Diagnoses were the most common data type used to identify HF patients and echocardiography was the second most frequent. Most studies used rule-based algorithms. Validation of algorithms varied considerably with 36% of HF subtype algorithms validated and 67% of acute HF algorithms validated. Demographics of any study population were reported in 57% of algorithm studies and 70% of ICD-only studies, however fewer than half (40% of algorithm and 46% of ICD-only) of studies reported demographics of the HF population identified by their computable phenotype. Of those reporting, most identified majority (>50%) male populations, including both algorithms for HF with preserved ejection fraction identified in this study.
Conclusion There is significant heterogeneity in phenotyping algorithms used to ascertain HF from EHRs. Validation of algorithms is inconsistent and largely relies on manual review of medical records. The concentration of algorithm development at a few sites limits their generalizability to identify HF patients in other settings. Differences between the reported demographics of algorithm-identified HF populations and those expected based on HF epidemiology suggest that current algorithms do not reflect the full spectrum of HF in the general population.
### Competing Interest Statement
The authors have declared no competing interest.
### Funding Statement
LKW was supported by the National Library of Medicine (NIH - K01LM013088) and institutional support from the Division of Biomedical Informatics and Personalized Medicine. LVR was supported by NHGRI grants U01HG008673-01 and NIGMS grant 2R01GM105688.
### Author Declarations
I confirm all relevant ethical guidelines have been followed, and any necessary IRB and/or ethics committee approvals have been obtained.
Yes
The details of the IRB/oversight body that provided approval or exemption for the research described are given below:
This study is a systematic evidence review that does not include human subjects and thus is exempt from IRB review.
I confirm that all necessary patient/participant consent has been obtained and the appropriate institutional forms have been archived, and that any patient/participant/sample identifiers included were not known to anyone (e.g., hospital staff, patients or participants themselves) outside the research group so cannot be used to identify individuals.
Yes
I understand that all clinical trials and any other prospective interventional studies must be registered with an ICMJE-approved registry, such as ClinicalTrials.gov. I confirm that any such study reported in the manuscript has been registered and the trial registration ID is provided (note: if posting a prospective study registered retrospectively, please provide a statement in the trial ID field explaining why the study was not registered in advance).
Yes
I have followed all appropriate research reporting guidelines and uploaded the relevant EQUATOR Network research reporting checklist(s) and other pertinent material as supplementary files, if applicable.
Yes
Data will be made available at the time of publication.
更多查看译文
AI 理解论文
溯源树
样例
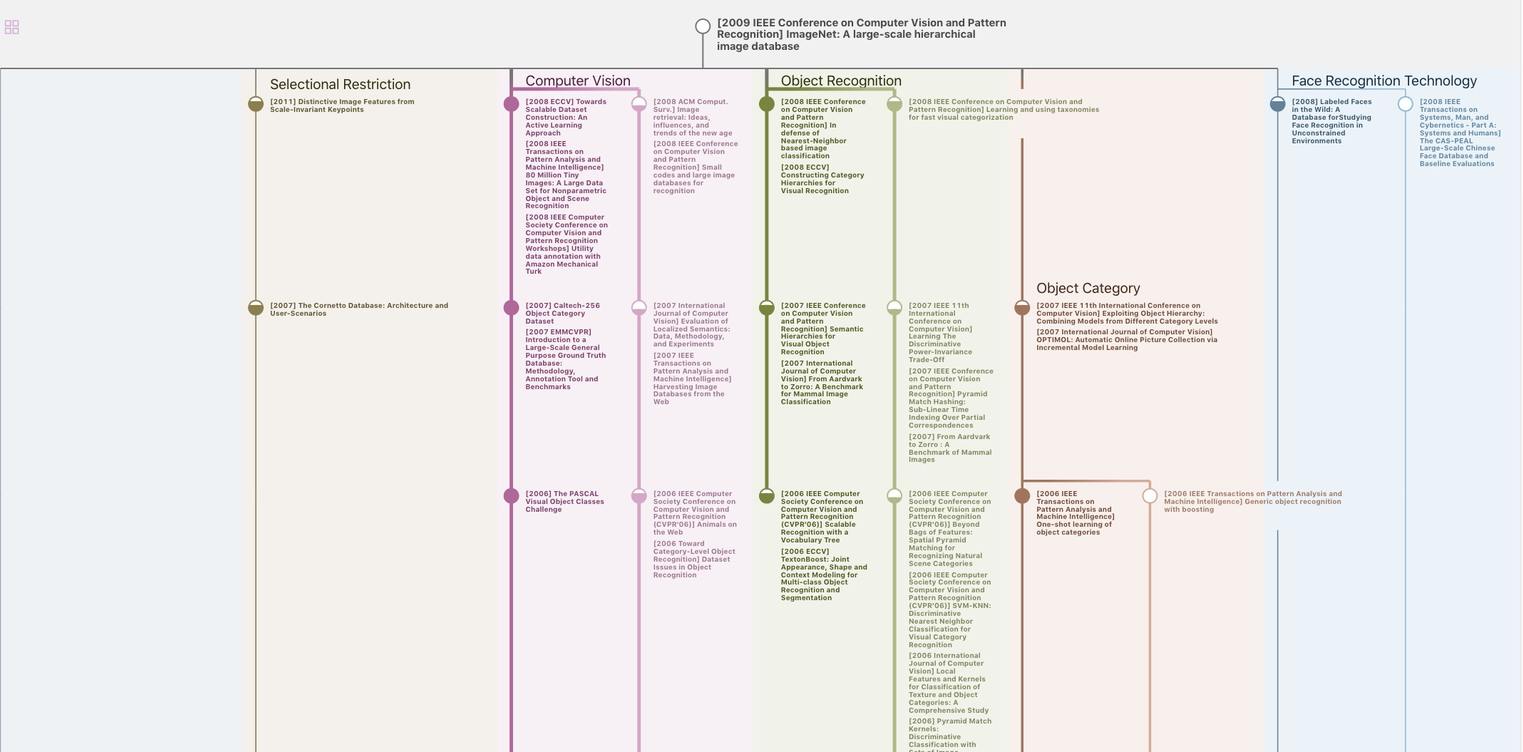
生成溯源树,研究论文发展脉络
Chat Paper
正在生成论文摘要