LSTM-SNP: A long short-term memory model inspired from spiking neural P systems
Knowledge-Based Systems(2022)
Abstract
Spiking neural P (SNP) systems are a class of neural-like membrane computing models that are abstracted by applying the mechanisms of spiking neurons. In SNP systems, each spiking neuron has three characteristics: (i) internal state, (ii) spike consumption, and (iii) spike generation. These three characteristics are used to form a parameterised nonlinear SNP system, which has a nonlinear spiking mechanism, three nonlinear gate functions, and trainable parameters. Based on the parameterised nonlinear SNP system, we develop a novel variant of long short-term memory (LSTM), called the LSTM-SNP model. LSTM-SNP is a recurrent-type model that can process sequential data. Time series forecasting problems are used to conduct a case study. Five benchmark time series are used to evaluate the proposed LSTM-SNP model and compare seven state-of-the-art prediction models and five baseline prediction models. The comparison results show the effectiveness of the proposed LSTM-SNP model for time series forecasting.
MoreTranslated text
Key words
Spiking neural P systems,Spiking neurons,Long short-term memory,Time series forecasting
AI Read Science
Must-Reading Tree
Example
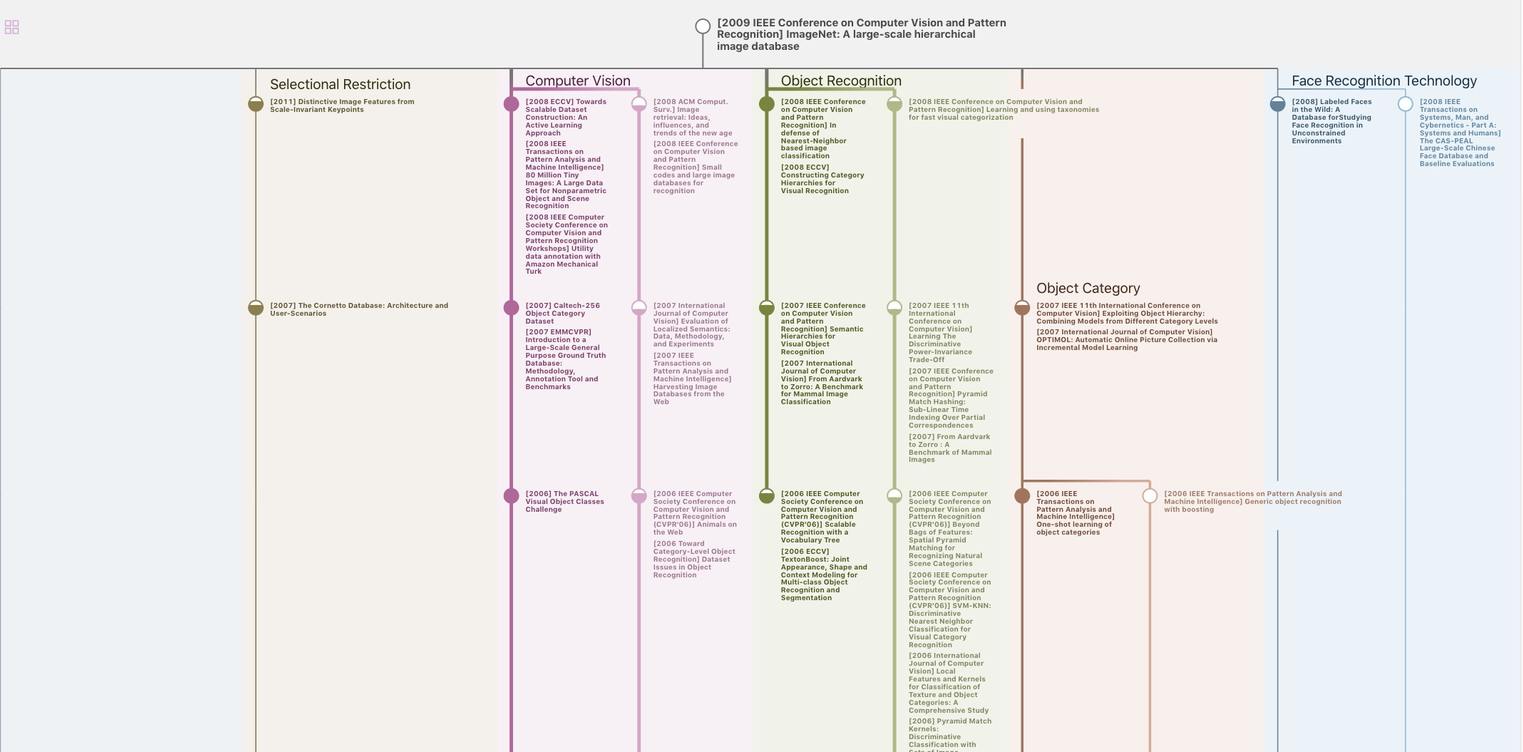
Generate MRT to find the research sequence of this paper
Chat Paper
Summary is being generated by the instructions you defined