Discriminative deep attributes for generalized zero-shot learning
Pattern Recognition(2022)
摘要
We indirectly predict a class by deriving user-defined (i.e., existing) attributes (UA) from an image in generalized zero-shot learning (GZSL). High-quality attributes are essential for GZSL, but the existing UAs are sometimes not discriminative. We observe that the hidden units at each layer in a convolutional neural network (CNN) contain highly discriminative semantic information across a range of objects, parts, scenes, textures, materials, and color. The semantic information in CNN features is similar to the attributes that can distinguish each class. Motivated by this observation, we employ CNN features like novel class representative semantic data, i.e., deep attribute (DA). Precisely, we propose three objective functions (e.g., compatible, discriminative, and intra-independent) to inject the fundamental properties into the generated DA. We substantially outperform the state-of-the-art approaches on four challenging GZSL datasets, including CUB, FLO, AWA1, and SUN. Furthermore, the existing UA and our proposed DA are complementary and can be combined to enhance performance further.
更多查看译文
关键词
Generalized zero-shot learning,Deep attribute,Discriminative latent attribute
AI 理解论文
溯源树
样例
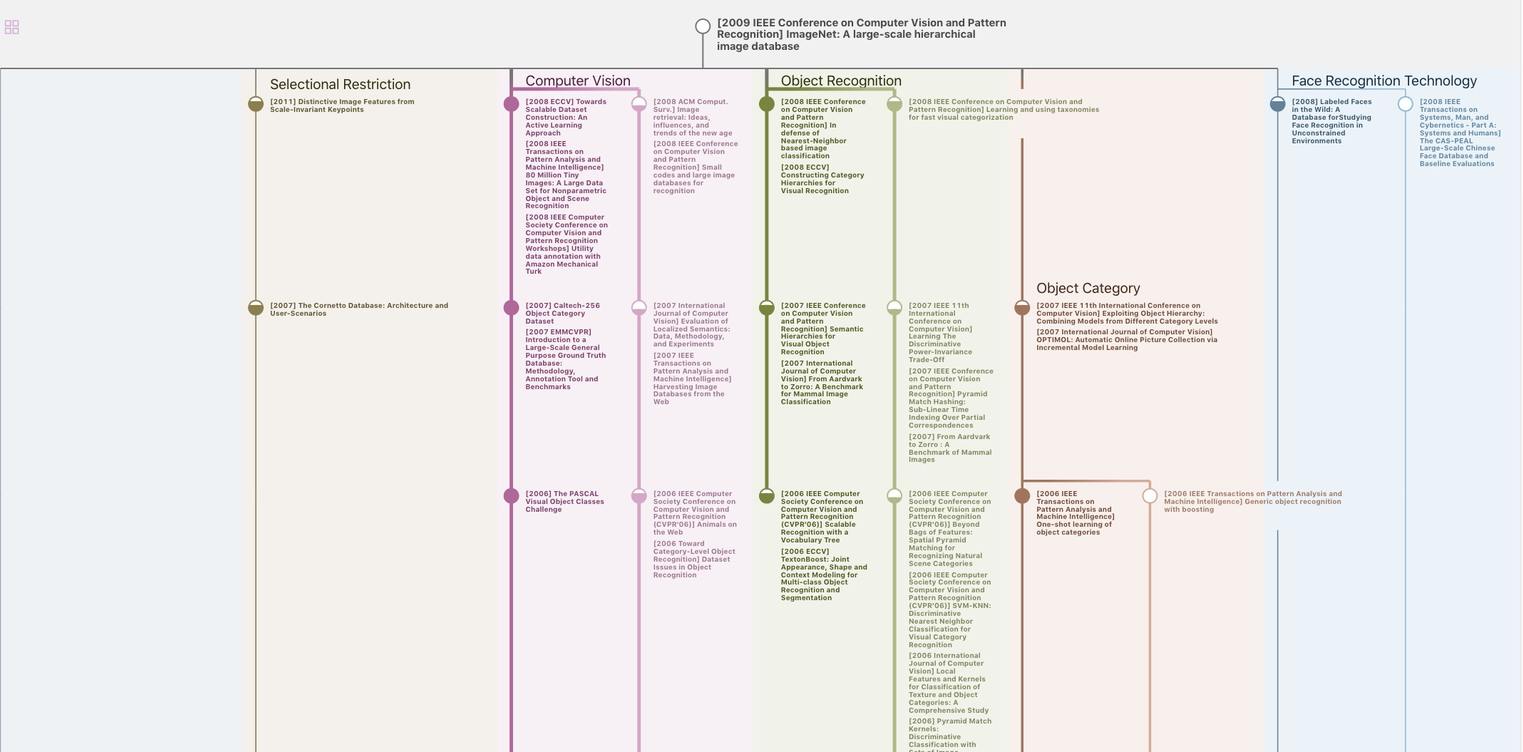
生成溯源树,研究论文发展脉络
Chat Paper
正在生成论文摘要