Creative memory semantization through adversarial dreaming
semanticscholar(2021)
摘要
Classical theories of memory consolidation emphasize the importance of episodic replay during non rapid-eye-movement (NREM) sleep as a key mechanism to extract semantic information from encoded memories. However, the experimentally reported creative nature of dreams suggest that memory semantization may go beyond merely replaying previous experiences. Here, we propose that rapid-eye-movement (REM) dreaming is essential for efficient memory semantization by randomly combining episodic memories to create new, virtual visual experiences. We support this hypothesis by implementing a cortical architecture with hierarchically organized feedforward and feedback pathways, loosely inspired by generative adversarial networks (GANs). Learning in our model is organized across three different global brain states mimicking wakefulness, NREM and REM sleep. In each of these phases, synaptic plasticity optimizes different, but complementary loss functions. We illustrate our model by training it on standard datasets of natural images and show that it develops structured latent representations in an unsupervised fashion which can be efficiently used by downstream areas, for example performing object recognition. Our results suggest that creative dreaming during REM forms semantic representations, while episodic replay during NREM improves their robustness against input perturbations such as visual occlusions. Our biologically-inspired framework provides a computational perspective on the functional relevance of sleep, memory replay and dreams. It thereby helps to interpret existing data, makes predictions for future experiments, and serves as a basis for detailed microcircuit models of the described mechanisms.
更多查看译文
关键词
memory
AI 理解论文
溯源树
样例
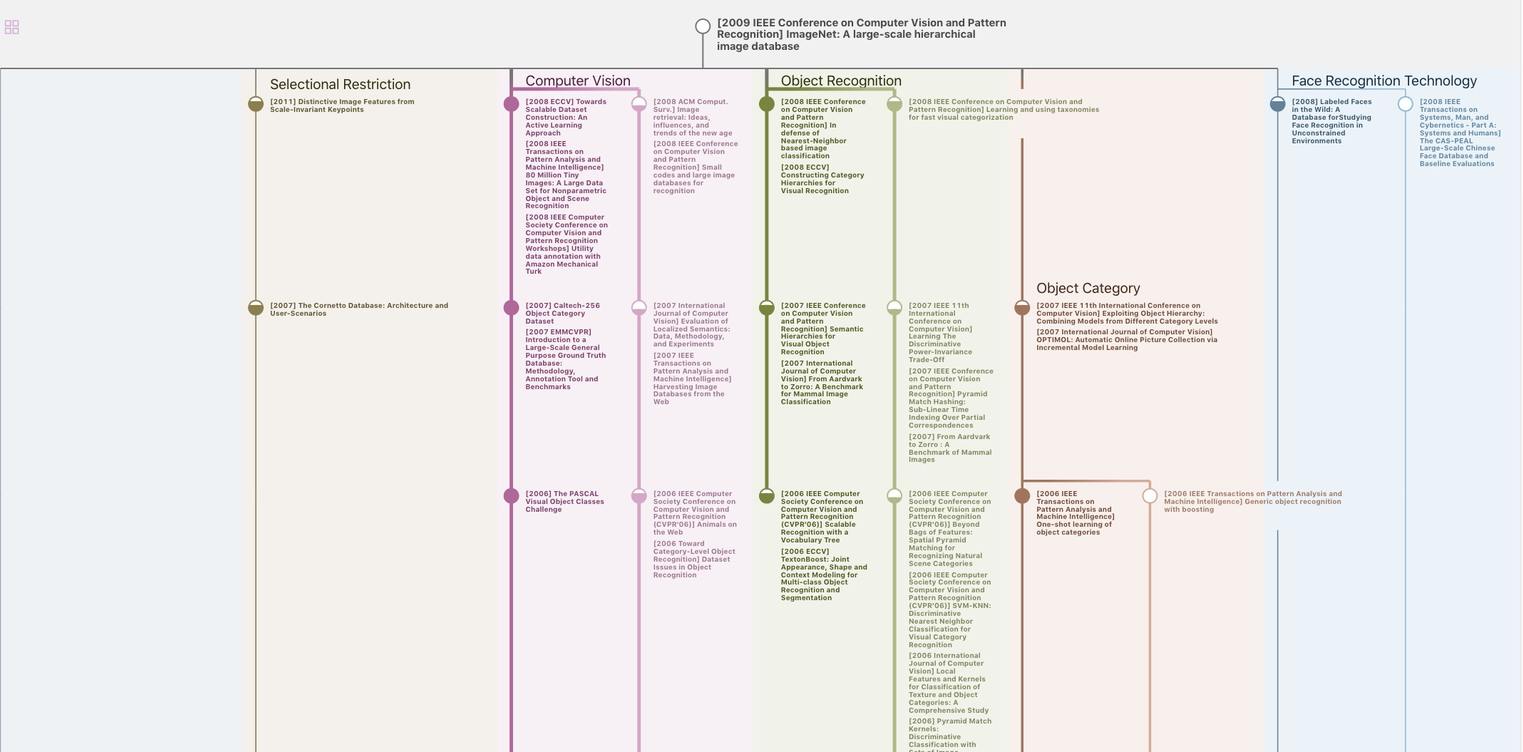
生成溯源树,研究论文发展脉络
Chat Paper
正在生成论文摘要