TTAN: Two-Stage Temporal Alignment Network for Few-shot Action Recognition
ArXiv(2021)
摘要
Few-shot action recognition aims to recognize novel action classes (query) using just a few samples (support). The majority of current approaches follow the metric learning paradigm, which learns to compare the similarity between videos. Recently, it has been observed that directly measuring this similarity is not ideal since different action instances may show distinctive temporal distribution, resulting in severe misalignment issues across query and support videos. In this paper, we arrest this problem from two distinct aspects – action duration misalignment and motion evolution misalignment. We address them sequentially through a Two-stage Temporal Alignment Network (TTAN). The first stage performs temporal transformation with the predicted affine warp parameters, while the second stage utilizes a cross-attention mechanism to coordinate the features of the support and query to a consistent evolution. Besides, we devise a novel multi-shot fusion strategy, which takes the misalignment among support samples into consideration. Ablation studies and visualizations demonstrate the role played by both stages in addressing the misalignment. Extensive experiments on benchmark datasets show the potential of the proposed method in achieving state-of-the-art performance for few-shot action recognition.
更多查看译文
关键词
action,recognition,ttan,two-stage,few-shot
AI 理解论文
溯源树
样例
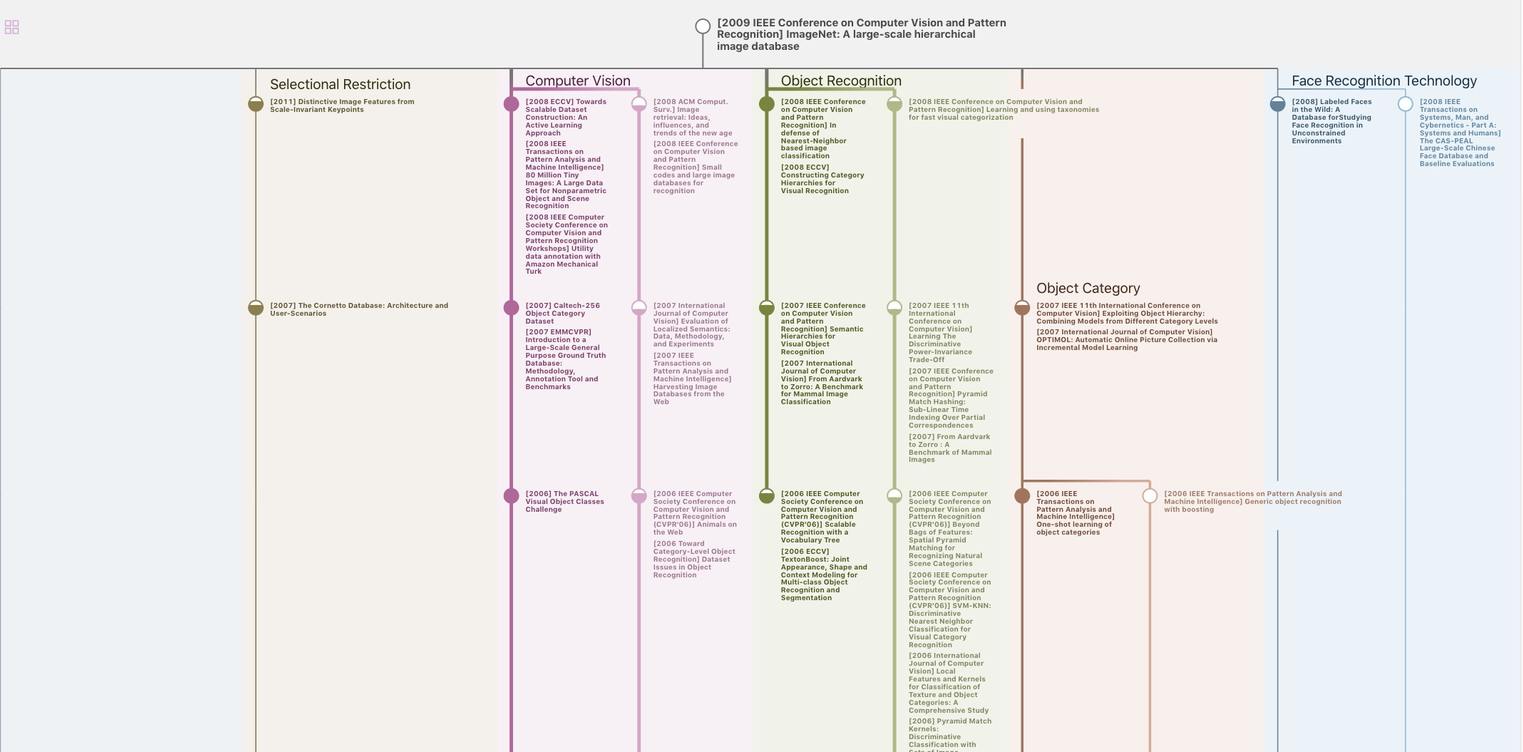
生成溯源树,研究论文发展脉络
Chat Paper
正在生成论文摘要