A Study of a Data Standardization and Cleaning Technique for a Facial Emotion Recognition System
semanticscholar(2020)
摘要
In this paper, we introduce a data standardization and cleaning technique with different facial emotion recognition (FER) datasets in order to improve the FER model system. We discovered some irrelevant facial images in FER 2013 dataset after we successfully converted the comma-separated values (CSV) file into actual images. For FER, we employ the mini-Xception model and in order to improve its performance, all irrelevant facial images must be discarded. After discarding these irrelevant images, we saw the gap between training and testing accuracy and error reducing. Moreover, we obtained a variety of FER datasets such as CohnKanade, Japanese Female Facial Expression (JAFFE), and EmotionNet. We cropped the images using multitask cascade neural network (MTCNN), and resized images using Pyimagesearch, a tool for resizing images, because each dataset that we obtained had images of different sizes and was not cropped. This technique for data standardization and cleaning enables us to use the Cohn-Kanade dataset for testing and the FER 2013 for training even though initially, they have images of different sizes. As a result, the validation accuracy is increased by 5%, and the validation loss decreases by 2%. We recommend data standardization and cleaning to obtain and use more raw data images in order to improve the performance of the FER model produced by mini-Xception Algorithm.
更多查看译文
AI 理解论文
溯源树
样例
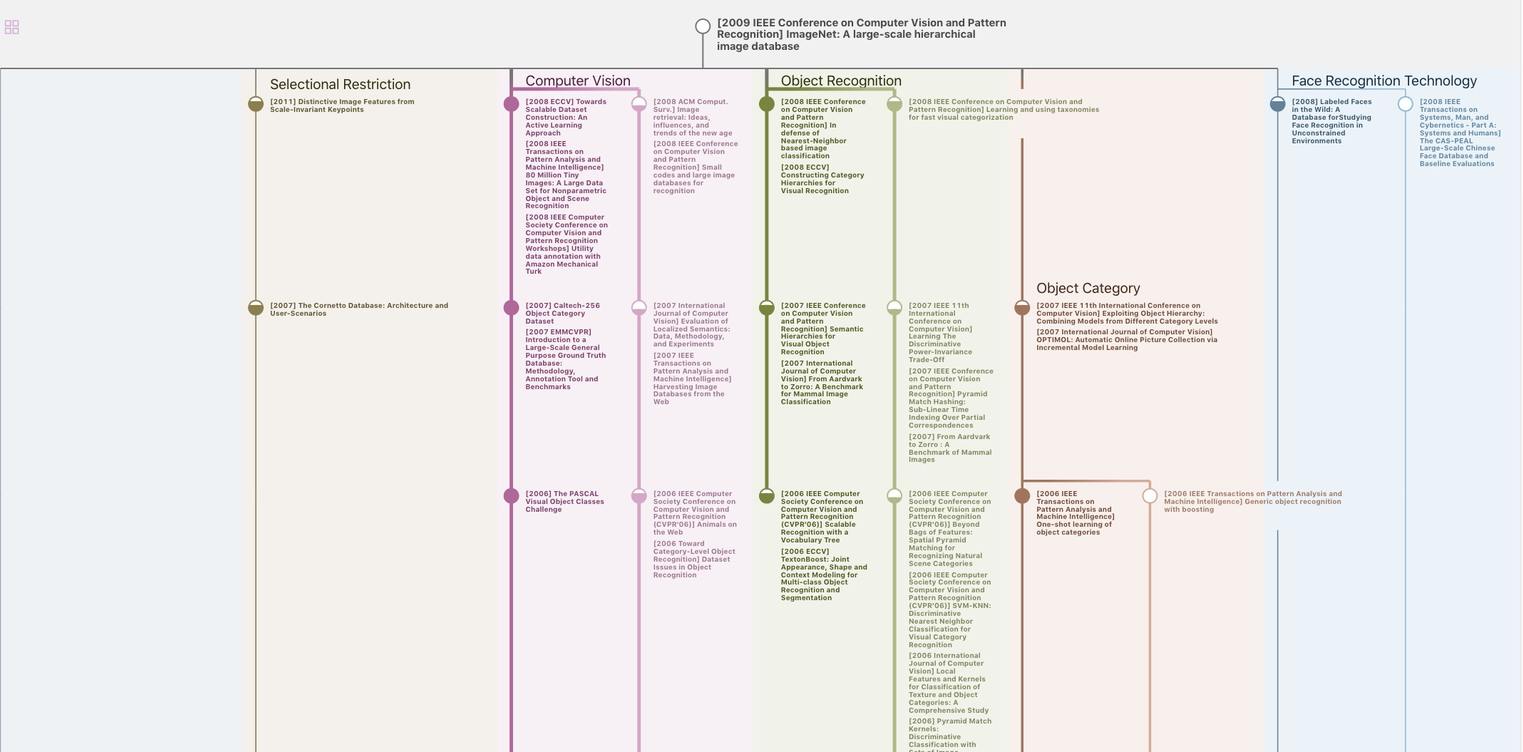
生成溯源树,研究论文发展脉络
Chat Paper
正在生成论文摘要