Joint dimension reduction and clustering analysis for single-cell RNA-seq and spatial transcriptomics data
biorxiv(2021)
摘要
Dimension reduction and (spatial) clustering are two key steps for the analysis of both single-cell RNA-sequencing (scRNA-seq) and spatial transcriptomics data collected from different platforms. Most existing methods perform dimension reduction and (spatial) clustering sequentially, treating them as two consecutive stages in tandem analysis. However, the low-dimensional embeddings estimated in the dimension reduction step may not necessarily be relevant to the class labels inferred in the clustering step and thus may impair the performance of the clustering and other downstream analysis. Here, we develop a computation method, DR-SC, to perform both dimension reduction and (spatial) clustering jointly in a unified framework. Joint analysis in DR-SC ensures accurate (spatial) clustering results and effective extraction of biologically informative low-dimensional features. Importantly, DR-SC is not only applicable for cell type clustering in scRNA-seq studies but also applicable for spatial clustering in spatial transcriptimics that characterizes the spatial organization of the tissue by segregating it into multiple tissue structures. For spatial transcriptoimcs analysis, DR-SC relies on an underlying latent hidden Markov random field model to encourage the spatial smoothness of the detected spatial cluster boundaries. We also develop an efficient expectation-maximization algorithm based on an iterative conditional mode. DR-SC is not only scalable to large sample sizes, but is also capable of optimizing the spatial smoothness parameter in a data-driven manner. Comprehensive simulations show that DR-SC outperforms existing clustering methods such as Seurat and spatial clustering methods such as BayesSpace and SpaGCN and extracts more biologically relevant features compared to the conventional dimension reduction methods such as PCA and scVI. Using 16 benchmark scRNA-seq datasets, we demonstrate that the low-dimensional embeddings and class labels estimated from DR-SC lead to improved trajectory inference. In addition, analyzing three published scRNA-seq and spatial transcriptomics data in three platforms, we show DR-SC can improve both the spatial and non-spatial clustering performance, resolving a low-dimensional representation with improved visualization, and facilitate the downstream analysis such as trajectory inference.
### Competing Interest Statement
The authors have declared no competing interest.
更多查看译文
AI 理解论文
溯源树
样例
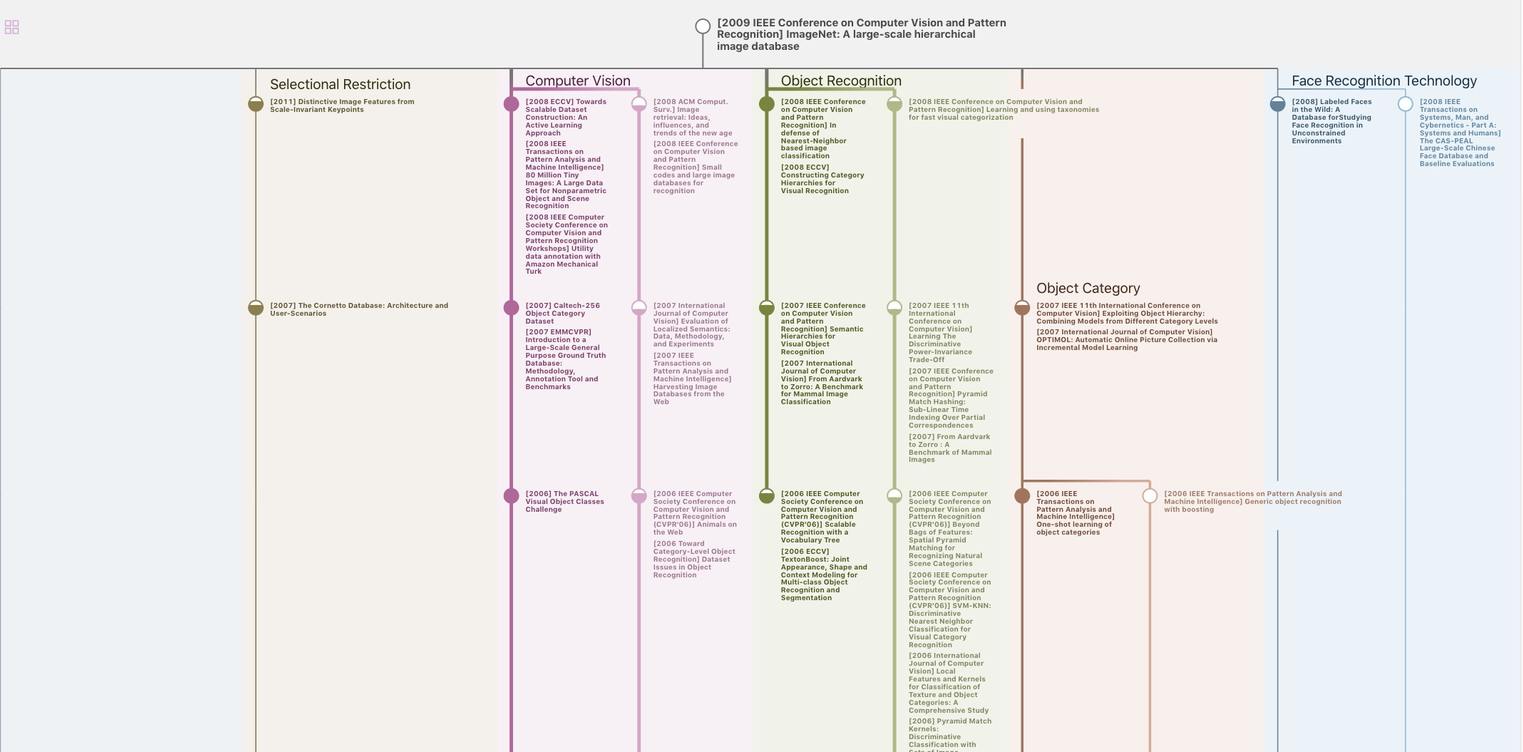
生成溯源树,研究论文发展脉络
Chat Paper
正在生成论文摘要