A Comprehensive Review of Machine Learning in Multi-objective Optimization
2021 IEEE 4th International Conference on Big Data and Artificial Intelligence (BDAI)(2021)
Abstract
In the real world, it is challenging to calculate a trade-off alternative with traditional classical methods for complex non-linear systems, which always involve multiple conflicting objectives. Such complicated systems urgently desire advanced methods to conquer the multi-objective optimization problems (MOPs). As a promising AI method, the development and application of Machine Learning (ML) attract increasingly more attention from researchers. The natures of ML methods, such as parallel computation possibility, no need for any priori assumptions, etc., ensure the effectiveness and efficiency for solving MOPs. However, as we know, there is no literature related to the comprehensive review of ML in multi-objective optimization domain until now. This literature review aims to provide researchers a global view of mainstream ML methods for MOO in a general domain and a reference for applying ML methods to solve a specific type of MOPs. In this paper, the general ML mainstream methods are summarized, based on which the literature relating to ML on MOPs are retrieved in comprehensive domains. The relevant literature is categorized according to the emphasis of object types, purposes and methods, and the categorization results are finally analyzed and discussed.
MoreTranslated text
Key words
multi-objective optimization,machine learning,reinforcement learning,neural network
AI Read Science
Must-Reading Tree
Example
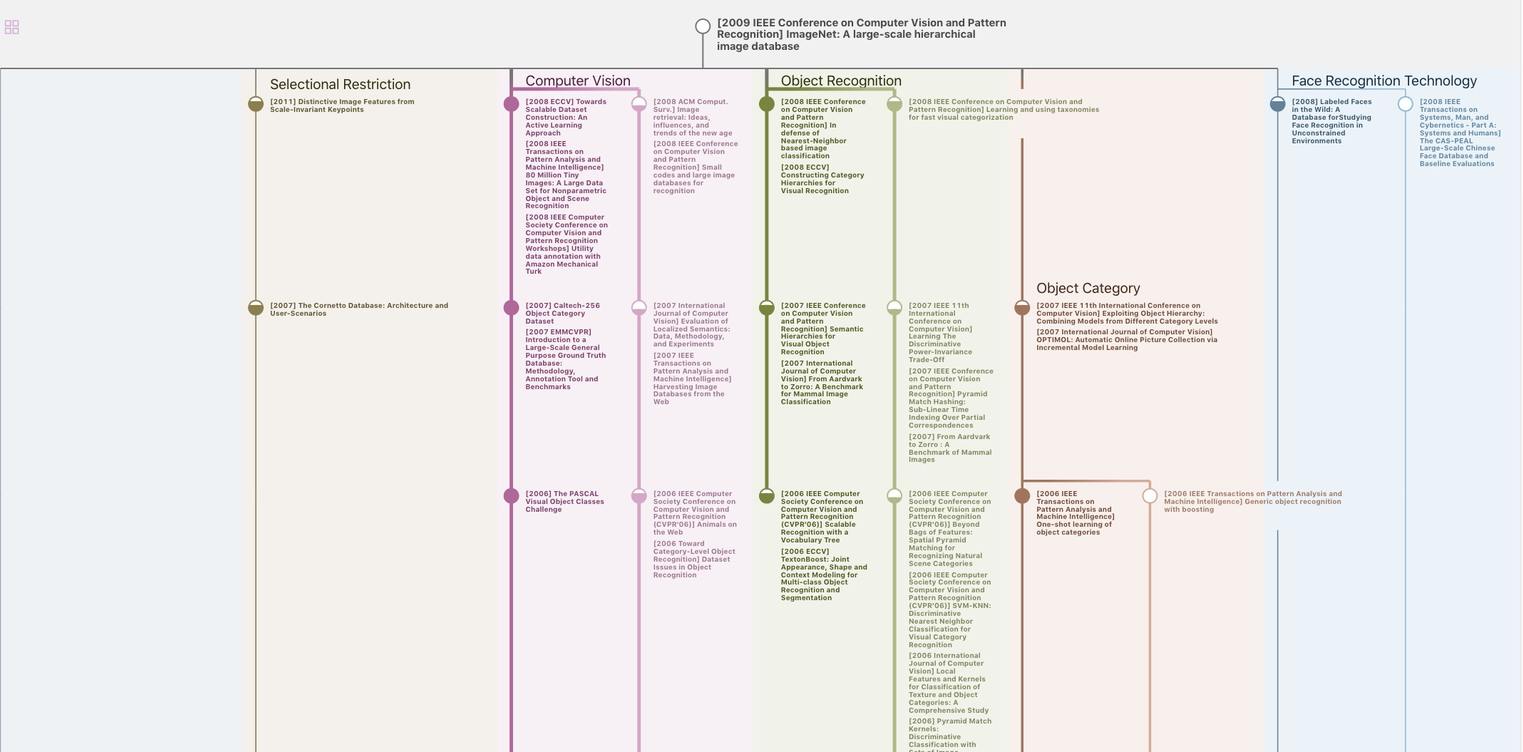
Generate MRT to find the research sequence of this paper
Chat Paper
Summary is being generated by the instructions you defined