Self-supervised Learning for Pore Detection in CT-Scans of Cast Aluminum Parts
semanticscholar(2019)
摘要
Automatically detecting pores in computed tomography (CT) scans of cast aluminum parts is a difficult task. This is especially the case when the data quality suffers from image artifacts. These can arise due to constraints from the imaging process and the short scan times that are common in industry. In previous work we have shown that using the synthetic ground truth generated from a realistically simulated CT data set is sufficient to train modern machine learning algorithms to detect pores on real data sets—even when heavy image artifacts like noise, beam hardening, or ring artifacts are present. Fully convolutional neural networks can yield promising results when moving from the simulated data set to real world examples. So, instead of first removing artifacts from the image and then applying defect detection algorithms, we propose to tackle the defect detection task directly without altering the original data. In this paper we evaluate three models: (1) a fast slice-wise approach which does the prediction without the full 3D context, (2) an encoder-decoder-pair with an additional refinement step, which acquires context by reducing the spatial resolution, and (3) a plain convolutional neural network using dilated convolutions for context aggregation. Further, we provide promising qualitative results on difficult real world cases, among others CT-scans from in-line scenarios and additively manufactured parts.
更多查看译文
AI 理解论文
溯源树
样例
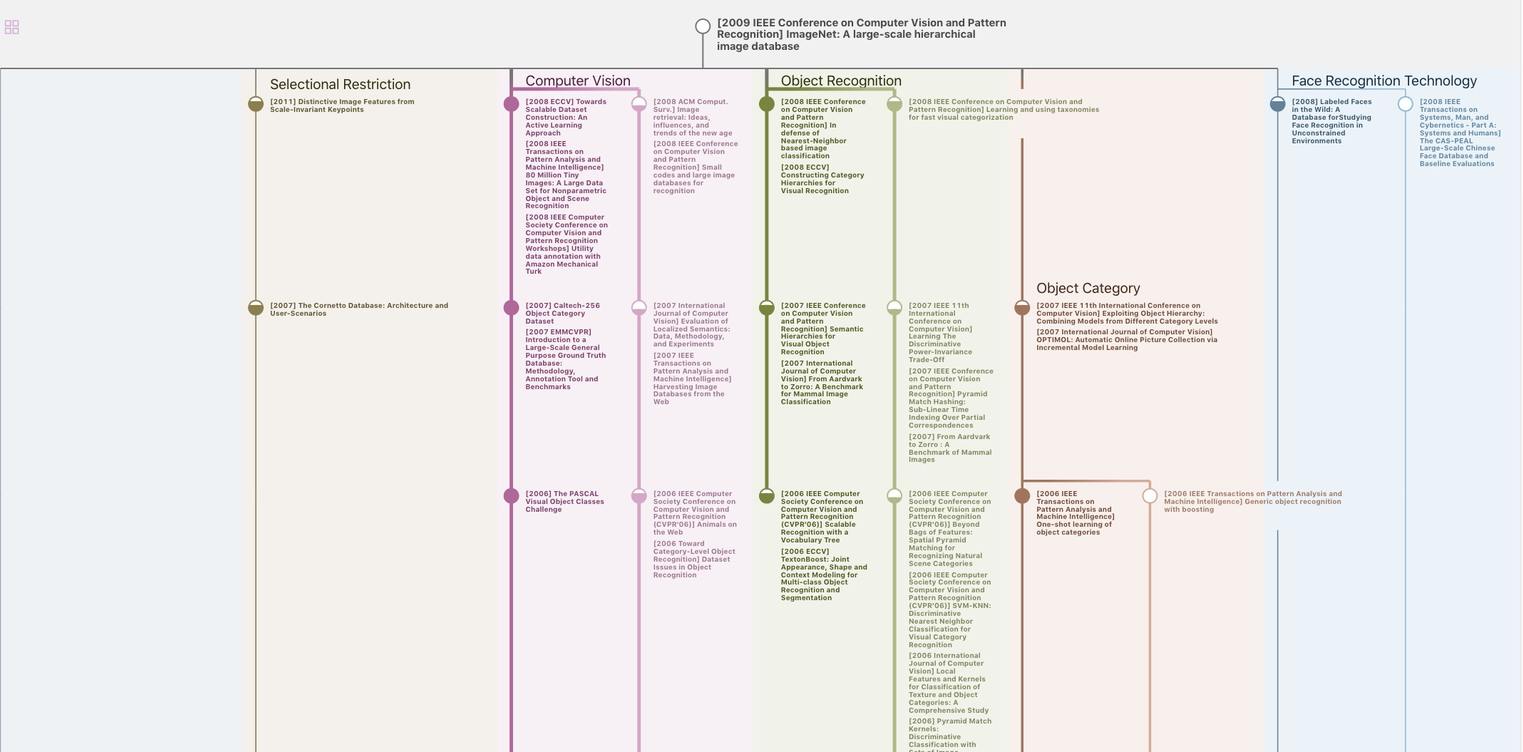
生成溯源树,研究论文发展脉络
Chat Paper
正在生成论文摘要