Transfer Learning vs. Batch Effects: what can we expect from neural networks in computational biology?
semanticscholar(2019)
摘要
The diverse applications of deep learning in computational biology include single-cell microscopy image analysis and prediction of transcription factor binding from DNA sequence. Although it is clear that CNNs and their derivatives will revolutionize these fields, it is not yet clear to what extent deep models will be transferred, reused or retrained for each application. For single cell identification/segmentation in microscope images, one study found remarkable generalization capacity of a mask-RCNN: with no parameter tuning, performance across microscopy datasets is competitive with conventional methods that have been highly tuned for each dataset. This type of generalization implies that a single model can be deployed over the web for all users. On the other hand, for protein subcellular localization classification in images, there is evidence for sensitivity to ‘batch’ or ‘out-of-sample’ effects, such that performance degrades on test sets taken at different times and on different instruments. We discuss similar issues in deep learning methods applied to transcription factor binding. We conclude that the issue of when models can generalize and when they must be retrained is largely unexplored, but will be critical in shaping how deep learning is applied to computational biology.
更多查看译文
AI 理解论文
溯源树
样例
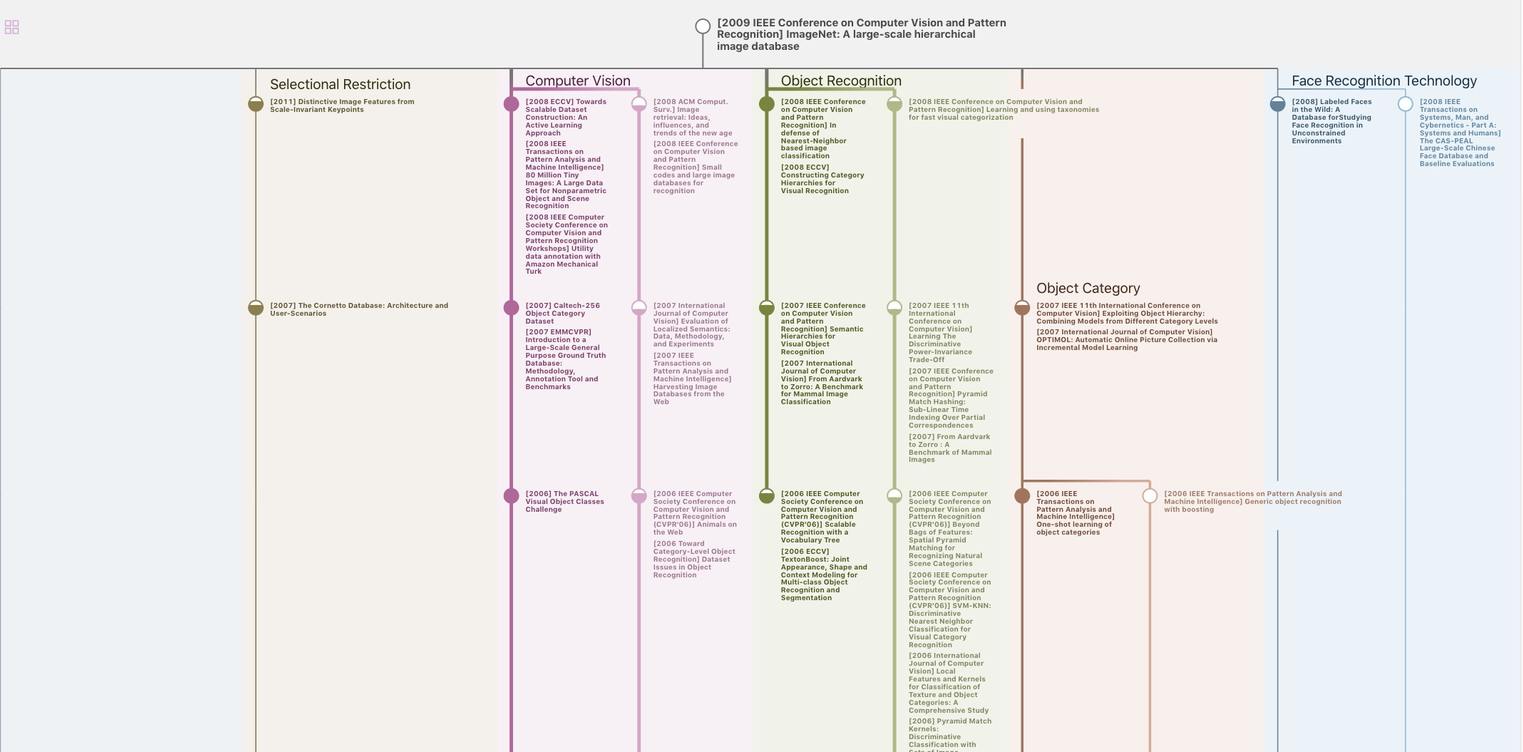
生成溯源树,研究论文发展脉络
Chat Paper
正在生成论文摘要