Development and Validation of a Multivariable Risk Prediction Model for Sudden Cardiac Death after Myocardial Infarction (PROFID Risk Model): Study Rationale, Design and Protocol
medRxiv(2021)
摘要
Introduction Sudden cardiac death (SCD) is the leading cause of death in patients with myocardial infarction (MI) and can be prevented by the implantable cardioverter defibrillator (ICD). Currently, risk stratification for SCD and decision on ICD implantation are based solely on impaired left ventricular ejection fraction (LVEF). However, this strategy leads to over- and under-treatment of patients because LVEF alone is insufficient for accurate assessment of prognosis. Thus, there is a need for better risk stratification. This is the study protocol for developing and validating a prediction model for risk of SCD in patients with prior MI. Methods and Analysis The EU funded PROFID project will analyse 23 datasets from Europe, Israel and the US (~225,000 observations). The datasets include patients with prior MI or ischemic cardiomyopathy with reduced LVEF<50%, with and without a primary prevention ICD. Our primary outcome is SCD in patients without an ICD, or appropriate ICD therapy in patients carrying an ICD as a SCD surrogate. For analysis, we will stack 18 of the datasets into a single database (datastack), with the remaining analysed remotely for data governance reasons (remote data). We will apply 5 analytical approaches to develop the risk prediction model in the datastack and the remote datasets, all under a competing risk framework: 1) Weibull model, 2) flexible parametric survival model, 3) random forest, 4) likelihood boosting machine, and 5) neural network. These dataset-specific models will be combined into a single model (one per analysis method) using model aggregation methods, which will be externally validated using systematic leave-one-dataset-out cross-validation. Predictive performance will be pooled using random effects meta-analysis to select the model with best performance. Ethics and dissemination Local ethical approval was obtained. The final model will be disseminated through scientific publications and a web-calculator. Statistical code will be published through open-source repositories.
更多查看译文
AI 理解论文
溯源树
样例
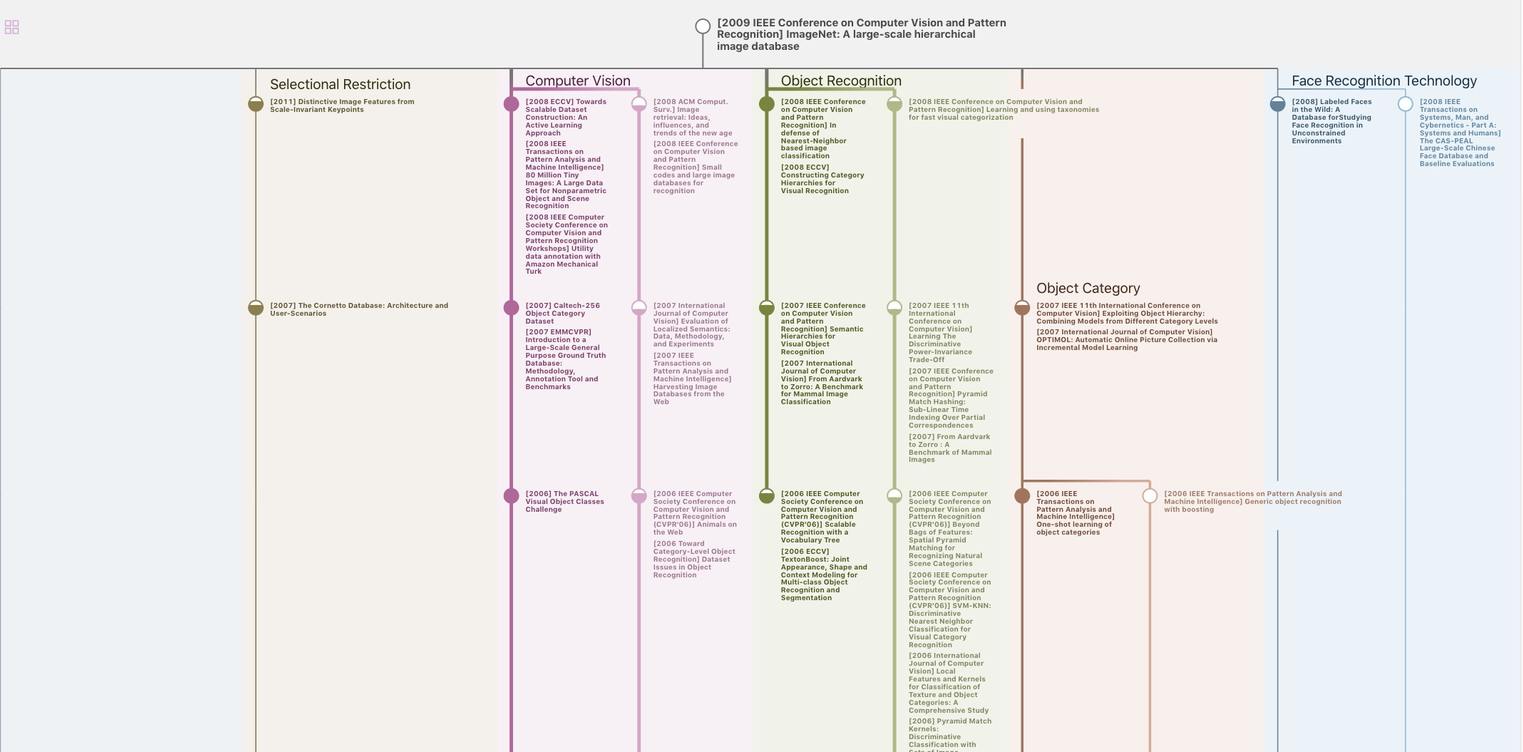
生成溯源树,研究论文发展脉络
Chat Paper
正在生成论文摘要