Scenario Reduction Network Based on Wasserstein Distance With Regularization
IEEE TRANSACTIONS ON POWER SYSTEMS(2024)
摘要
Power systems with high penetration of renewable energy contain various uncertainties. Scenario-based optimization problems need a large number of discrete scenarios to obtain a reliable approximation for the probabilistic model. It is important to choose typical scenarios and ease the computational burden. This paper presents a scenario reduction network model based on Wasserstein distance. Entropy regularization is used to transform the scenario reduction problem into an unconstrained problem. Through an explicit neural network structure design, the output of the scenario reduction network corresponds to the Sinkhorn distance function. The scenario reduction network can generate the typical scenario set through unsupervised learning. An efficient algorithm is proposed for continuous/discrete scenario reduction. The superiority of the scenario reduction network-model is verified through case studies. The numerical results highlight the high accuracy and computational efficiency of the proposed model over state-of-the-art models, and it makes an ideal candidate for large-scale scenario reduction problems.
更多查看译文
关键词
Scenario reduction,Wasserstein distance,entropy regularization,Sinkhorn distance,neural network
AI 理解论文
溯源树
样例
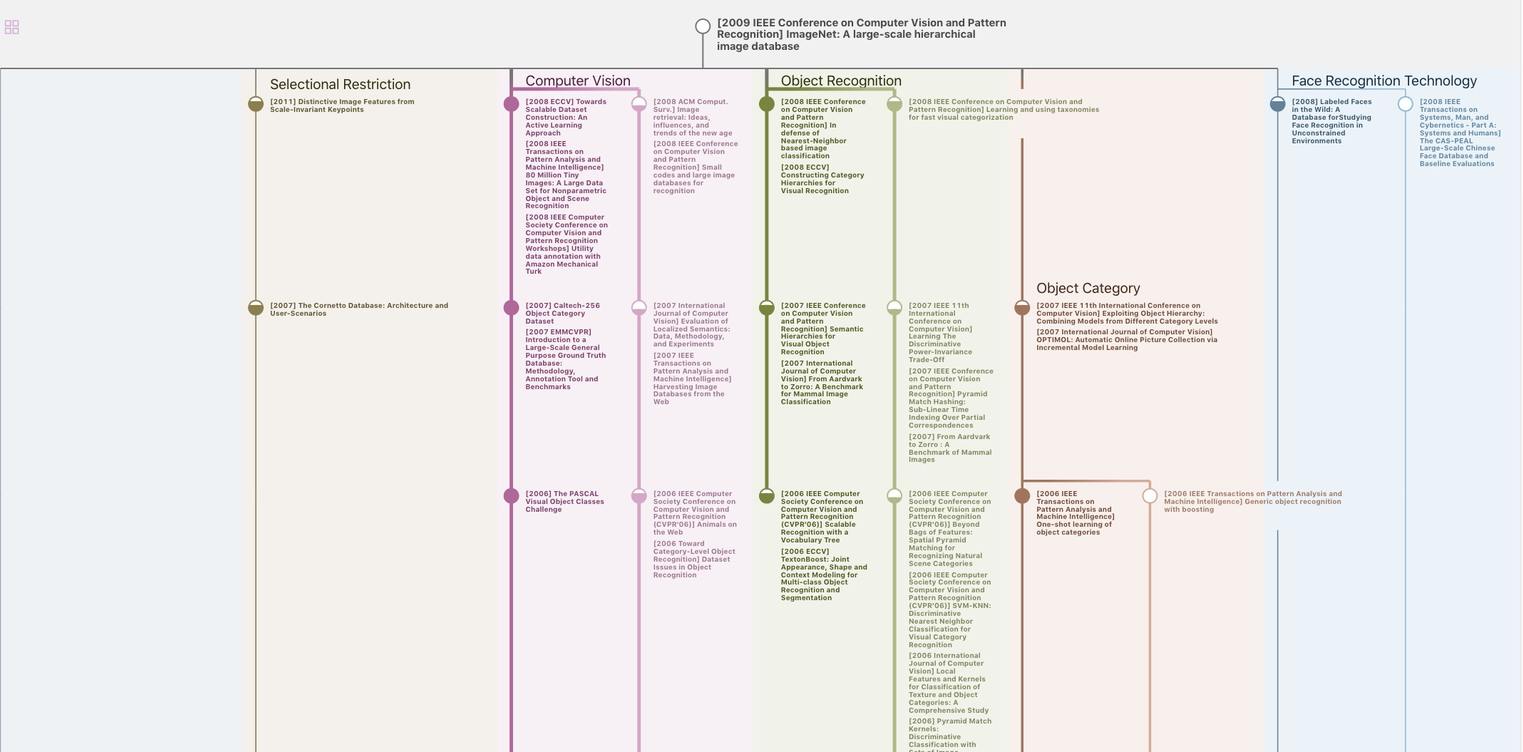
生成溯源树,研究论文发展脉络
Chat Paper
正在生成论文摘要