Survey of Bias Mitigation in Federated Learning
HAL (Le Centre pour la Communication Scientifique Directe)(2021)
摘要
Federated Learning (FL) interestingly allows a set of participants to collectively resolve a machine learning problem in a decentralized and privacy preserving manner. However, data distribution and heterogeneity, that are inherent to FL, may induce and exacerbate the problem of bias, with its prejudicial consequences such as racial or sexist segregation, illegal actions, or reduced revenues. In this paper, we describe the problem of bias in Federated Learning, and provide a comparative review of existing approaches of FL bias mitigation, before discussing open challenges and interesting research directions.
更多查看译文
关键词
bias mitigation,learning
AI 理解论文
溯源树
样例
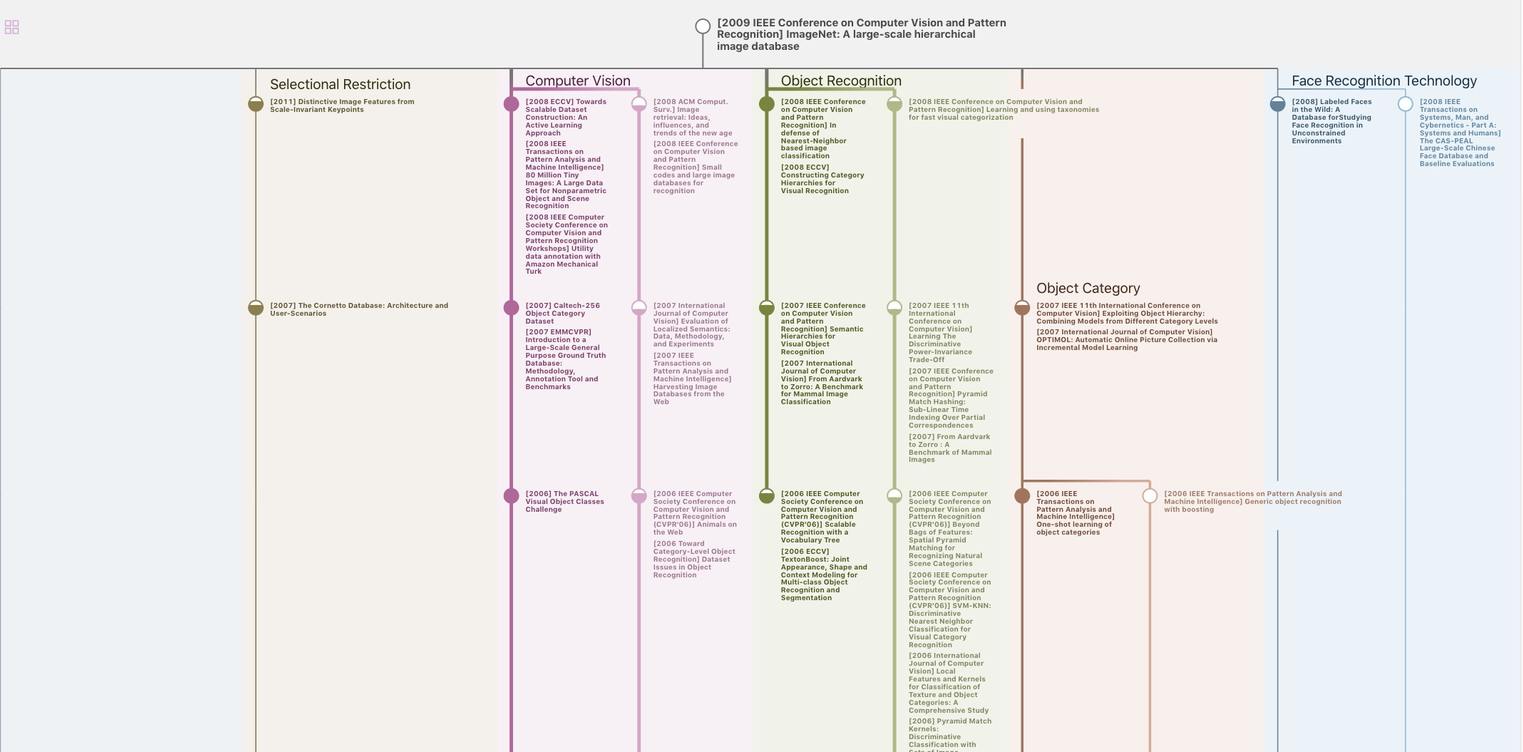
生成溯源树,研究论文发展脉络
Chat Paper
正在生成论文摘要