End-to-End Method With Transformer for 3-D Detection of Oil Tank From Single SAR Image
IEEE TRANSACTIONS ON GEOSCIENCE AND REMOTE SENSING(2022)
摘要
In recent years, deep learning has been successfully applied in the field of synthetic aperture radar (SAR) image object detection. However, unlike ships and tanks targets, the oil tank targets in SAR image are usually dense and compact with more overlaps and discrete scattering centers, which greatly increases the difficulty of extracting location and structural parameters. Most of the existing methods transfer the methods suitable for natural image to the SAR image field, without considering the unique characteristics of SAR image. Therefore, in this article, we propose an improved model based on the end-to-end transformer network, which is the first model introducing transformer network to 3-D detection of oil tank targets from single SAR image. We input the incidence angle into the transformer model as a priori token. Then, we propose a feature description operator (FDO) based on the scattering centers that are used as an aid to improve the precision of predictions. In addition, we also propose a cylinder IOU as a more suitable evaluation metric for 3-D detection of oil tank. Finally, we evaluate our model on an SAR image dataset that contains SAR images from RADARSAT-2, TerraSAR-X, and GF-3 with different incidence angles. Our experiments demonstrate that our proposed model achieves the AP of 77.6% compared with 60.8% of baseline, which proves the effectiveness of the introduction of observation conditions, cylinder IOU loss (CI Loss), and the FDO based on the scattering centers in our model and is appealing for 3-D detection of oil tank.
更多查看译文
关键词
3-D detection of oil tank,cylinder intersection over union (IOU) (cylinder IOU),feature description operator (FDO),priori token,single SAR image,transformer
AI 理解论文
溯源树
样例
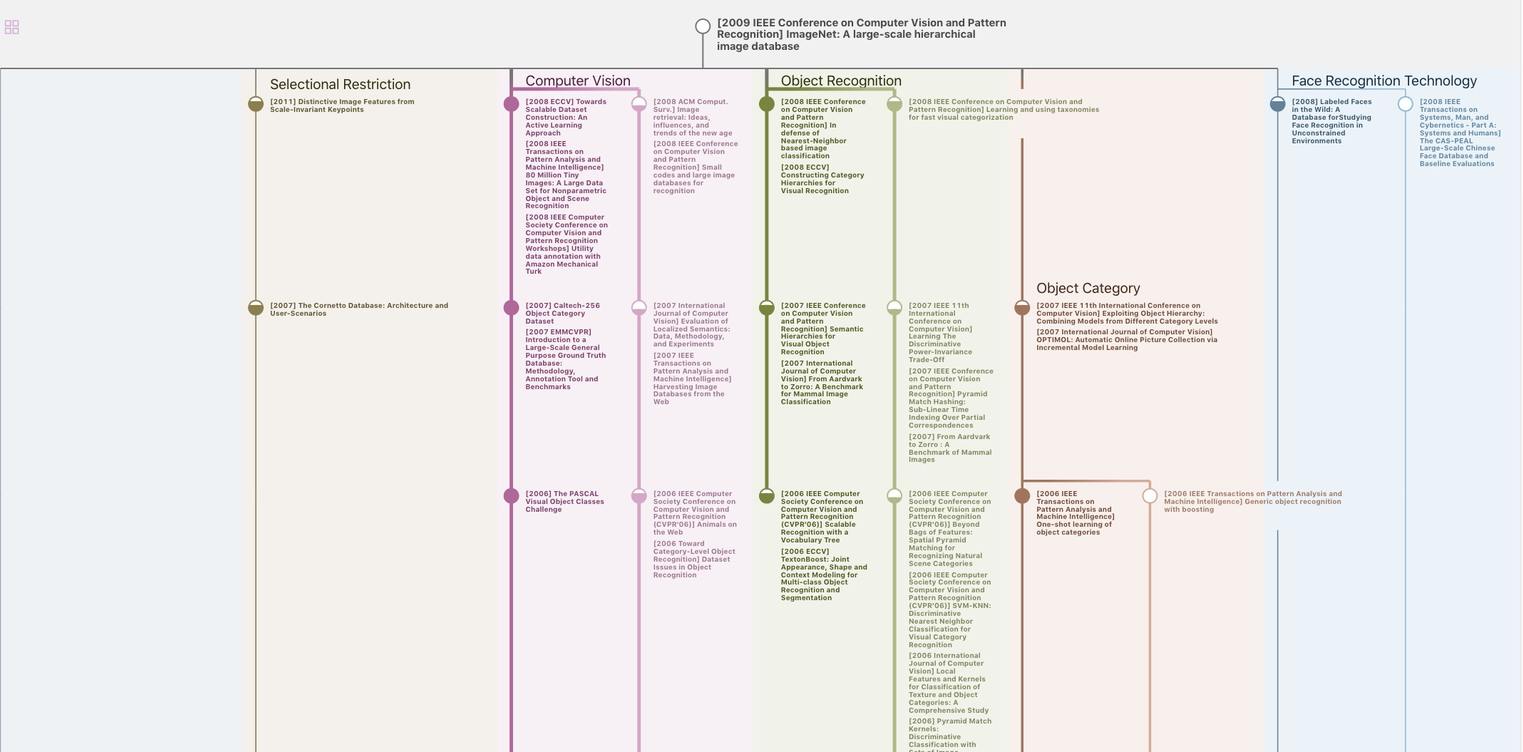
生成溯源树,研究论文发展脉络
Chat Paper
正在生成论文摘要