Short Text Clustering in Continuous Time Using Stacked Dirichlet-Hawkes Process with Inverse Cluster Frequency Prior
COMAD/CODS(2023)
摘要
Traditional models for short text clustering ignore the time information associated with the text documents. However, existing works have shown that temporal characteristics of streaming documents are significant features for clustering. In this paper we propose a stacked Dirichlet-Hawkes process with inverse cluster frequency prior as a simple but effective solution for the task of short text clustering using temporal features in continuous time. Based on the classical formulation of the Dirichlet-Hawkes process, our model provides an elegant, theoretically grounded and interpretable solution while performing at par with recent state of the art models in short text clustering. ACM Reference Format: Avirup Saha and Balaji Ganesan. 2021. Short Text Clustering in Continuous Time Using Stacked Dirichlet-Hawkes Process with Inverse Cluster Frequency Prior. In MileTS ’21: 7th KDD Workshop on Mining and Learning from Time Series, August 14th, 2021, Singapore. ACM, New York, NY, USA, 5 pages. https://doi.org/10.1145/1122445.1122456
更多查看译文
AI 理解论文
溯源树
样例
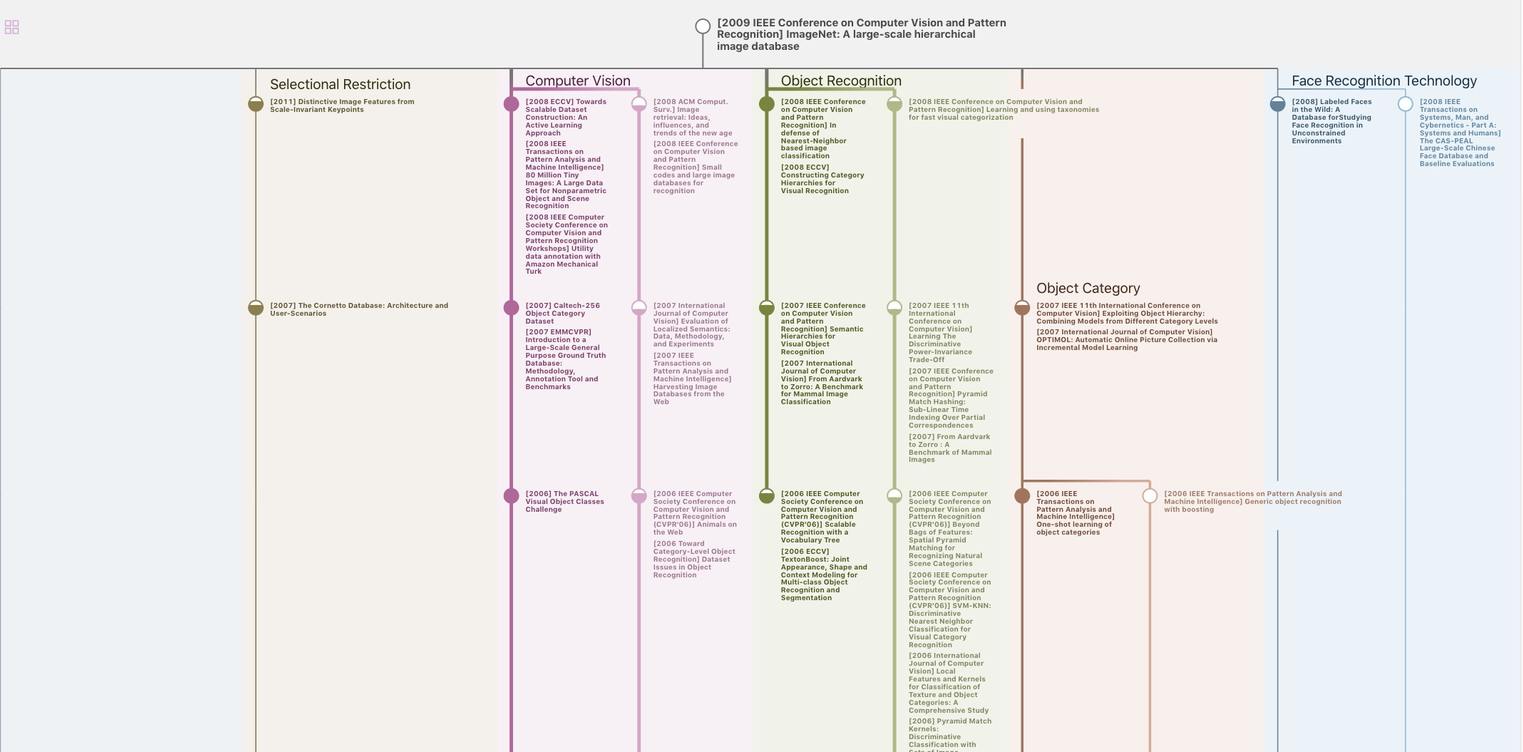
生成溯源树,研究论文发展脉络
Chat Paper
正在生成论文摘要