Developing an Interpretable Schizophrenia Deep Learning Classifier on fMRI and sMRI using a Patient-Centered DeepSHAP
semanticscholar(2020)
摘要
This paper aims to explore the applications of interpretable methods to the task of schizophrenia classification. We use the Mind Research Network’s data which consists of functional network connectivity values (FNC) and source-based morphometry (SBM) loadings. These features are derived from fMRI and sMRI, respectively. We design a deep neural network for the classification task and achieve high AUC for the literature. Then, we explore various iterations of DeepSHAP, a form of SHapley Additive exPlanations or estimations of Shapley values, as our interpretable method. We find that Standard DeepSHAP is a successful tool for schizophrenia interpretability. However, we note that a Label-Consolidated DeepSHAP more accurately portrays collective feature importance for FNC values. Lastly, we conduct initial needfinding for a Patient-Centered DeepSHAP concept; from a human-centered perspective, we explore a patient experience with our DeepSHAP results.
更多查看译文
AI 理解论文
溯源树
样例
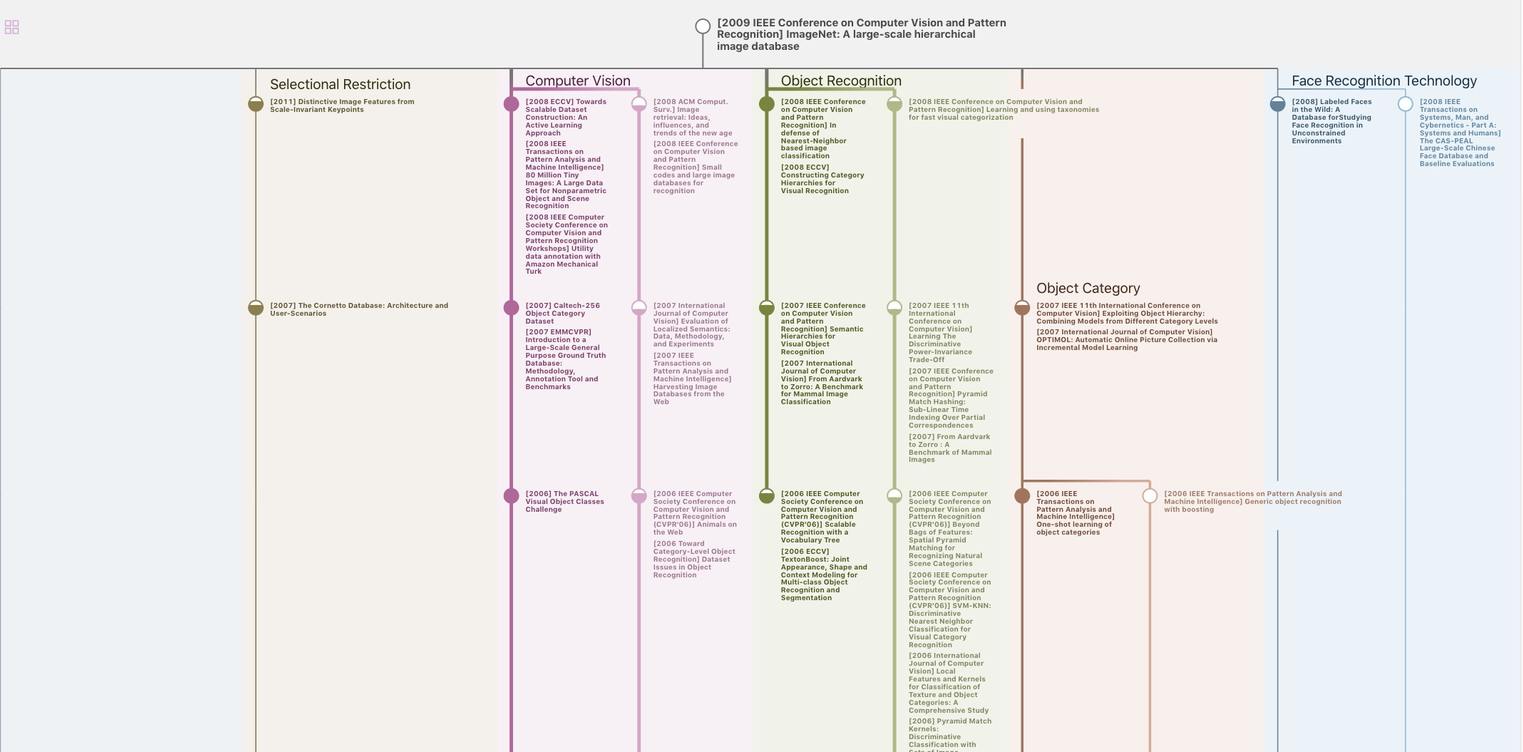
生成溯源树,研究论文发展脉络
Chat Paper
正在生成论文摘要