Semi-Supervised Unmixing of Hyperspectral Data via Spectral-Spatial Factorization
IEEE SENSORS JOURNAL(2021)
Abstract
Due to the limited spatial resolution of hyperspectral sensors, each pixel in hyperspectral image often consists of several components, called endmembers. Hyperspectral unmixing aims at extracting these endmembers and corresponding fractional abundances from the hyperspectral image (HSI) data. With the availability of spectral libraries, semi-supervised unmixing which estimates the abundance from given endmember matrix, have become more and more popular. General semi-supervised methods take advantage of the sparsity constraint on the abundance matrix and consider the pixels as independent trials. However, the spatial information for example the correlation between pixels often cannot be taken into consideration. In this paper, we derive a semi-supervised hyperspectral image unmixing algorithm which handles both spectral and spatial prior efficiently using matrix factorization. The abundance matrix is recast as a multiplication of two variables, in which the spectral and spatial priors are captured respectively. Numerical tests in both simulated and real datasets show that compared to state-of-the-art unmixing algorithms, the proposed spectral-spatial factorization method has lower computation cost, better unmixing results, and is more robust to regularization parameter selection.
MoreTranslated text
Key words
Hyperspectral imaging,Sparse matrices,Libraries,Sensors,Minimization,Signal processing algorithms,Art,Hyperspectral unmixing,sparse unmixing,spectral-spatial factorization
AI Read Science
Must-Reading Tree
Example
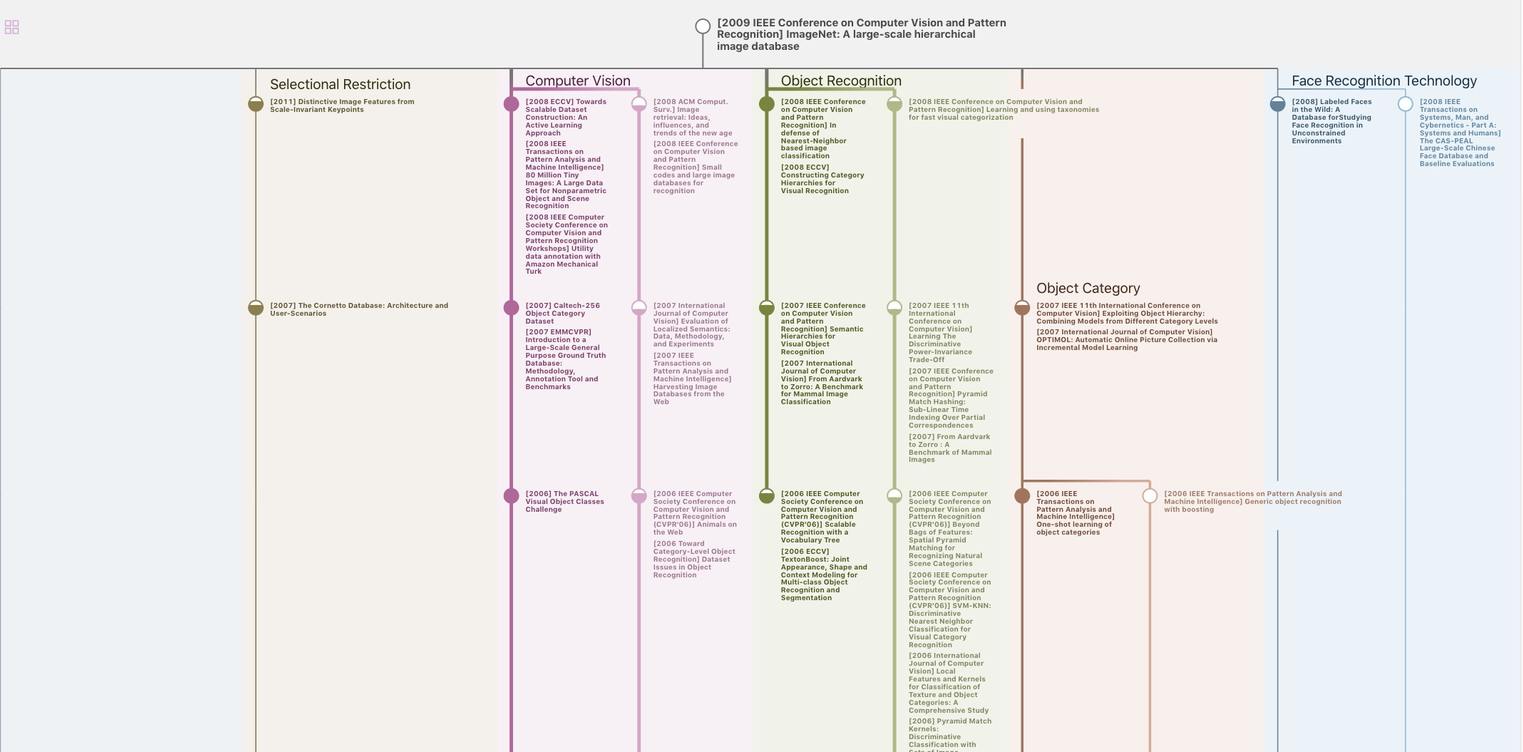
Generate MRT to find the research sequence of this paper
Chat Paper
Summary is being generated by the instructions you defined