CANet: An Unsupervised Deep Convolutional Neural Network for Efficient Cluster-Analysis-Based Multibaseline InSAR Phase Unwrapping
IEEE TRANSACTIONS ON GEOSCIENCE AND REMOTE SENSING(2022)
摘要
Multibaseline (MB) phase unwrapping (PU) is a vital processing procedure for MB synthetic aperture radar interferometry (InSAR) signal processing and can improve the traditional InSAR by changing the ill-posed problem to the well-posed problem. The existing research has shown that the MB PU problem can be successfully converted into an unsupervised cluster analysis problem. Using the high feature descriptiveness of the deep learning technique, an unsupervised deep convolutional neural network, referred to as CANet, is proposed to cluster all the pixels into different groups according to the input's recognizable pattern of the ambiguity number of the MB interferometric phase. Subsequently, we extend our previous two-stage programming-based MB processing approach (TSPA) to processing MB PU on a sparse irregular network, which is established from the clustering result of CANet. Both theoretical analysis and experimental results show that the proposed method is an effective MB PU method, and its execution time is drastically lower than those of many classical MB PU methods.
更多查看译文
关键词
Histograms,Cathode ray tubes,Feature extraction,Phase noise,Synthetic aperture radar,Pattern recognition,Transforms,Cluster analysis (CA),multibaseline (MB),phase unwrapping (PU),synthetic aperture radar interferometry (InSAR),unsupervised deep convolutional neural network (DCNN)
AI 理解论文
溯源树
样例
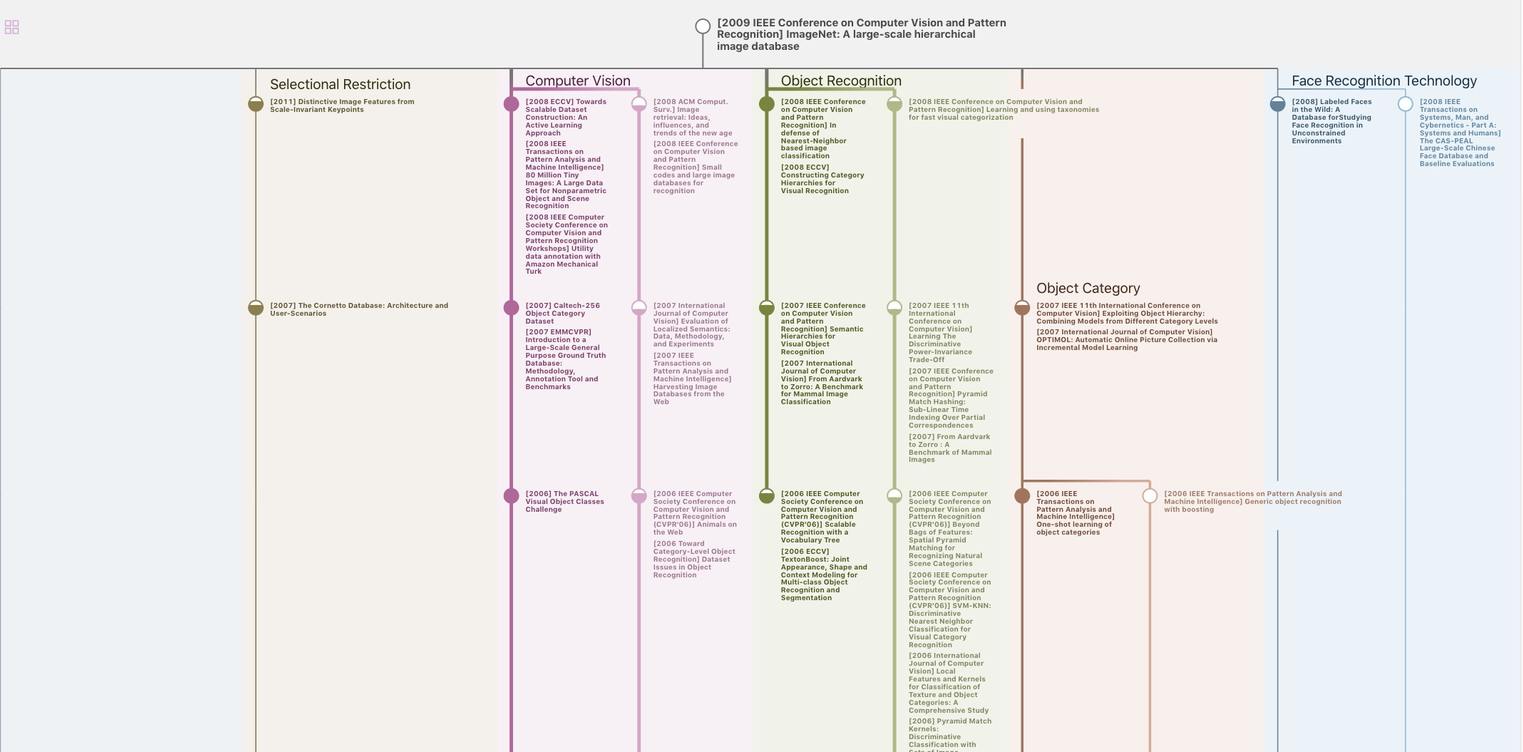
生成溯源树,研究论文发展脉络
Chat Paper
正在生成论文摘要