Computational Inverse Design of Surface-based Inflatables Supplementary Material
semanticscholar(2021)
摘要
We initialize our flatting by computing a least-squares conformal map Lévy et al. [2002] and then run 1000 iterations of the local-global fitting algorithm minimizing Efit so that the singular values σ0 and σ1 are well separated: this is necessary to avoid singularities in our parametrization gradient and Hessian formulas. This process takes only a few seconds on all examples shown, and the resulting singular value histogram gives a good early indication of whether the target surface can feasibly be produced with a single inflatable patch. We then run several rounds of the parametrization algorithm, gradually reducing the regularization weights. A typical weight sequence starts with (wφ, wσ, wκ) = (100, 10, 500) and runs 4 additional rounds, dividing wφ and wσ by 10 and wκ by 2 after each round. Subsequent runs after the first tend to converge relatively quickly, allowing semi-interactive weight tuning. Since our parametrization energy definition is not mesh dependent and we normalize the input surface dimensions before flattening, similar weights can be used across models. Our Newton solver’s default strategy for coping with indefinite Hessians (like those of our highly nonconvex parametrization energy) is the common approach of adding an increasingly large multiple of the identity matrix until a positive definite matrix is achieved. This trial-and-error process is slow and the modification ends up severely damping the solver’s progress. We dramatically accelerate the flattening algorithm by projecting each triangle’s contribution to the singular-value-fitting term’s Hessian to be positive semi-definite using a 6×6 Eigendecomposition. We use a simple heuristic for enabling and disabling this projection: after 5 consecutive Newton iterations with indefinite Hessians we enable the projection for 15 iterations. We note that while the smoothing and bending regularization terms have a different structure and cannot be handled by this per-triangle projection, they primarily act to increase the Hessian eigenvalues, not decrease them, meaning our simple projection approach is still effective in practice.
更多查看译文
AI 理解论文
溯源树
样例
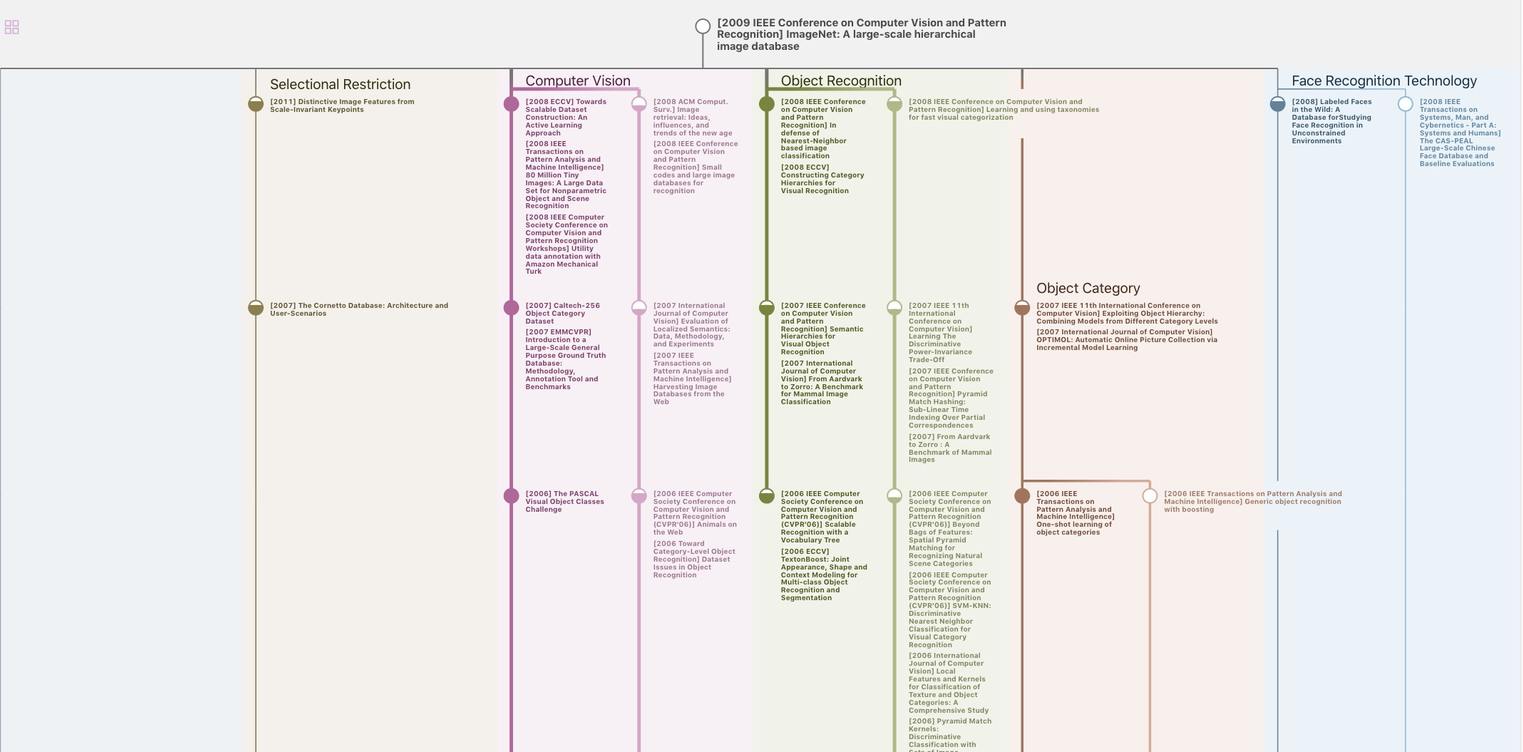
生成溯源树,研究论文发展脉络
Chat Paper
正在生成论文摘要