Meta-learning as a promising approach for few-shot cross-domain fault diagnosis: Algorithms, applications, and prospects
Knowledge-Based Systems(2022)
摘要
The advances of intelligent fault diagnosis in recent years show that deep learning has strong capability of automatic feature extraction and accurate identification for fault signals. Nevertheless, data scarcity and varying working conditions can degrade the performance of the model. More recently, a tool has been proposed to address the above challenges simultaneously. Meta-learning, also known as learning to learn, uses a small sample to quickly adapt to a new task. It has great application potential in few-shot and cross-domain fault diagnosis, and thus has become a promising tool. However, there is a lack of a survey to conclude existing work and look into the future. This paper comprehensively investigates deep meta-learning in fault diagnosis from three views: (i) what to use, (ii) how to use, and (iii) how to develop, i.e. algorithms, applications, and prospects. Algorithms are illustrated by optimization-, metric-, and model-based methods, the applications are concluded in few-shot cross-domain fault diagnosis, and open challenges, as well as prospects, are given to motivate the future work. Additionally, we demonstrate the performance of three approaches on two few-shot cross-domain tasks. Typical meta-learning methods are implemented and available at https://github.com/fyancy/MetaFD.
更多查看译文
关键词
Meta-learning,Few-shot learning,Small sample,Cross-domain,Fault diagnosis
AI 理解论文
溯源树
样例
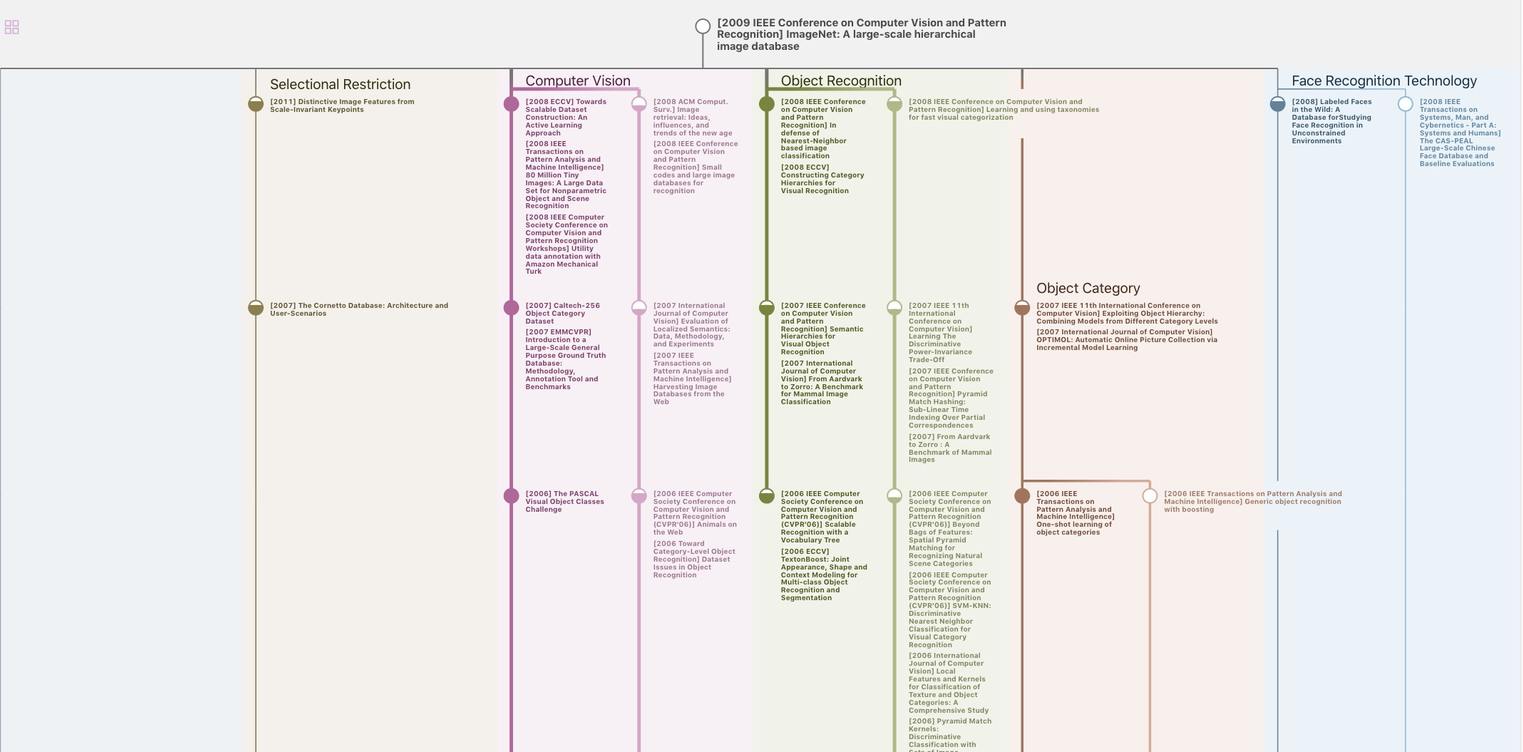
生成溯源树,研究论文发展脉络
Chat Paper
正在生成论文摘要