Graph convolutional network for protein sequence sampling and prediction using structural data
semanticscholar(2021)
摘要
The increasing integration between protein engineering and machine learning has led to many interesting results. A problem still to solve is to evaluate the likelihood that a sequence will fold into a target structure. This problem can be also viewed as sequence prediction from a known structure.In the current work, we propose improvements in the recent architecture of Geometric Vector Perceptrons [1] in order to optimize the sampling of sequences from a known backbone structure. The proposed model differs from the original in that there is: (i) no updating in the vectorial embedding, only in the scalar one, (ii) only one layer of decoding. The first aspect improves the accuracy of the model and reduces the use of memory, the second allows for training of the model with several tasks without incurring data leakage.We treat the trained classifier as an Energy-Based Model and sample sequences by sampling amino acids in a non-autoreggresive manner in the empty positions of the sequence using energy-guided criteria and followed by Monte Carlo optimization. We improve the median identity of samples from 40.2% to 44.7%.An additional question worth investigating is whether sampled and original sequences fold into similar structures independent of their identity. We chose proteins in our test set whose sampled sequences show low identity (under 30%) but for which our model predicted favorable energies. We used trRosetta server [2] and observed that the predicted structures for sampled sequences highly resemble the predicted structures for original sequences, with an average TM score of 0.848.
更多查看译文
AI 理解论文
溯源树
样例
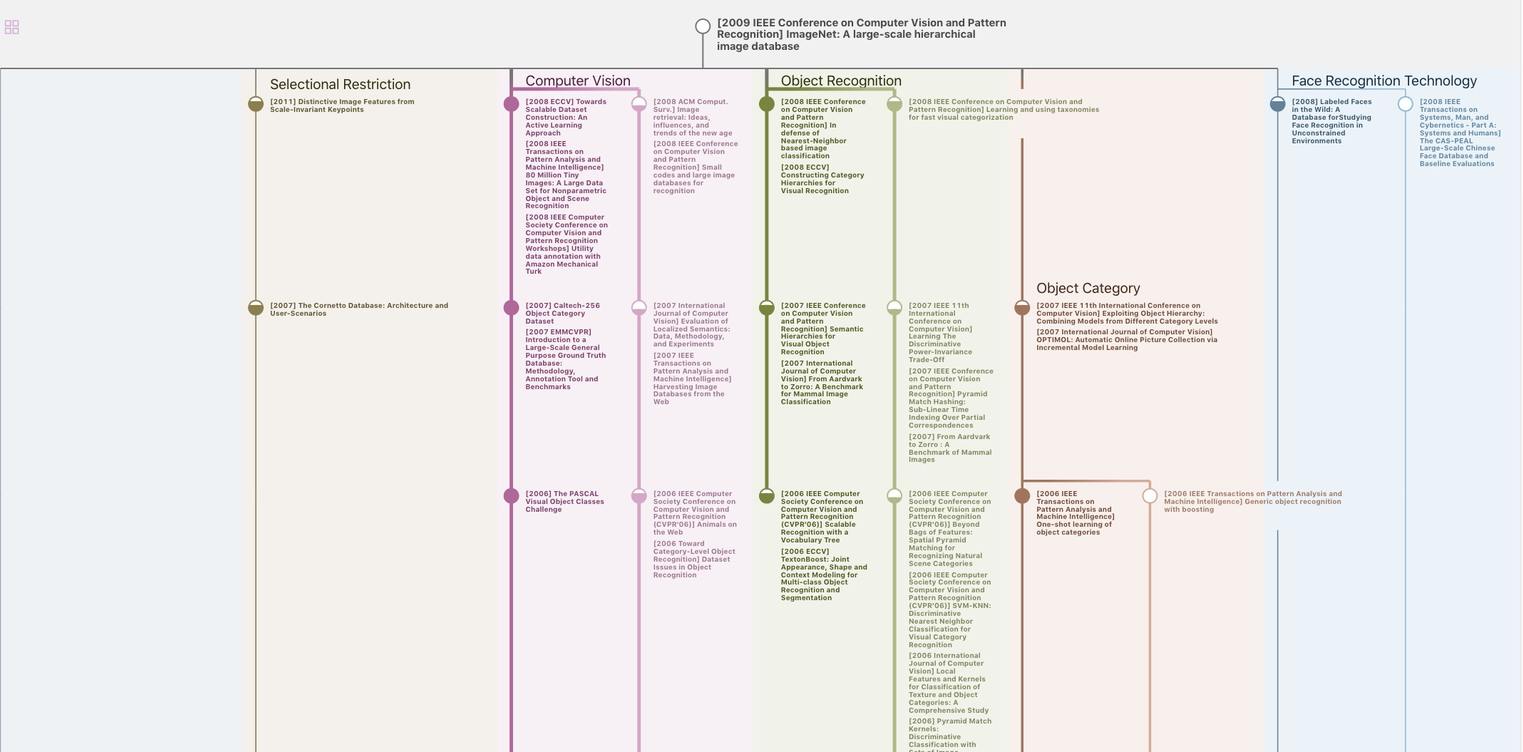
生成溯源树,研究论文发展脉络
Chat Paper
正在生成论文摘要