AN-GCN: An Anonymous Graph Convolutional Network Against Edge-Perturbing Attacks
IEEE TRANSACTIONS ON NEURAL NETWORKS AND LEARNING SYSTEMS(2024)
摘要
Recent studies have revealed the vulnerability of graph convolutional networks (GCNs) to edge-perturbing attacks, such as maliciously inserting or deleting graph edges. However, theoretical proof of such vulnerability remains a big challenge, and effective defense schemes are still open issues. In this article, we first generalize the formulation of edge-perturbing attacks and strictly prove the vulnerability of GCNs to such attacks in node classification tasks. Following this, an anonymous GCN, named AN-GCN, is proposed to defend against edge-perturbing attacks. In particular, we present a node localization theorem to demonstrate how GCNs locate nodes during their training phase. In addition, we design a staggered Gaussian noise-based node position generator and a spectral graph convolution-based discriminator (in detecting the generated node positions). Furthermore, we provide an optimization method for the designed generator and discriminator. It is demonstrated that the AN-GCN is secure against edge-perturbing attacks in node classification tasks, as AN-GCN is developed to classify nodes without the edge information (making it impossible for attackers to perturb edges anymore). Extensive evaluations verify the effectiveness of the general edge-perturbing attack (G-EPA) model in manipulating the classification results of the target nodes. More importantly, the proposed AN-GCN can achieve 82.7% in node classification accuracy without the edge-reading permission, which outperforms the state-of-the-art GCN.
更多查看译文
关键词
Perturbation methods,Image edge detection,Training,Task analysis,Location awareness,Adaptation models,Robustness,Anonymous classification,general attack model,graph adversarial attacks,graph convolutional network (GCN)
AI 理解论文
溯源树
样例
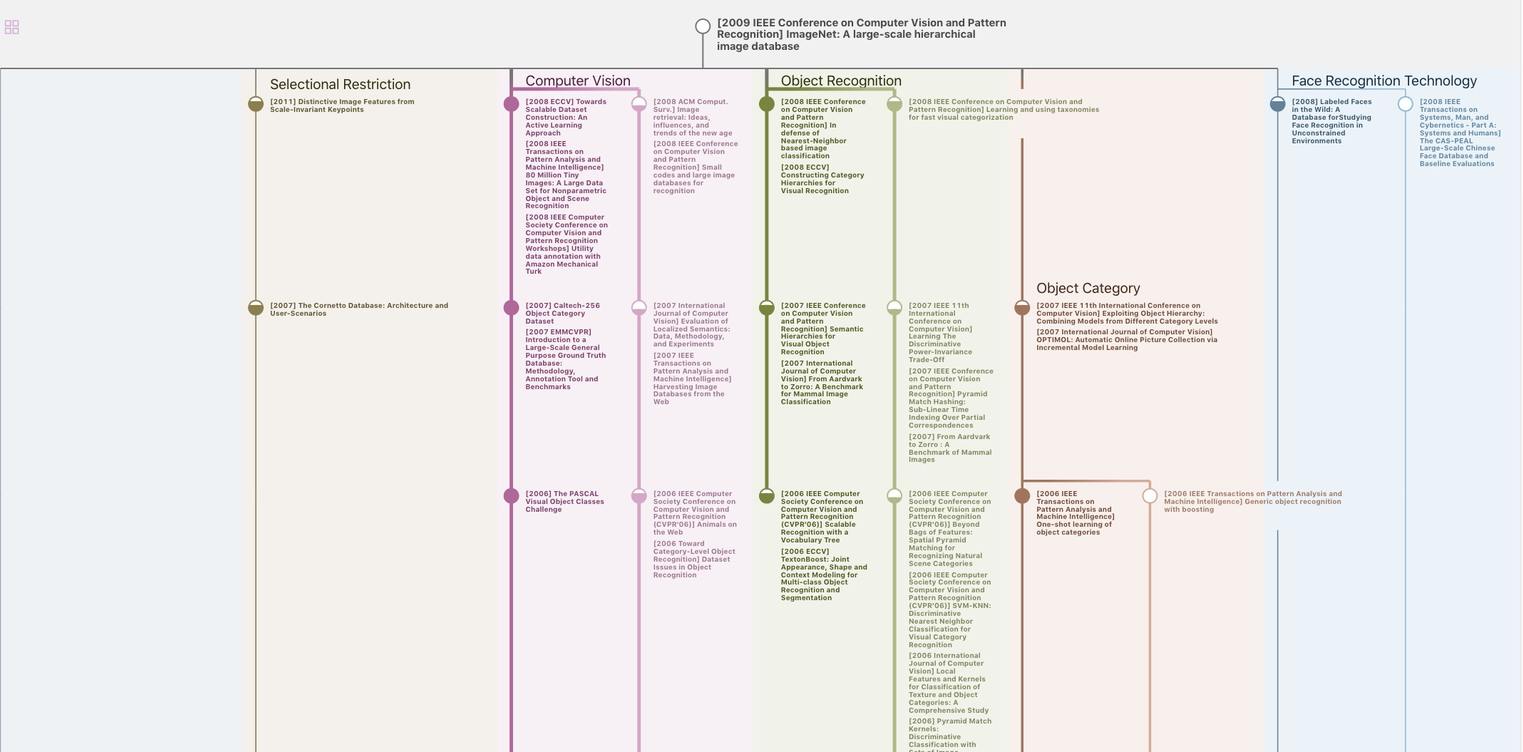
生成溯源树,研究论文发展脉络
Chat Paper
正在生成论文摘要