Automated Semantic Segmentation of Brain Tumors using Modified UNet Architecture
semanticscholar(2021)
Abstract
There are various categories of tumors found in the human brain at different localities, each owning its features or characteristics (i.e. Sizes, shapes, and contrast) at different intervals of time. The intervention of medical images (especially brain MRI images) greatly assists in the identification and categorization of these tumors. Delicate analysis and precise segmentation of these lesions are crucial for the proper diagnosis of this life-threatening disease. A lot of time and effort is required to perform this task manually, which may also be error prone. A plethora of work has been done in the near past, for the automation of this task by employing image processing, machine and deep learning-based methods. Due to high performance, deep learning-based methods have diverted the focus of the research community towards neural networks and their variants. In our study, we have employed generative deep learning-based encoder and decoder structure for the multi-class semantic segmentation of brain tumors. The proposed architecture is trained over a publically available BRATS-2015 multi-modality brain MRI image dataset. To reduce the computational cost, the largest-shortest bounding box has been extracted, which removes unused background area. The anticipated architecture has out-performed state-of-the-art semantic segmentation UNet architecture, while achieved a DICE score of 86.45%.
MoreTranslated text
AI Read Science
Must-Reading Tree
Example
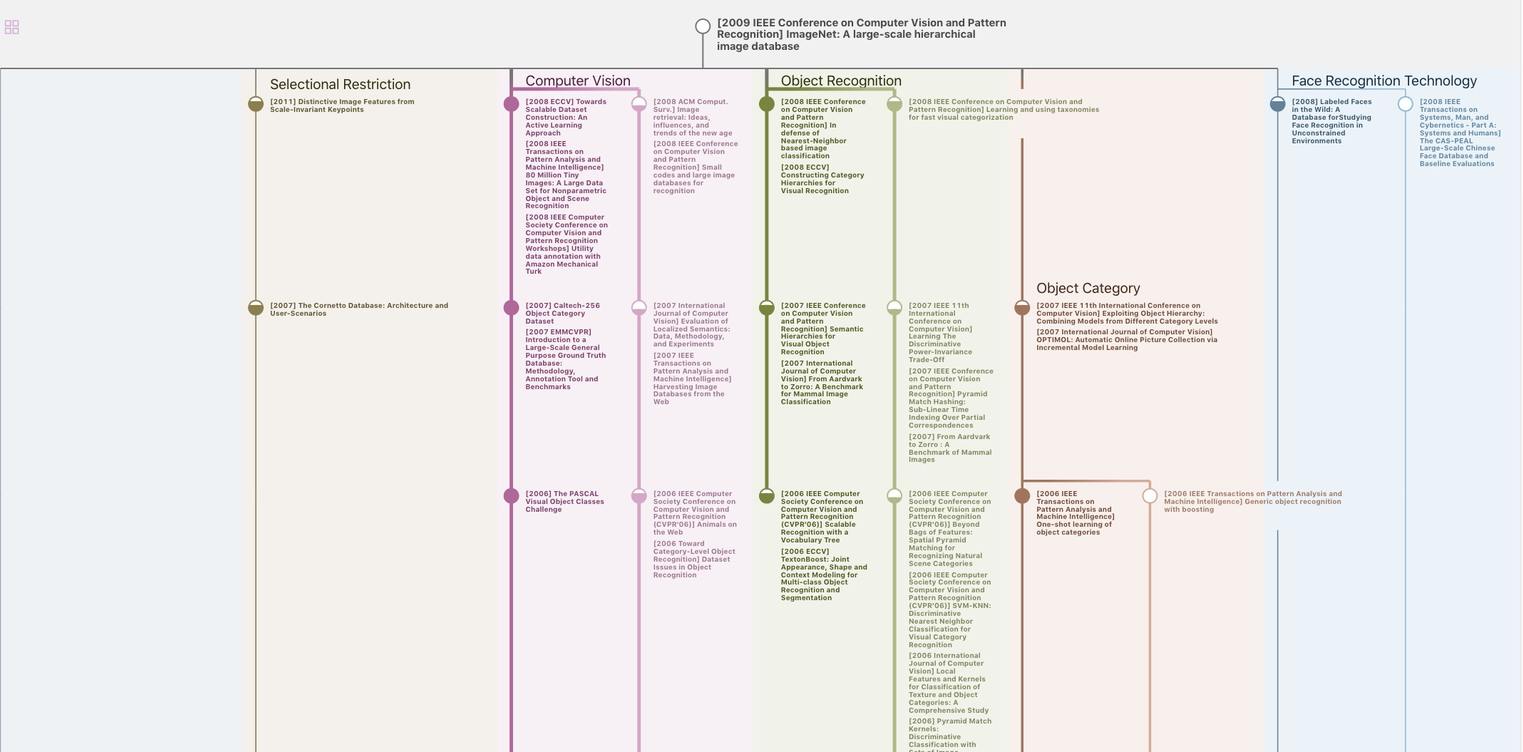
Generate MRT to find the research sequence of this paper
Chat Paper
Summary is being generated by the instructions you defined