Machine learning to predict retention and viral suppression in South African HIV treatment cohorts
medRxiv(2021)
摘要
Background: To optimize South Africa's HIV response and reach PEPFAR's 95:95:95 targets require same day initiations and patients successfully remaining on antiretroviral therapy, and remaining virally suppressed. Much effort and resources with HIV programmes have centered on tracking and tracing for those lost to follow-up (LTFU), through various back to care strategies to ensure retention of patients. However, programmes have noted the need for targeted, data driven and predictive approaches for identifying most at risk of patients for LTFU and viral unsuppression outcomes using available phenotypic data. Methods: We applied predictive machine learning algorithms to anonymised, patient-level HIV programmatic data from two districts in South Africa, January 2016 - July 2019. We developed patient risk scores for two outcomes: 1) visit attendance within 28 days of next scheduled clinic visit and 2) suppression of next HIV viral load (VL). Demographic, clinical, behavioural (e.g. visit patterns) and laboratory data were investigated in multiple models as potential predictor variables of attending next scheduled visit and VL result at next visit. Findings: Longitudinal data was analysed using combinations of predictor features and classification algorithms. 445,636 Patients were included in the retention model and 363,977 in the VL model. Predictive accuracy, summarized by Area Under the Curve (AUC), ranged from 0.69 for attendance at next scheduled visit to 0.76 for VL suppression, suggesting the model correctly classified patients to whether a scheduled visit would be attended in 2 out of 3 patients and whether the VL result at next test would be suppressed in approximately 3 out of 4 patients. Key predictors for both retention & VL suppression include metrics on prior late or LTFU visits, the number of prior VL tests, the time since their last visit, the number of visits on their current regimen, the patients' age, and their time on treatment. For retention, the number of visits at the current facility and the details of the next appointment date were also predictors. For VL suppression, other predictors included the range of thee previous VL value. Conclusion: Machine learning can be used to identify HIV patients at risk for defaulting on treatment and not being virally suppressed. Predictive modelling can improve targeting of interventions through differentiated models of care, increasing cost-effectiveness and improving patient outcomes.
更多查看译文
关键词
hiv,viral suppression,machine learning,retention
AI 理解论文
溯源树
样例
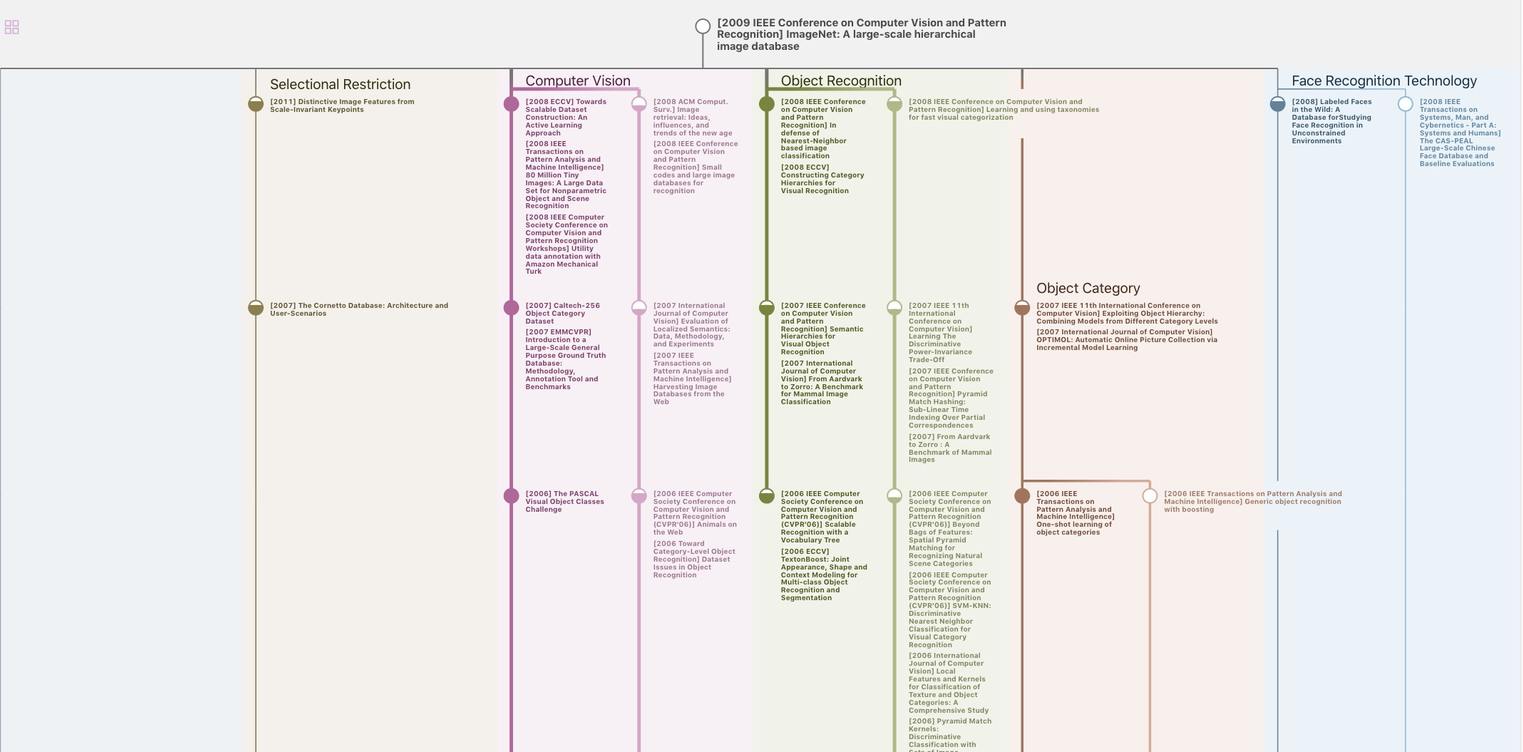
生成溯源树,研究论文发展脉络
Chat Paper
正在生成论文摘要