Numerical Algorithms for Estimating Probability Density Function Based on the Maximum Entropy Principle and Fup Basis Functions
ENTROPY(2021)
Abstract
Estimation of the probability density function from the statistical power moments presents a challenging nonlinear numerical problem posed by unbalanced nonlinearities, numerical instability and a lack of convergence, especially for larger numbers of moments. Despite many numerical improvements over the past two decades, the classical moment problem of maximum entropy (MaxEnt) is still a very demanding numerical and statistical task. Among others, it was presented how Fup basis functions with compact support can significantly improve the convergence properties of the mentioned nonlinear algorithm, but still, there is a lot of obstacles to an efficient pdf solution in different applied examples. Therefore, besides the mentioned classical nonlinear Algorithm 1, in this paper, we present a linear approximation of the MaxEnt moment problem as Algorithm 2 using exponential Fup basis functions. Algorithm 2 solves the linear problem, satisfying only the proposed moments, using an optimal exponential tension parameter that maximizes Shannon entropy. Algorithm 2 is very efficient for larger numbers of moments and especially for skewed pdfs. Since both Algorithms have pros and cons, a hybrid strategy is proposed to combine their best approximation properties.
MoreTranslated text
Key words
maximum entropy, Shannon entropy, Fup basis functions, probability density function
AI Read Science
Must-Reading Tree
Example
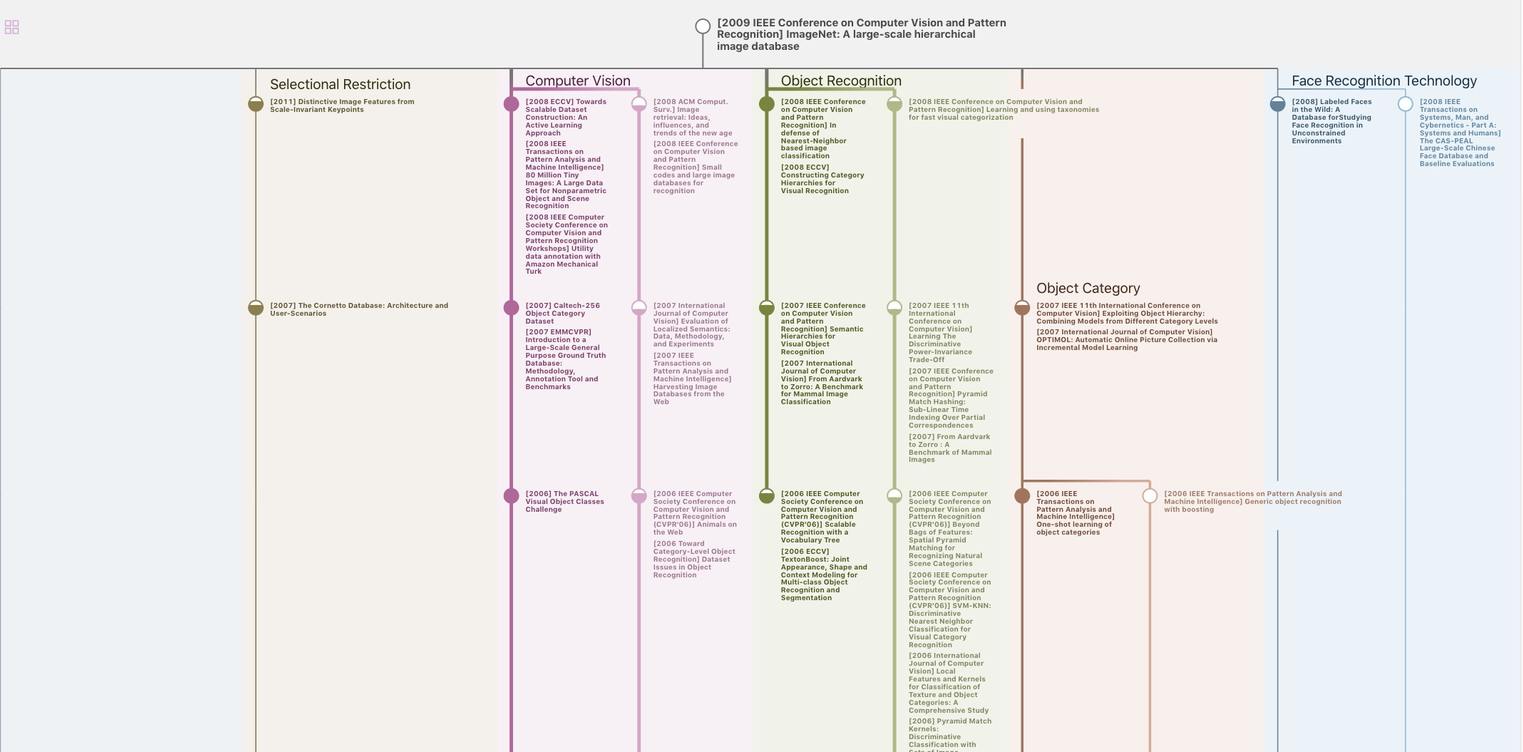
Generate MRT to find the research sequence of this paper
Chat Paper
Summary is being generated by the instructions you defined