Building a trustworthy AI differential diagnosis application for Crohn’s disease and intestinal tuberculosis
BMC medical informatics and decision making(2023)
摘要
Background Differentiating between Crohn’s disease (CD) and intestinal tuberculosis (ITB) with endoscopy is challenging. We aim to perform more accurate endoscopic diagnosis between CD and ITB by building a trustworthy AI differential diagnosis application. Methods A total of 1271 electronic health record (EHR) patients who had undergone colonoscopies at Peking Union Medical College Hospital (PUMCH) and were clinically diagnosed with CD ( n = 875) or ITB ( n = 396) were used in this study. We build a workflow to make diagnoses with EHRs and mine differential diagnosis features; this involves finetuning the pretrained language models, distilling them into a light and efficient TextCNN model, interpreting the neural network and selecting differential attribution features, and then adopting manual feature checking and carrying out debias training. Results The accuracy of debiased TextCNN on differential diagnosis between CD and ITB is 0.83 (CR F1: 0.87, ITB F1: 0.77), which is the best among the baselines. On the noisy validation set, its accuracy was 0.70 (CR F1: 0.87, ITB: 0.69), which was significantly higher than that of models without debias. We also find that the debiased model more easily mines the diagnostically significant features. The debiased TextCNN unearthed 39 diagnostic features in the form of phrases, 17 of which were key diagnostic features recognized by the guidelines. Conclusion We build a trustworthy AI differential diagnosis application for differentiating between CD and ITB focusing on accuracy, interpretability and robustness. The classifiers perform well, and the features which had statistical significance were in agreement with clinical guidelines.
更多查看译文
关键词
Neural network,Integrated gradients,Knowledge distillation,Crohn’s disease,Intestinal tuberculosis
AI 理解论文
溯源树
样例
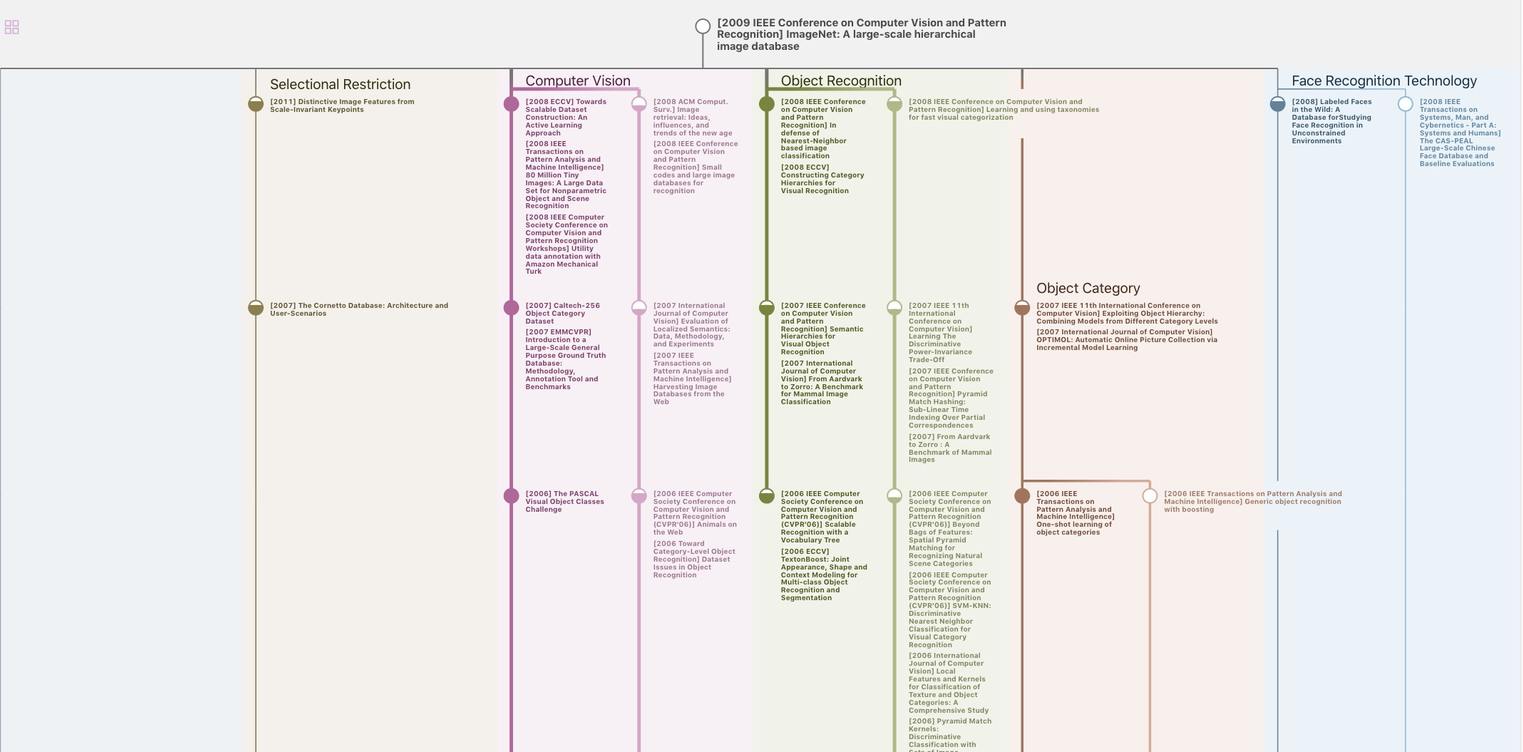
生成溯源树,研究论文发展脉络
Chat Paper
正在生成论文摘要