Applying machine learning methods to predict geology using soil sample geochemistry
Geological Society of America Abstracts with Programs(2020)
Abstract
In this study we compared various machine learning techniques that used soil geochemistry to aid in geologic mapping. We tested six different sampling methods (undersample, oversample, Synthetic Minority Oversampling Technique (SMOTE), Adaptive Synthetic Sampling (ADASYN), SMOTE and Edited Nearest Neighbor (SMOTEENN), and SMOTE and Tomek links (SMOTETomek)). SMOTE performed best with ADASYN and SMOTETomek having slightly lower effectiveness. Nine machine learning algorithms (naïve Bayes, logistic regression, quadratic discriminant analysis, nearest neighbors, radial basis function support-vector machine, artificial neural network, random forest, AdaBoost classifier, and gradient boosting classifier) were compared and AdaBoost classifiers and gradient boosting classifiers were found to be most effective. Finally, we experimented with multiple classifier systems (MCS) testing different combinations of algorithms and various combinatorial functions. It was found that MCS can outperform individual models, and the best MCS combined nearest neighbors, radial basis function support-vector machine, artificial neural network, random forest, AdaBoost classifiers, and gradient boosting classifier, then applied a logistic regression to the probabilities output by the models. Ultimately, we created a tool that is able to adequately predict underlying geology in the study area using soil geochemistry.
MoreTranslated text
Key words
Geological mapping,Soil geochemistry,Data science,Machine learning,Sampling method,Multiple classifier system
AI Read Science
Must-Reading Tree
Example
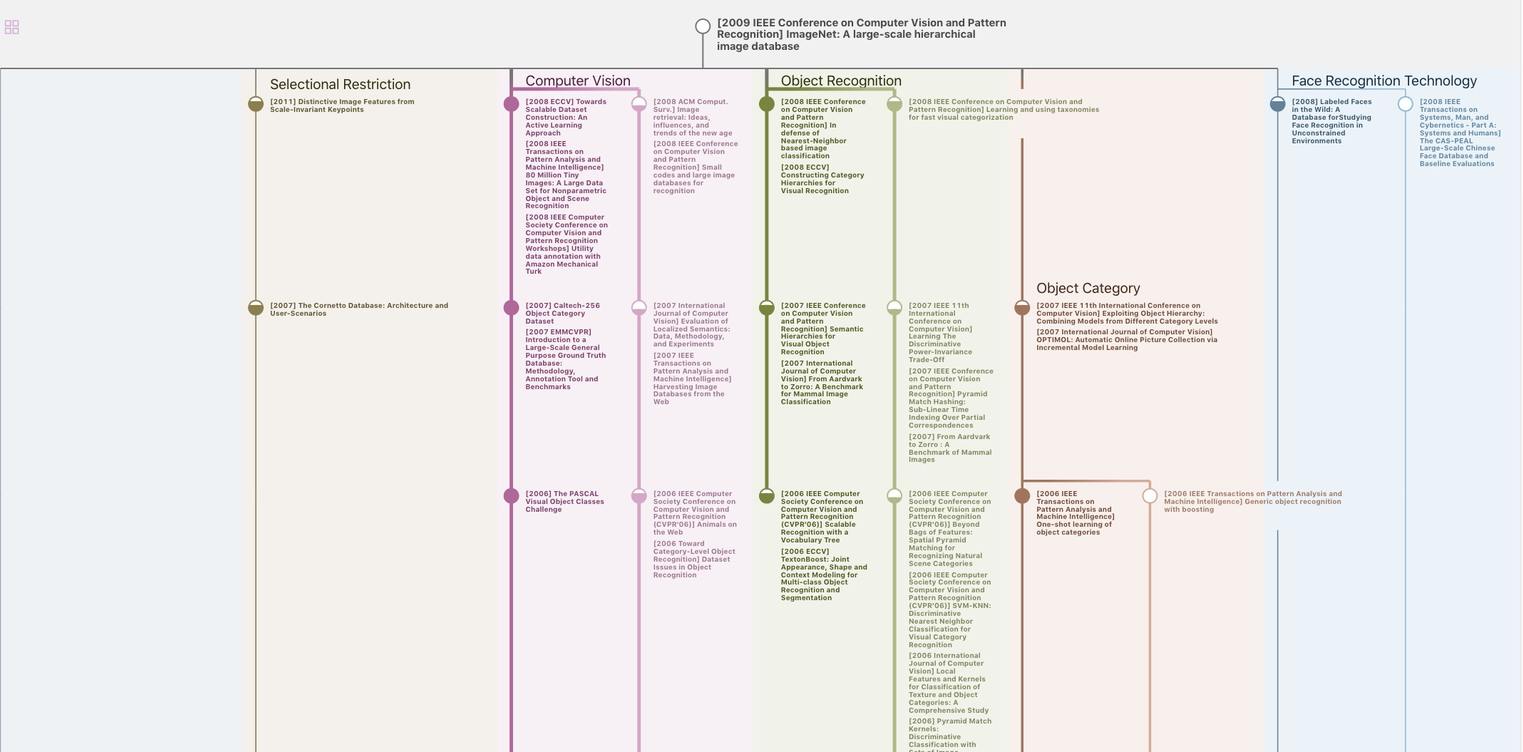
Generate MRT to find the research sequence of this paper
Chat Paper
Summary is being generated by the instructions you defined