Predicting mobile government service continuance: A two-stage structural equation modeling-artificial neural network approach
Government Information Quarterly(2022)
Abstract
Retaining users of mobile government services (MGS) is critical to the success of mobile government. Drawing on Bagozzi's self-regulation framework, the current research investigates the predictors of MGS continuance by considering both perceived quality and perceived value. Unlike most e-government research that has utilized linear models, this study employed a two-stage structural equation modeling-artificial neural network approach that can identify non-linear and non-compensatory interactions. The current research first applied a structural equation model to examine the significant factors influencing MGS continuance, and then utilized a neural network model recheck the structural equation modeling findings and rank the importance of these factors. Data were obtained from 335 "one-stop-shop service platform" app users in China through an online survey platform. The research findings reveal that user satisfaction is the utmost influential determinant of their continuous-use intention regarding MGS, followed by user trust; moreover, perceived quality and perceived value strongly influence satisfaction and trust. However, perceived risk does not affect intention to continue use. We also discuss theoretical and practical implications for government agencies and service providers, for improving user continuance rates for MGS.
MoreTranslated text
Key words
Mobile government service,Continuance intention,Perceived quality,Perceived value,User satisfaction,User trust
AI Read Science
Must-Reading Tree
Example
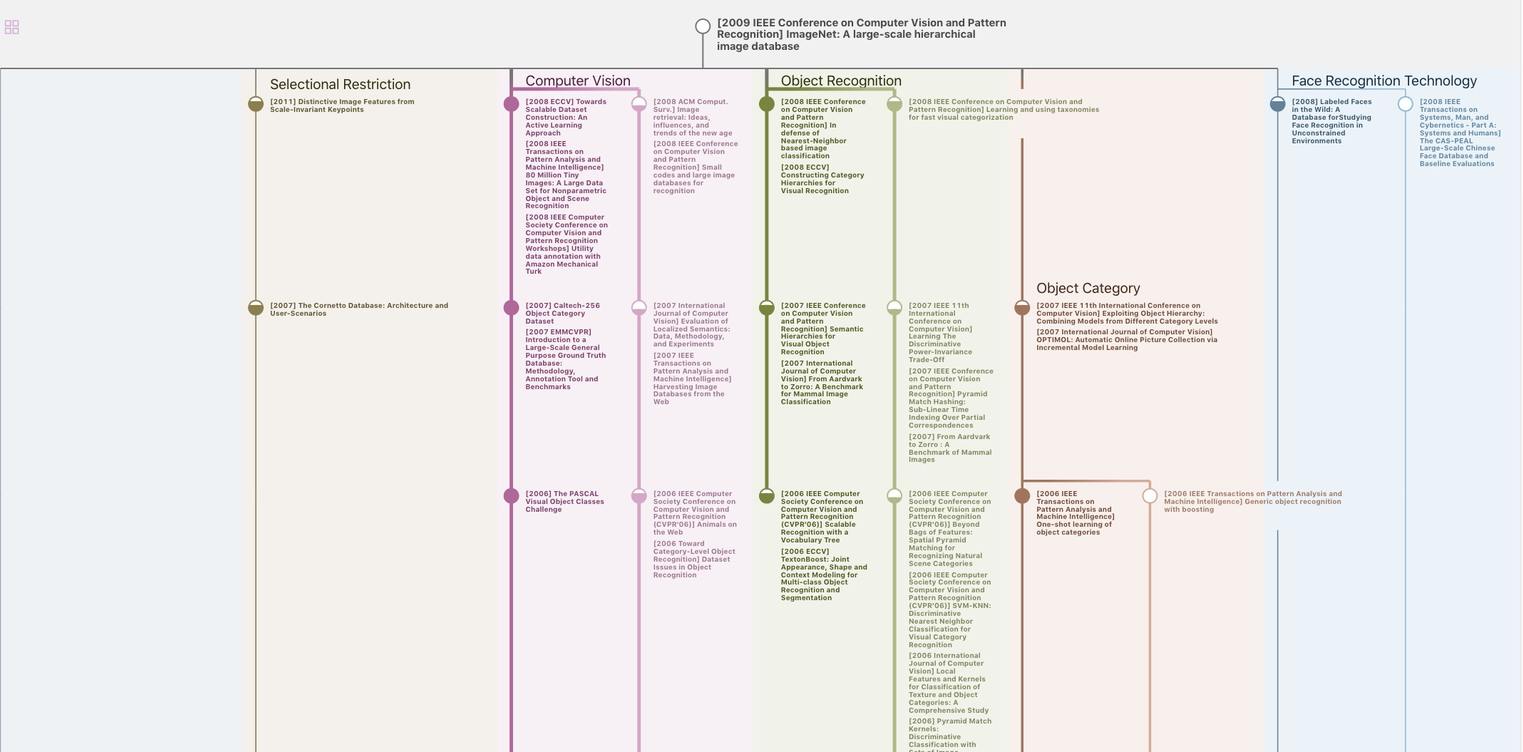
Generate MRT to find the research sequence of this paper
Chat Paper
Summary is being generated by the instructions you defined