Use of real-time artificial intelligence in detection of abnormal image patterns in standard sonographic reference planes in screening for fetal intracranial malformations
ULTRASOUND IN OBSTETRICS & GYNECOLOGY(2022)
Abstract
Objectives To develop and validate an artificial intelligence system, the Prenatal ultrasound diagnosis Artificial Intelligence Conduct System (PAICS), to detect different patterns of fetal intracranial abnormality in standard sonographic reference planes for screening for congenital central nervous system (CNS) malformations. Methods Neurosonographic images from normal fetuses and fetuses with CNS malformations at 18-40 gestational weeks were retrieved from the databases of two tertiary hospitals in China and assigned randomly (ratio, 8:1:1) to training, fine-tuning and internal validation datasets to develop and evaluate the PAICS. The system was built based on a real-time convolutional neural network (CNN) algorithm, You Only Look Once, version 3 (YOLOv3). An image dataset from a third tertiary hospital was used to further validate, externally, the performance of the PAICS and to compare its performance with that of sonologists with different levels of expertise. Furthermore, a prospective video dataset was employed to evaluate the performance of the PAICS in a real-time scan scenario. The diagnostic accuracy, sensitivity, specificity and area under the receiver-operating-characteristics curve (AUC) were calculated to assess the performance of the PAICS and to compare this with the performance of sonologists with different levels of experience. Results In total, 43 890 images from 16 297 pregnancies and 169 videos from 166 pregnancies were used to develop and validate the PAICS. The system achieved excellent performance in identifying 10 types of intracranial image pattern, with macro- and microaverage AUCs, respectively, of 0.933 (95% CI, 0.798-1.000) and 0.977 (95% CI, 0.970-0.985) for the internal validation image dataset, 0.902 (95% CI, 0.816-0.989) and 0.898 (95% CI, 0.885-0.911) for the external validation image dataset and 0.969 (95% CI, 0.886-1.000) and 0.981 (95% CI, 0.974-0.988) in the real-time scan setting. The performance of the PAICS was comparable to that of expert sonologists in terms of macroand microaverage accuracy (P=0.863 and P=0.775, respectively), sensitivity (P=0.883, P=0.846) and AUC (P=0.891, P=0.788), but required significantly less time (0.025 s per image for PAICS vs 4.4 s for experts, P<0.001). Conclusions Both in the image dataset and in the real-time scan setting, the PAICS achieved excellent diagnostic performance for various fetal CNS abnormalities. Its performance was comparable to that of experts, but it required less time. A CNN algorithm can be trained to detect fetal CNS abnormalities. The PAICS has the potential to be an effective and efficient tool in screening for fetal CNS malformations in clinical practice. (C) 2021 International Society of Ultrasound in Obstetrics and Gynecology.
MoreTranslated text
Key words
artificial intelligence,CNS malformation,congenital central nervous system malformation,prenatal ultrasound
AI Read Science
Must-Reading Tree
Example
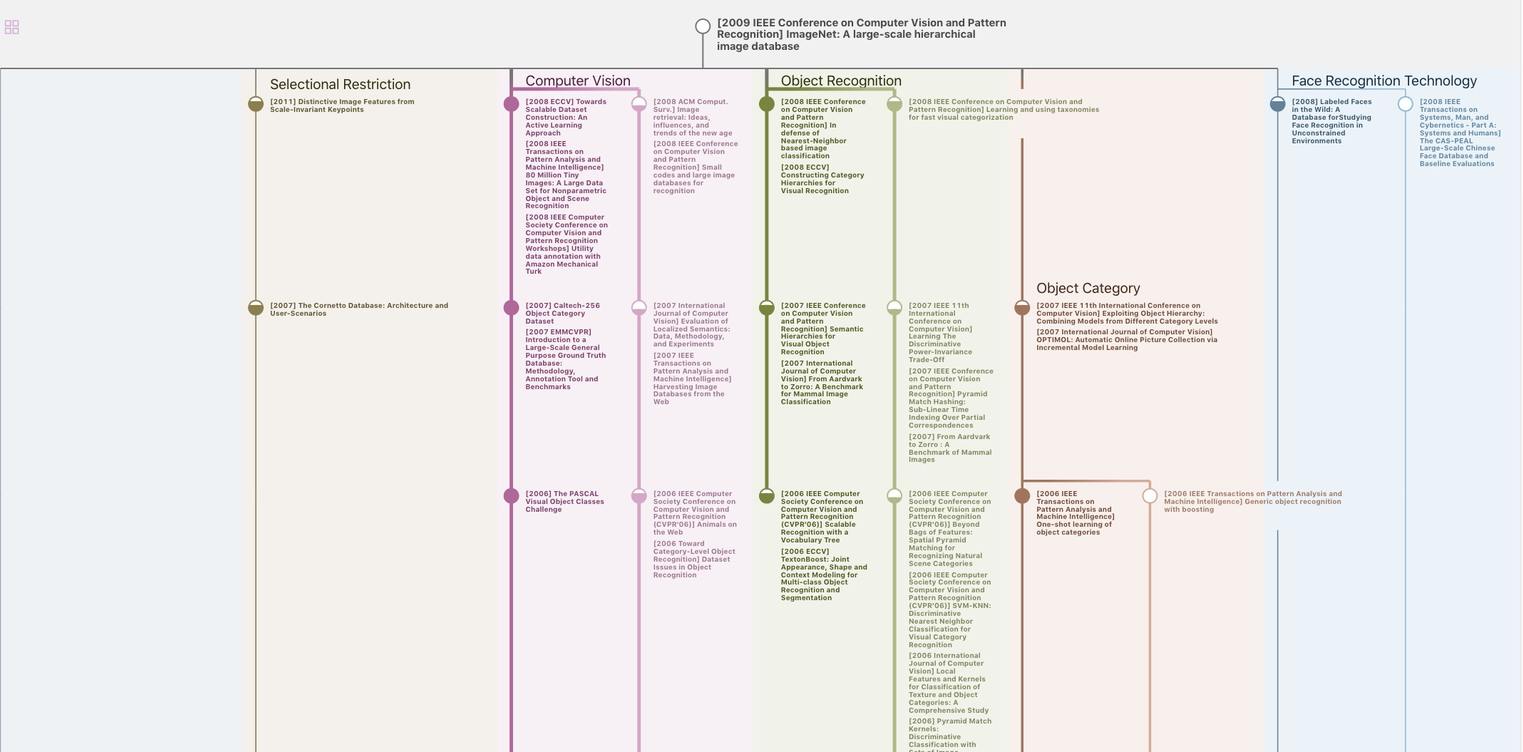
Generate MRT to find the research sequence of this paper
Chat Paper
Summary is being generated by the instructions you defined