Application of Machine Learning Techniques and Spectrum Images of Vibration Orbits for Fault Classification of Rotating Machines
Journal of Control, Automation and Electrical Systems(2021)
Abstract
A comparative analysis of machine learning techniques for fault diagnosis of rotating machines based on images of vibration spectra is presented. The feature extraction of different types of faults, including unbalance, misalignment, shaft crack, rotor–stator rubbing, and hydrodynamic instability, is performed by processing spectral images of vibration orbits acquired during the machine run-up. The classifiers are trained with simulated data and tested with both simulated and experimental data. The latter are obtained from laboratory measurements performed on an rotor-disc system supported on hydrodynamic bearings. To generate the simulated data, a numerical model is developed using the finite element method. Deep learning, ensemble and traditional classification methods are evaluated. The ability of the methods to generalize the image classification is evaluated based on their performance in classifying experimental test patterns that were not used during training. The results of this research indicate that, despite considerable computational cost, the method based on convolutional neural networks presents the best performance.
MoreTranslated text
Key words
Rotating machines, Machine fault diagnosis, Spectral image, Vibration, Machine learning
AI Read Science
Must-Reading Tree
Example
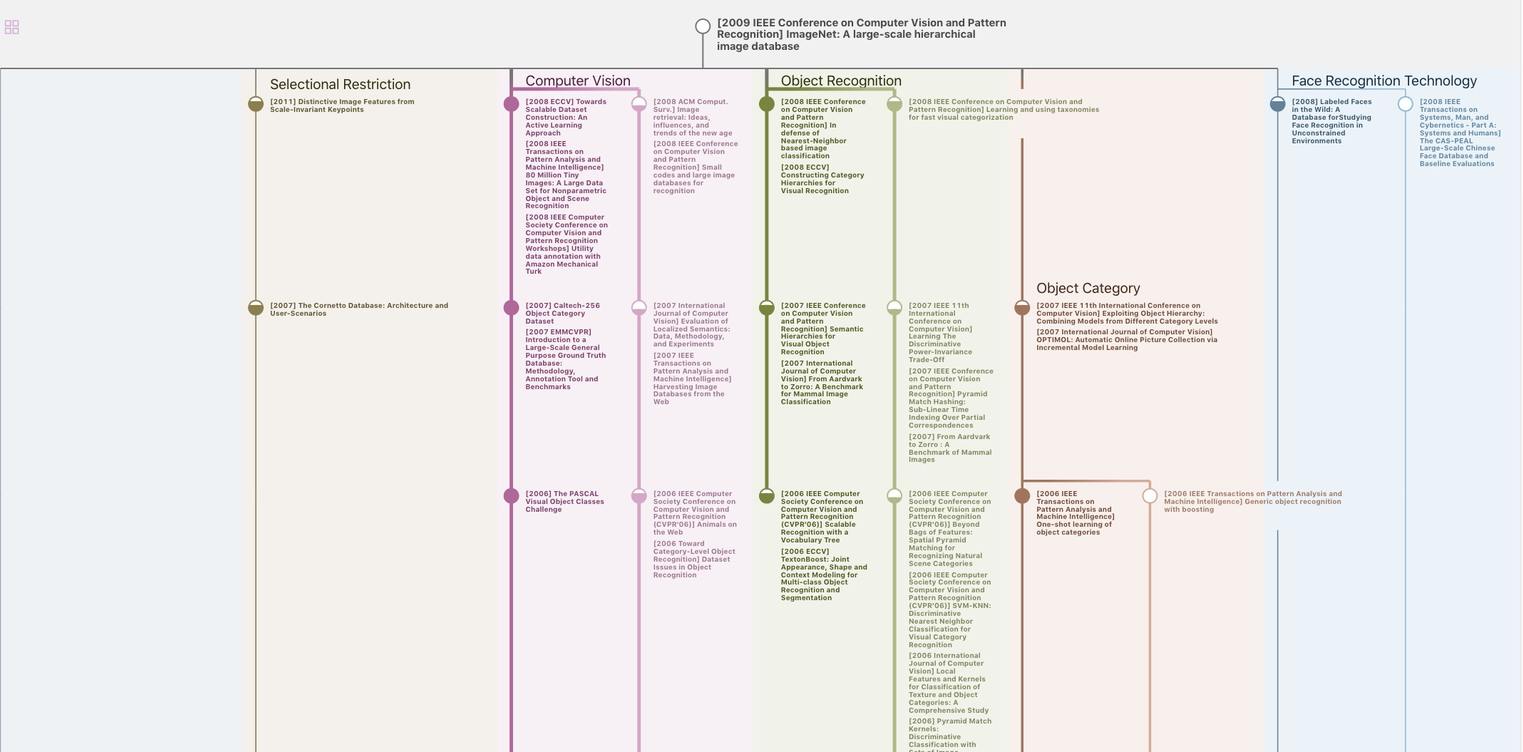
Generate MRT to find the research sequence of this paper
Chat Paper
Summary is being generated by the instructions you defined