ModelRevelator: Fast phylogenetic model estimation via deep learning
biorxiv(2023)
摘要
Selecting the best model of sequence evolution for a multiple-sequence-alignment (MSA) constitutes the first step of phylogenetic tree reconstruction. Common approaches for inferring nucleotide models typically apply maximum likelihood (ML) methods, with discrimination between models determined by one of several information criteria. This requires tree reconstruction and optimisation which can be computationally expensive. We demonstrate that neural networks can be used to perform model selection, without the need to reconstruct trees, optimise parameters, or calculate likelihoods.We introduce ModelRevelator, a model selection tool underpinned by two deep neural networks. The first neural network, NNmodelfind, recommends one of six commonly used models of sequence evolution, ranging in complexity from Jukes and Cantor to General Time Reversible. The second, NNalphafind, recommends whether or not a Gamma-distributed rate heterogeneous model should be incorporated, and if so, provides an estimate of the shape parameter, alpha. Users can simply input an MSA into ModelRevelator, and swiftly receive output recommending the evolutionary model, inclusive of the presence or absence of rate heterogeneity, and an estimate of alpha.We show that ModelRevelator performs comparably with likelihood-based methods and the recently published machine learning method ModelTeller over a wide range of parameter settings, with significant potential savings in computational effort. Further, we show that this performance is not restricted to the alignments on which the networks were trained, but is maintained even on unseen empirical data. We expect that ModelRevelator will provide a valuable alternative for phylogeneticists, especially where traditional methods of model selection are computationally prohibitive.
更多查看译文
关键词
Phylogenetic model estimation,Deep learning,Artificial intelligence,Phylogenetics,Phylogenomics
AI 理解论文
溯源树
样例
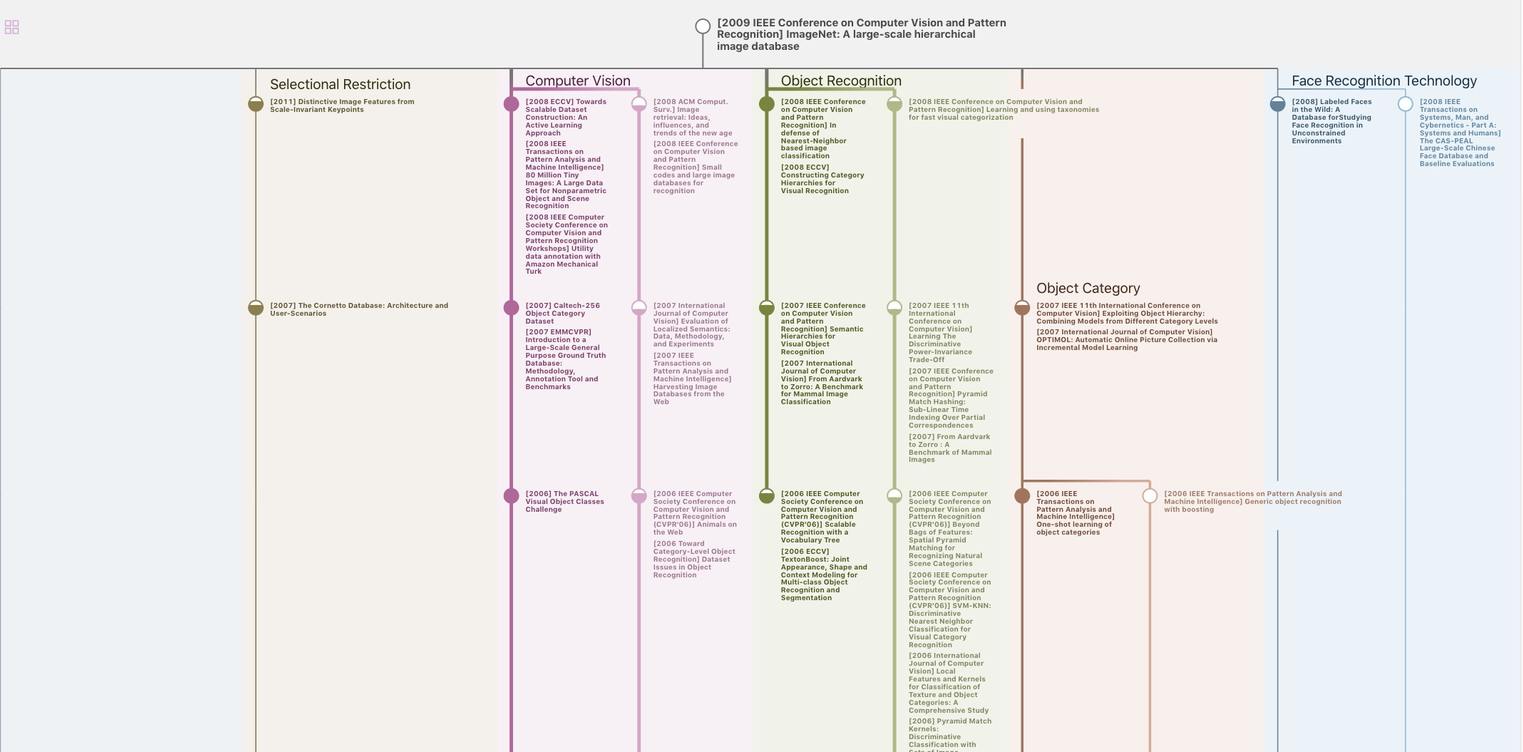
生成溯源树,研究论文发展脉络
Chat Paper
正在生成论文摘要