Curriculum learning for safe mapless navigation.
ACM Symposium on Applied Computing (SAC)(2022)
摘要
This work investigates the effects of Curriculum Learning (CL)-based approaches on the agent's performance. In particular, we focus on the safety aspect of robotic mapless navigation, comparing over a standard end-to-end (E2E) training strategy. To this end, we present a CL approach that leverages Transfer of Learning (ToL) and fine-tuning in a Unity-based simulation with the Robotnik Kairos as a robotic agent. For a fair comparison, our evaluation considers an equal computational demand for every learning approach (i.e., the same number of interactions and difficulty of the environments) and confirms that our CL-based method that uses ToL outperforms the E2E methodology. In particular, we improve the average success rate and the safety of the trained policy, resulting in 10% fewer collisions in unseen testing scenarios. To further confirm these results, we employ a formal verification tool to quantify the number of correct behaviors of Reinforcement Learning policies over desired specifications.
更多查看译文
关键词
Deep Reinforcement Learning (DRL), Curriculum Learning (CL), Transfer of Learning (ToL), Fine-tuning, Mapless Navigation, Formal verification
AI 理解论文
溯源树
样例
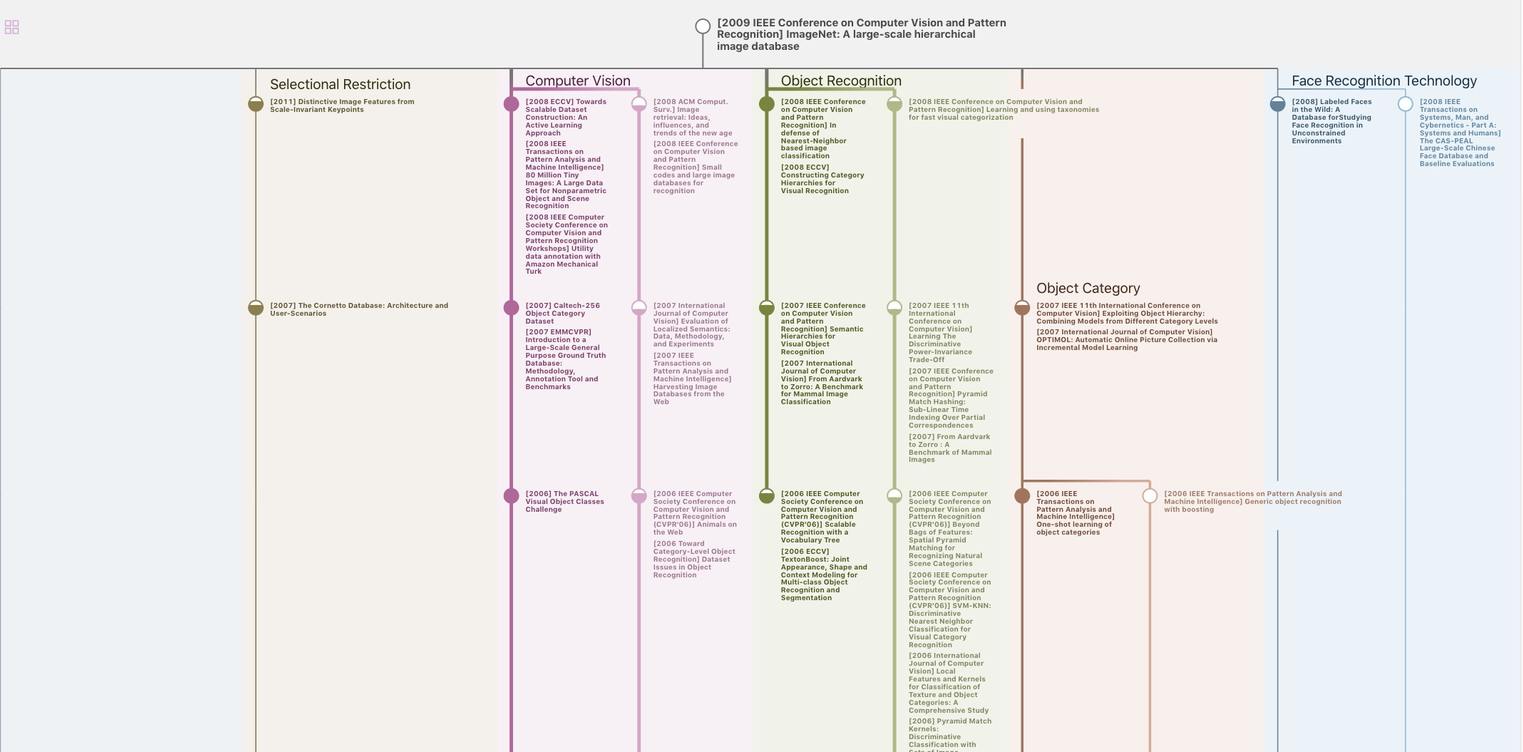
生成溯源树,研究论文发展脉络
Chat Paper
正在生成论文摘要