Few-Shot Object Detection: A Comprehensive Survey
ArXiv(2023)
Abstract
Humans are able to learn to recognize new objects even from a few examples. In contrast, training deep-learning-based object detectors requires huge amounts of annotated data. To avoid the need to acquire and annotate these huge amounts of data, few-shot object detection (FSOD) aims to learn from few object instances of new categories in the target domain. In this survey, we provide an overview of the state of the art in FSOD. We categorize approaches according to their training scheme and architectural layout. For each type of approach, we describe the general realization as well as concepts to improve the performance on novel categories. Whenever appropriate, we give short takeaways regarding these concepts in order to highlight the best ideas. Eventually, we introduce commonly used datasets and their evaluation protocols and analyze the reported benchmark results. As a result, we emphasize common challenges in evaluation and identify the most promising current trends in this emerging field of FSOD.
MoreTranslated text
Key words
Training,Object detection,Detectors,Benchmark testing,Transfer learning,Task analysis,Feature extraction,Few-shot learning,meta learning,object detection,survey,transfer learning
AI Read Science
Must-Reading Tree
Example
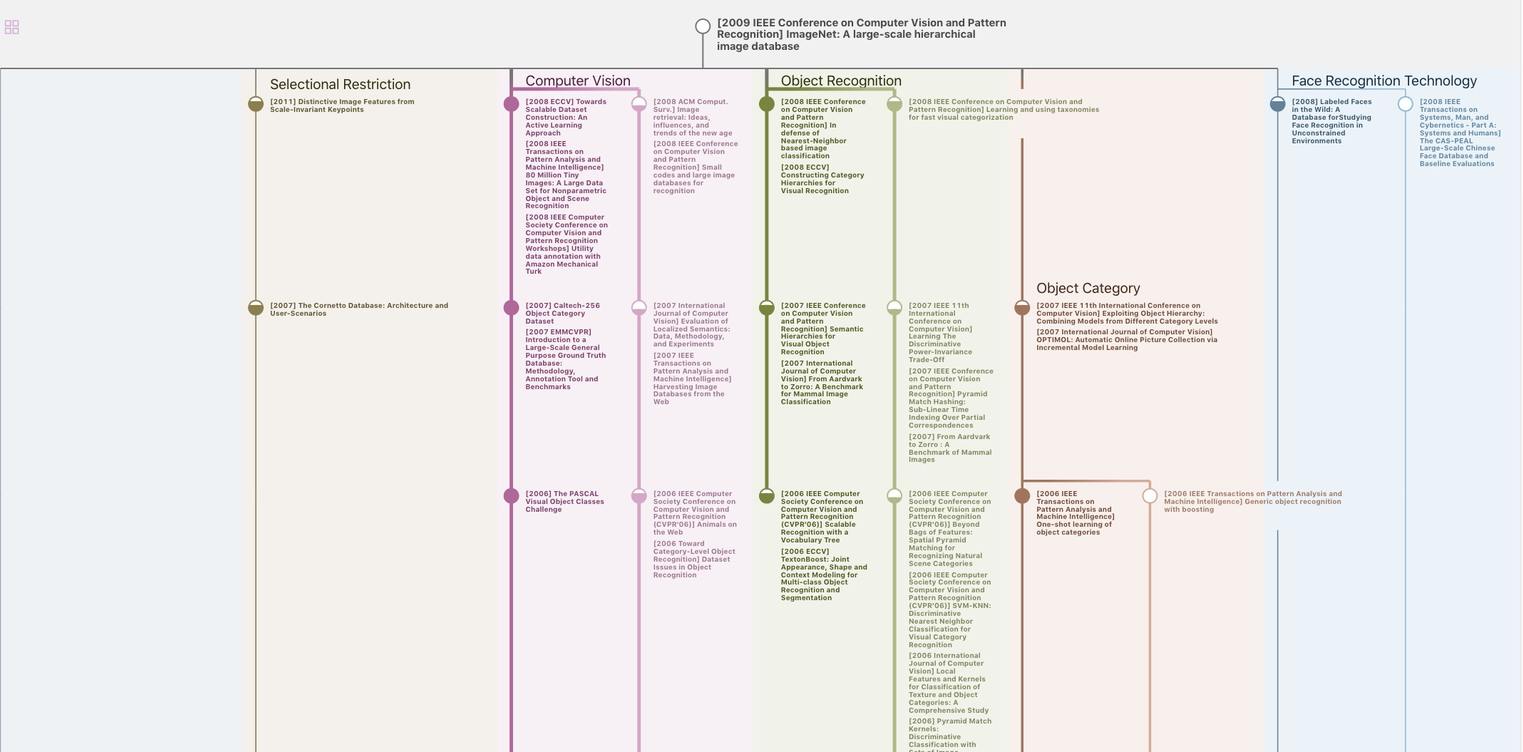
Generate MRT to find the research sequence of this paper
Chat Paper
Summary is being generated by the instructions you defined