Prediction and associated factors of hypothyroidism in systemic lupus erythematosus: a cross-sectional study based on multiple machine learning algorithms
CURRENT MEDICAL RESEARCH AND OPINION(2022)
摘要
Objectives The prevalence of hypothyroidism in systemic lupus erythematosus (SLE) is significantly higher than that in the common public. While SLE itself can affect multiple organs, abnormal thyroid function may exacerbate organ damage in patients with SLE. We aimed to predict abnormal thyroid function and to examine the associated factors with multiple machine learning approaches. Methods In a cross-sectional study, 255 patients diagnosed with SLE at the rheumatology department in Xiangya Hospital between June 2012 and December 2016 were investigated. Feature engineering was used for filtering out principle clinical parameters, and five different machine learning methods were used to build prediction models for SLE with hypothyroidism. Results Feature engineering selected 11 variables with which to build machine learning models. Among them, random forest modelling obtained the best prediction performance, with an accuracy rate of 88.37 and an area under the receiver operating characteristic curve of 0.772. The weights of anti-SSB antibody and anti-dsDNA antibody were 1.421 and 1.011, respectively, indicating a strong association with hypothyroidism in SLE. Conclusions Random Forest model performed best and is recommended for selecting vital indices and assessing clinical complications of SLE, it indicated that anti-SSB and anti-dsDNA antibodies may play principal roles in the development of hypothyroidism in SLE patients. It's feasible to build an accurate machine learning model for early diagnosis or risk factors assessment in SLE using clinical parameters, which would provide a reference for the research work of SLE in China.
更多查看译文
关键词
Feature engineering, machine learning, SLE, random forest modelling
AI 理解论文
溯源树
样例
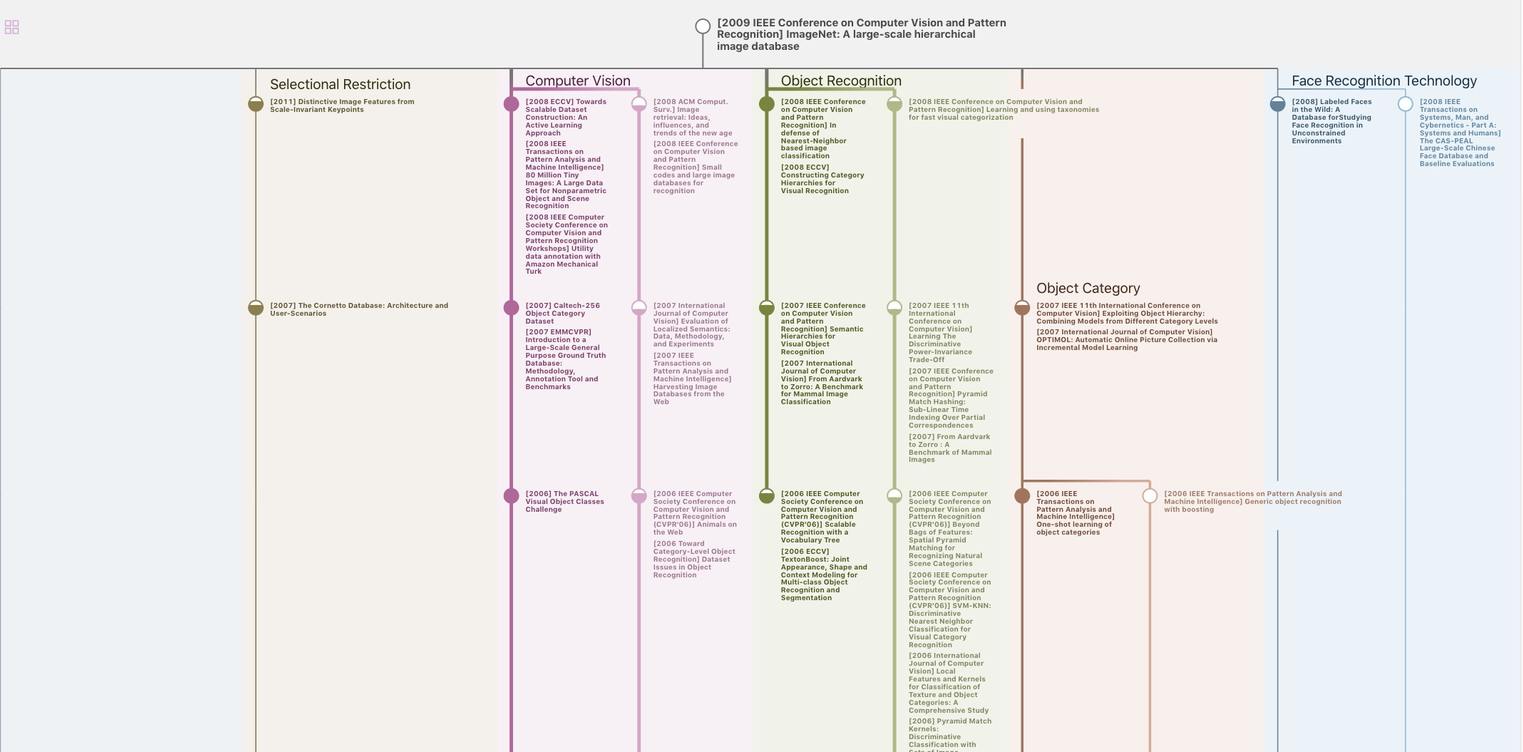
生成溯源树,研究论文发展脉络
Chat Paper
正在生成论文摘要