Group Lasso merger for sparse prediction with high-dimensional categorical data
arXiv (Cornell University)(2021)
摘要
Sparse prediction with categorical data is challenging even for a moderate number of variables, because one parameter is roughly needed to encode one category or level. The Group Lasso is a well known efficient algorithm for selection continuous or categorical variables, but all estimates related to a selected factor usually differ, so a fitted model may not be sparse. To make the Group Lasso solution sparse, we propose to merge levels of the selected factor, if a difference between its corresponding estimates is less than some predetermined threshold. We prove that under weak conditions our algorithm, called GLAMER for Group LAsso MERger, recovers the true, sparse linear or logistic model even for the high-dimensional scenario, that is when a number of parameters is greater than a learning sample size. To our knowledge, selection consistency has been proven many times for different algorithms fitting sparse models with categorical variables, but our result is the first for the high-dimensional scenario. Numerical experiments show the satisfactory performance of the GLAMER.
更多查看译文
关键词
lasso,sparse prediction,categorical data,group,high-dimensional
AI 理解论文
溯源树
样例
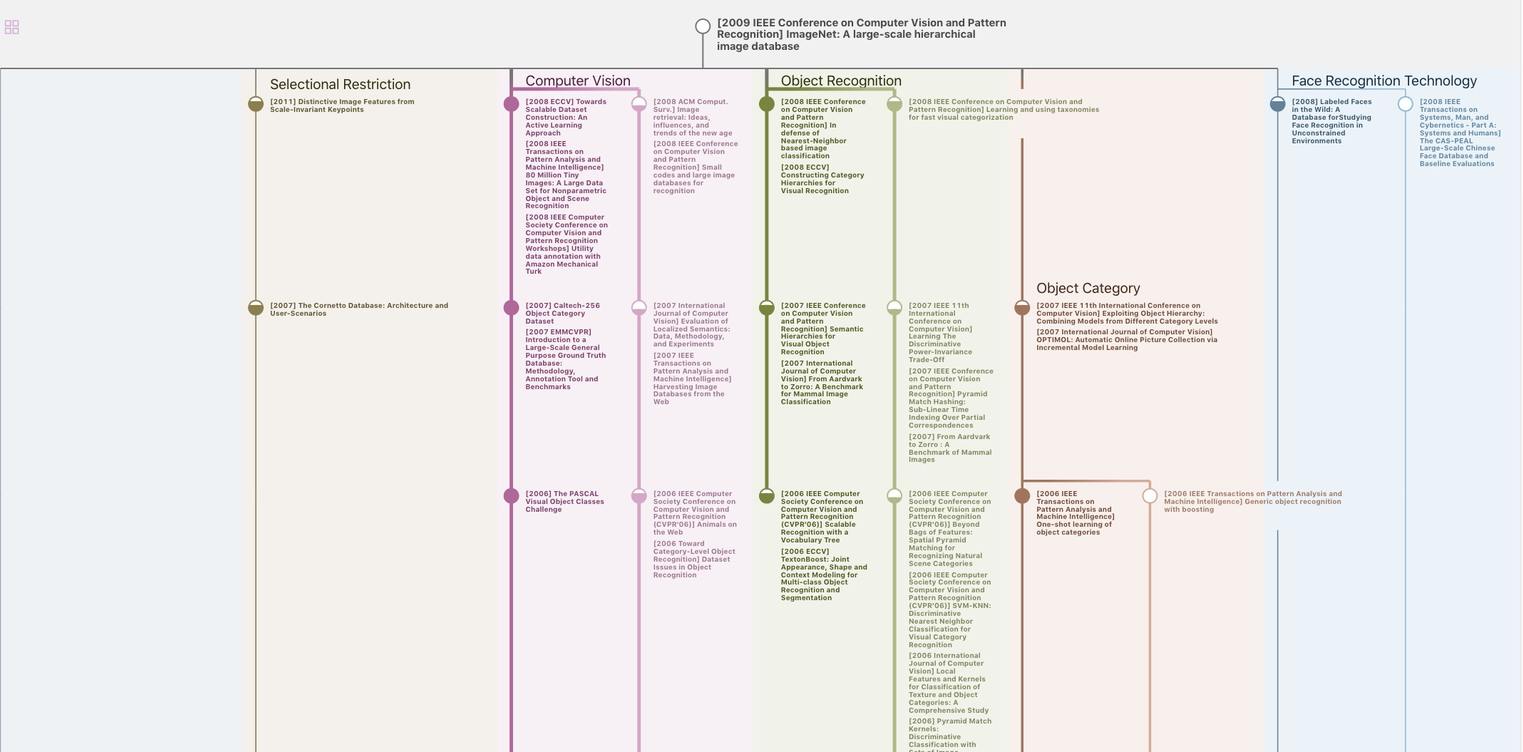
生成溯源树,研究论文发展脉络
Chat Paper
正在生成论文摘要