Stochastic-HD: Leveraging Stochastic Computing on Hyper-Dimensional Computing
2021 IEEE 39th International Conference on Computer Design (ICCD)(2021)
摘要
Brain-inspired Hyperdimensional (HD) computing is a novel and efficient computing paradigm which is more hardware-friendly than the traditional machine learning algorithms, however, the latest encoding and similarity checking schemes still require thousands of operations. To further reduce the hardware cost of HD computing, we present Stochastic-HD that combines the simplicity of operations in Stochastic Computing (SC) with the complex task solving capabilities of the latest HD computing algorithms. Stochastic-HD leverages deterministic SC, which uses structured input binary bitstreams instead of the traditional randomly generated bitstreams thus avoids expensive SC components like stochastic number generators. We also propose an in-memory hardware design for Stochastic-HD that exploits its high level of parallelism and robustness to approximation. Our hardware uses in-memory bitwise operations along with associative memory-like operations to enable a fast and energy-efficient implementation. With Stochastic-HD, we were able to reach a comparable accuracy with the Baseline-HD. As compared to the best PIM design for HD [1], Stochastic-HD is also 4.4% more accurate and 43.1x more energy-efficient.
更多查看译文
关键词
Training,Machine learning algorithms,Costs,Parallel processing,Hardware,Energy efficiency,Robustness
AI 理解论文
溯源树
样例
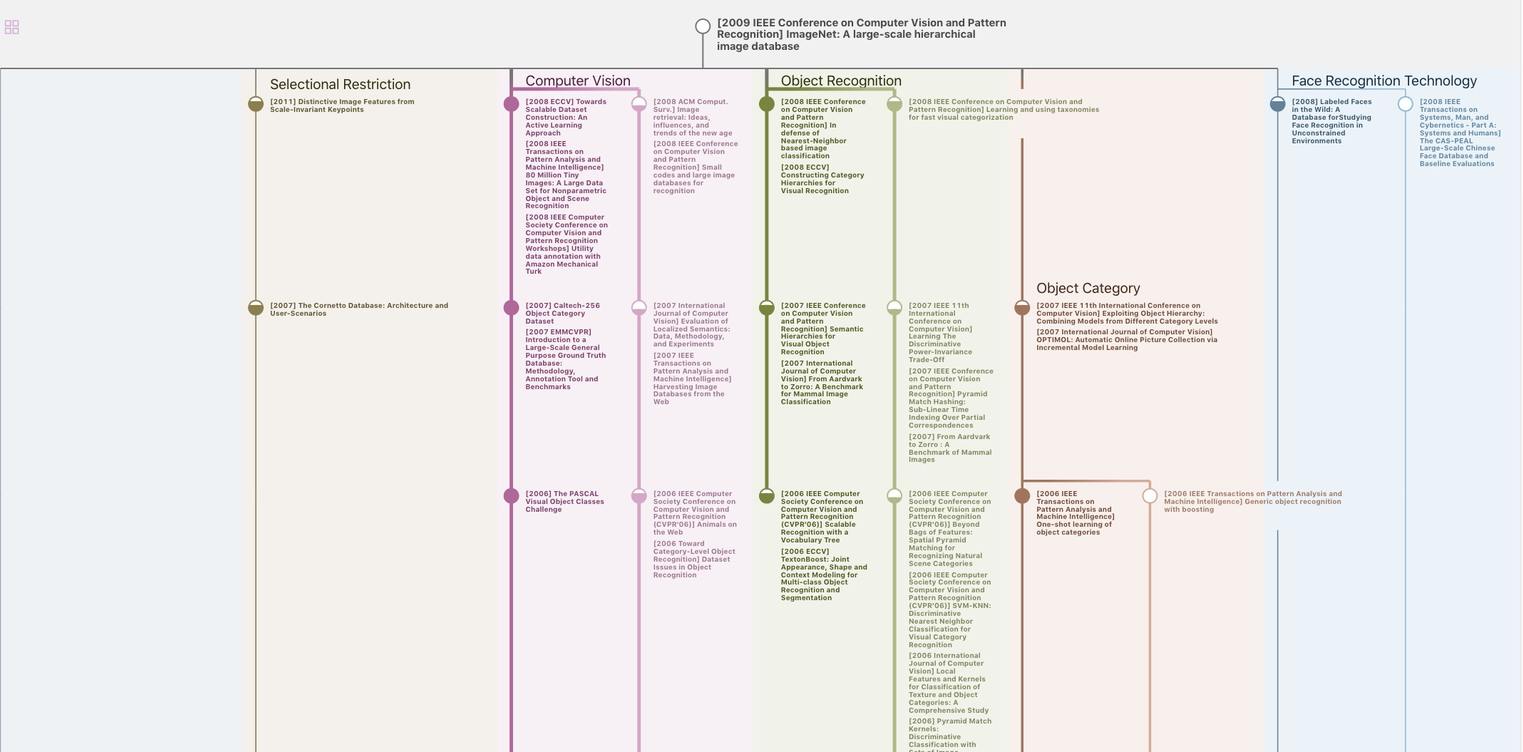
生成溯源树,研究论文发展脉络
Chat Paper
正在生成论文摘要