Efficient design of peptide-binding polymers using active learning approaches
JOURNAL OF CONTROLLED RELEASE(2023)
摘要
Active learning (AL) has become a subject of active recent research both in industry and academia as an efficient approach for rapid design and discovery of novel chemicals, materials, and polymers. Herein, we have assessed the applicability of AL for the discovery of polymeric micelle formulations for poorly soluble drugs. We were motivated by the key advantages of this approach making it a desirable strategy for rational design of drug delivery systems due toto its ability to (i) employ relatively small datasets for model development, (ii) iterate between model development and model assessment using small external datasets that can be either generated in focused experimental studies or formed from subsets of the initial training data, and (iii) progressively evolve models towards increasingly more reliable predictions and the identification of novel chemicals with the desired properties. In this study, we compared various AL protocols for their effectiveness in finding biologically active molecules using synthetic datasets. We have investigated the dependency of AL performance on the size of the initial training set, the relative complexity of the task, and the choice of the initial training dataset. We found that AL techniques as applied to regression modeling offer no benefits over random search, while AL used for clas-sification tasks performs better than models built for randomly selected training sets but still quite far from perfect. Using the best performing AL protocol,. Finally, the best performing AL approach was employed to discover and experimentally validate novel binding polymers for a case study of asialoglycoprotein receptor (ASGPR).
更多查看译文
关键词
Active learning,Molecular design,Bioactivity,Binders,Polymers,Polymer binders
AI 理解论文
溯源树
样例
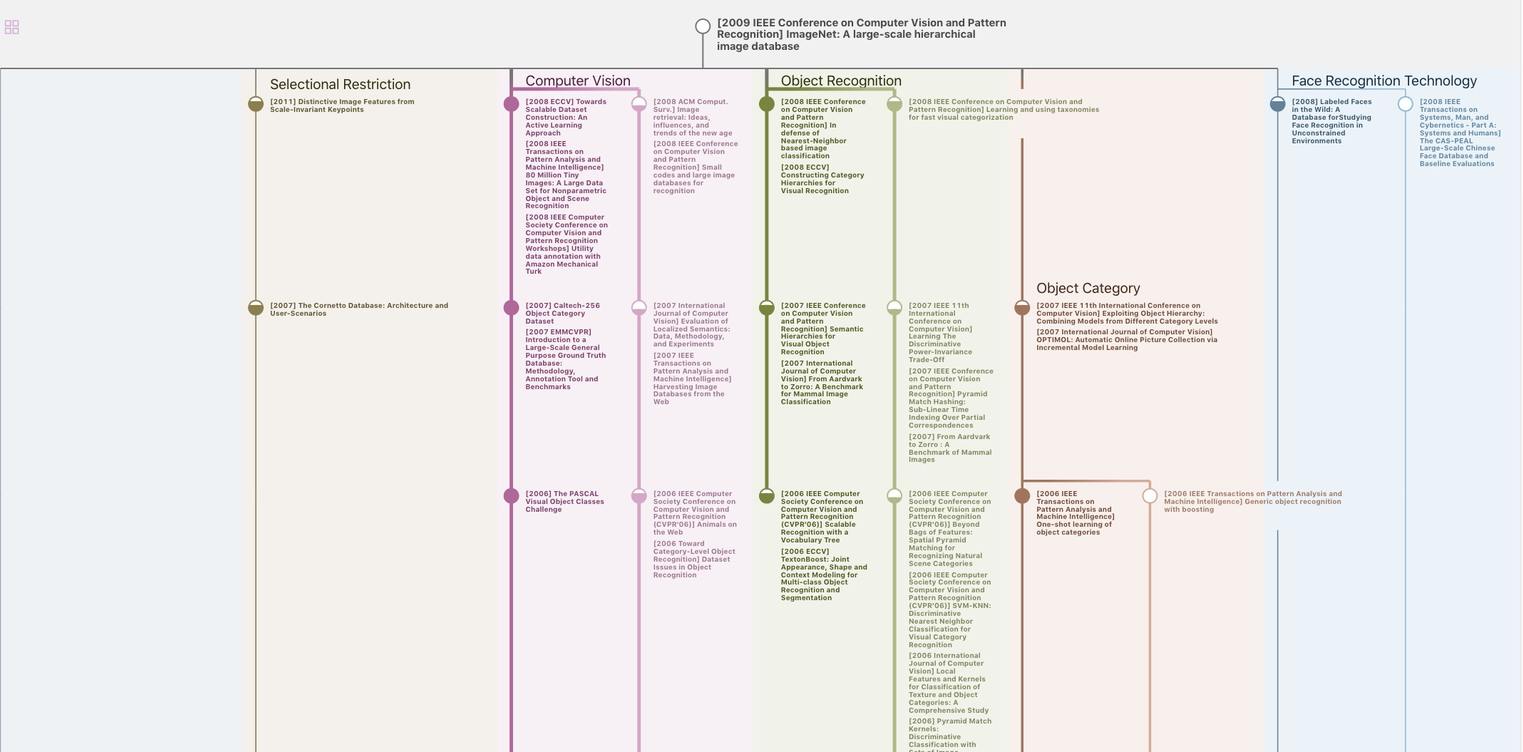
生成溯源树,研究论文发展脉络
Chat Paper
正在生成论文摘要