Learning to Model the Relationship Between Brain Structural and Functional Connectomes
IEEE Transactions on Signal and Information Processing over Networks(2022)
摘要
Recent neuroimaging advances along with algorithmic innovations in statistical learning from network data offer a unique pathway to integrate brain structure and function, and thus facilitate revealing some of the brain's organizing principles at the system level. In this direction, we develop a supervised graph representation learning framework to model the relationship between brain structural connectivity (SC) and functional connectivity (FC) via a graph encoder-decoder system, where the SC is used as input to predict empirical FC. A trainable graph convolutional encoder captures direct and indirect interactions between brain regions-of-interest that mimic actual neural communications, as well as to integrate information from both the structural network topology and nodal (i.e., region-specific) attributes. The encoder learns node-level SC embeddings which are combined to generate (whole brain) graph-level representations for reconstructing empirical FC networks. The proposed end-to-end model utilizes a multi-objective loss function to jointly reconstruct FC networks and learn discriminative graph representations of the SC-to-FC mapping for downstream subject (i.e., graph-level) classification. Comprehensive experiments demonstrate that the learnt representations of said relationship capture valuable information from the intrinsic properties of the subject's brain networks and lead to improved accuracy in classifying a large population of heavy drinkers and non-drinkers from the Human Connectome Project. Our work offers new insights on the relationship between brain networks that support the promising prospect of using graph representation learning to discover more about brain function.
更多查看译文
关键词
Brain connectomes,encoder-decoder system,graph classification,graph convolutional network,graph representation learning,graph signal processing
AI 理解论文
溯源树
样例
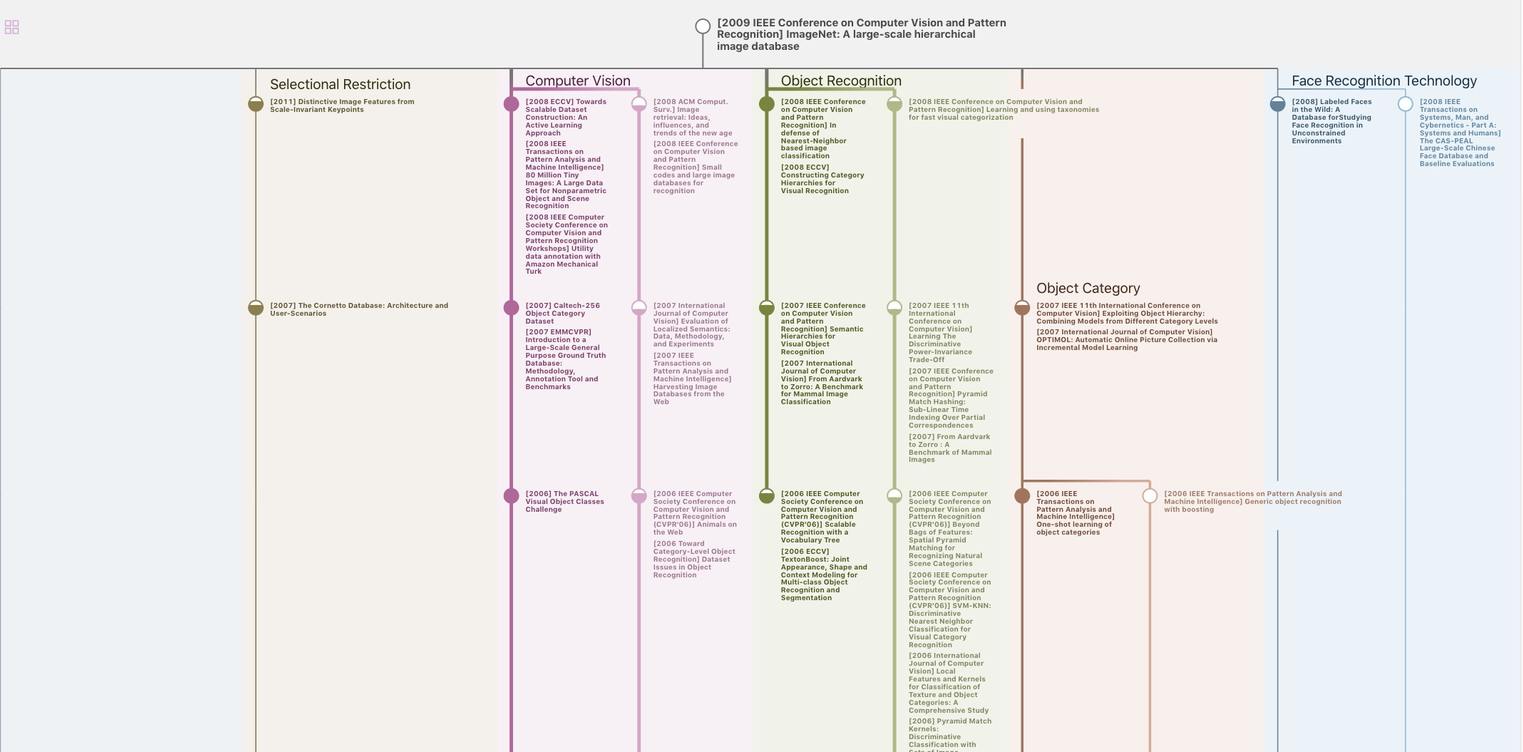
生成溯源树,研究论文发展脉络
Chat Paper
正在生成论文摘要