Scheduling HVAC loads to promote renewable generation integration with a learning-based joint chance-constrained approach
arxiv(2022)
摘要
The integration of distributed renewable generation (DRG) in distribution networks can be effectively promoted by scheduling flexible resources such as heating, ventilation, and air conditioning (HVAC) loads. However, finding the optimal scheduling for them is nontrivial because DRG outputs are highly uncertain. To address this issue, this paper proposes a learning-based joint chance-constrained approach to coordinate HVAC loads with DRG. Unlike cutting-edge works adopting individual chance constraints to manage uncertainties, this paper controls the violation probability of all critical constraints with joint chance constraints (JCCs). This joint manner can explicitly guarantee the operational security of the entire system based on operators' preferences. To overcome the intractability of JCCs, we first prove that JCCs can be safely approximated by robust constraints with proper uncertainty sets. A famous machine learning algorithm, one-class support vector clustering, is then introduced to construct a small enough polyhedron uncertainty set for these robust constraints. A linear robust counterpart is further developed based on the strong duality to ensure computational efficiency. Numerical results based on various distributed uncertainties confirm the advantages of the proposed method in optimality and feasibility.
更多查看译文
关键词
Demand-side flexibility,joint chance constraints,support vector clustering,HVAC systems,renewable energies
AI 理解论文
溯源树
样例
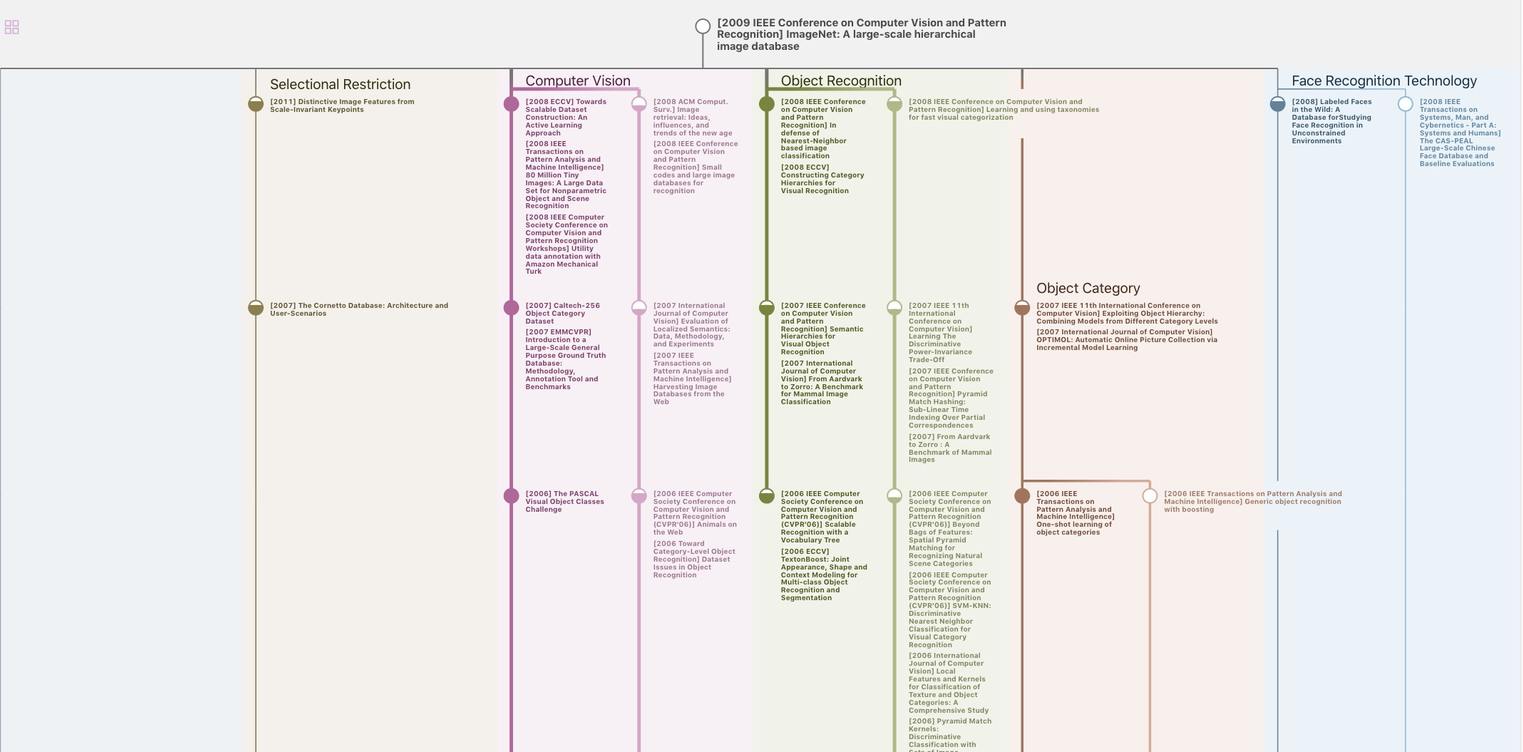
生成溯源树,研究论文发展脉络
Chat Paper
正在生成论文摘要