Towards Explainable Metaheuristics: PCA for Trajectory Mining in Evolutionary Algorithms
ARTIFICIAL INTELLIGENCE XXXVIII(2021)
摘要
The generation of explanations regarding decisions made by population-based meta-heuristics is often a difficult task due to the nature of the mechanisms employed by these approaches. With the increase in use of these methods for optimisation in industries that require end-user confirmation, the need for explanations has also grown. We present a novel approach to the extraction of features capable of supporting an explanation through the use of trajectory mining - extracting key features from the populations of NDAs. We apply Principal Components Analysis techniques to identify new methods of population diversity tracking post-runtime after projection into a lower dimensional space. These methods are applied to a set of benchmark problems solved by a Genetic Algorithm and a Univariate Estimation of Distribution Algorithm. We show that the new sub-space derived metrics can capture key learning steps in the algorithm run and how solution variable patterns that explain the fitness function may be captured in the principal component coefficients.
更多查看译文
关键词
Evolutionary algorithms, PCA, Explainability, Population diversity
AI 理解论文
溯源树
样例
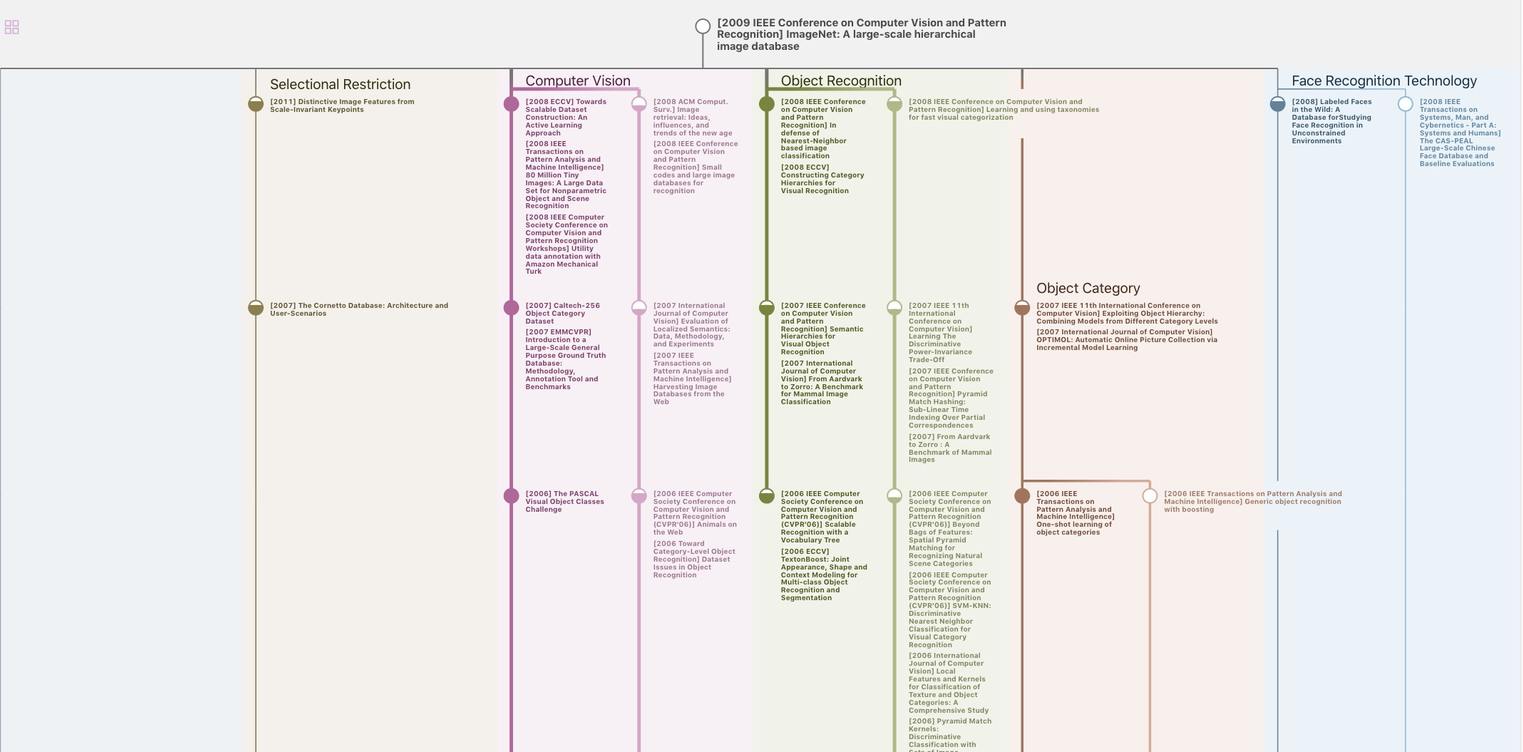
生成溯源树,研究论文发展脉络
Chat Paper
正在生成论文摘要