Adaptively Customizing Activation Functions for Various Layers
IEEE Transactions on Neural Networks and Learning Systems(2023)
Abstract
To enhance the nonlinearity of neural networks and increase their mapping abilities between the inputs and response variables, activation functions play a crucial role to model more complex relationships and patterns in the data. In this work, a novel methodology is proposed to adaptively customize activation functions only by adding very few parameters to the traditional activation functions such as Sigmoid, Tanh, and rectified linear unit (ReLU). To verify the effectiveness of the proposed methodology, some theoretical and experimental analysis on accelerating the convergence and improving the performance is presented, and a series of experiments are conducted based on various network models (such as AlexNet, VggNet, GoogLeNet, ResNet and DenseNet), and various datasets (such as CIFAR10, CIFAR100, miniImageNet, PASCAL VOC, and COCO). To further verify the validity and suitability in various optimization strategies and usage scenarios, some comparison experiments are also implemented among different optimization strategies (such as SGD, Momentum, AdaGrad, AdaDelta, and ADAM) and different recognition tasks such as classification and detection. The results show that the proposed methodology is very simple but with significant performance in convergence speed, precision, and generalization, and it can surpass other popular methods such as ReLU and adaptive functions such as Swish in almost all experiments in terms of overall performance.
MoreTranslated text
Key words
Neural networks,Training,Shape,Adaptive systems,Mathematical models,Learning systems,Deep learning,Adaptable parameters,adaptive activation function,deep learning,various layers
AI Read Science
Must-Reading Tree
Example
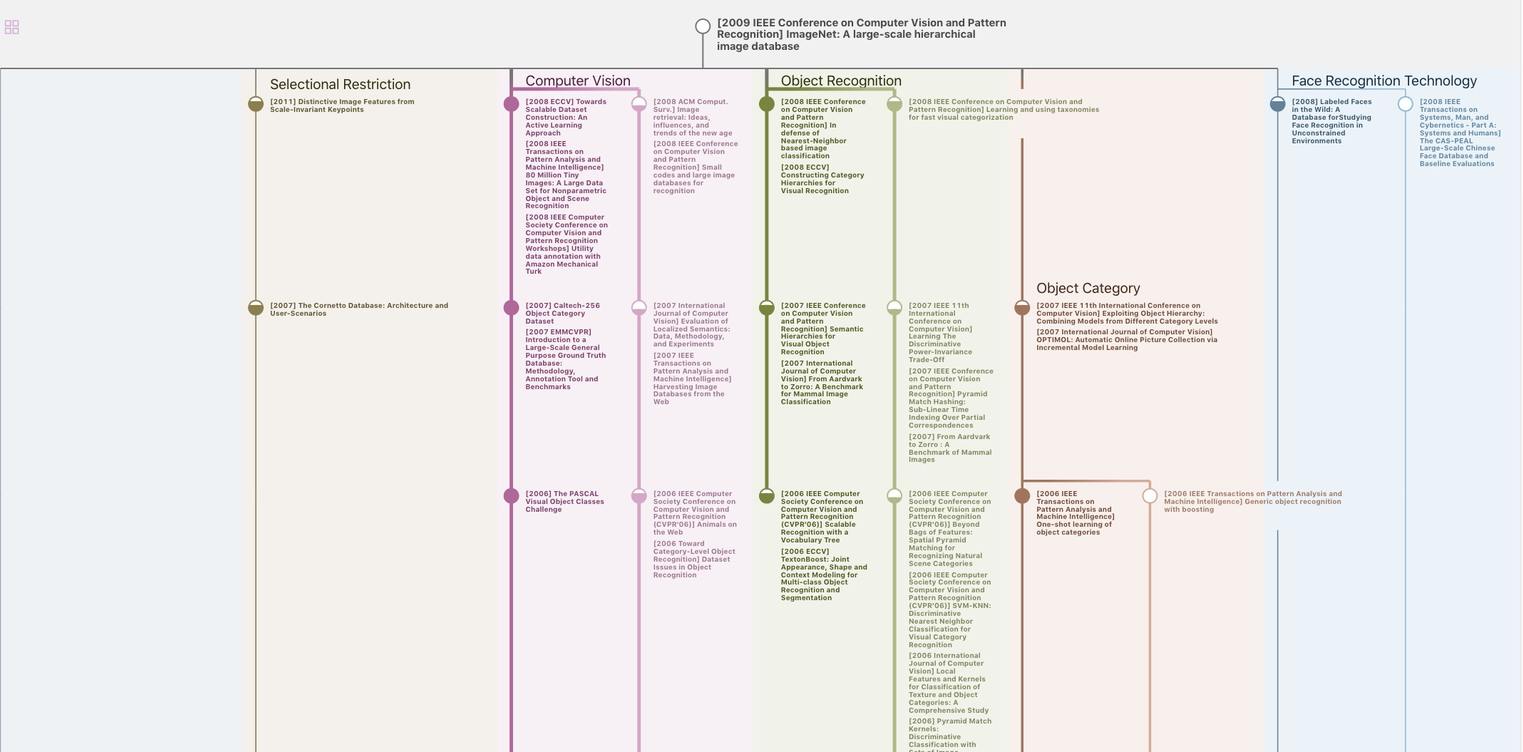
Generate MRT to find the research sequence of this paper
Chat Paper
Summary is being generated by the instructions you defined