Automated Deep Learning: Neural Architecture Search Is Not the End
FOUNDATIONS AND TRENDS IN MACHINE LEARNING(2024)
Abstract
Deep learning (DL) has proven to be a highly effective approach for developing models in diverse contexts, including visual perception, speech recognition, and machine translation. However, the end-to-end process for applying DL is not trivial. It requires grappling with problem formulation and context understanding, data engineering, model development, deployment, continuous monitoring and maintenance, and so on. Moreover, each of these steps typically relies heavily on humans, in terms of both knowledge and interactions, which impedes the further advancement and democratization of DL. Consequently, in response to these issues, a new field has emerged over the last few years: automated deep learning (AutoDL). This endeavor seeks to minimize the need for human involvement and is best known for its achievements in neural architecture search (NAS), a topic that has been the focus of several surveys. That stated, NAS is not the be-all and end-all of AutoDL. Accordingly, this review adopts an overarching perspective, examining research efforts into automation across the entirety of an archetypal DL workflow. In so doing, this work also proposes a comprehensive set of ten criteria by which to assess existing work in both individual publications and broader research areas. These criteria are: novelty, solution quality, efficiency, stability, interpretability, reproducibility, engineering quality, scalability, generalizability, and eco-friendliness. Thus, ultimately, this review provides an evaluative overview of AutoDL in the early 2020s, identifying where future opportunities for progress may exist.
MoreTranslated text
Key words
Automated deep learning (AutoDL),neural architecture search (NAS),hyperparameter optimization (HPO),automated data engineering,hardware search,automated deployment,life-long learning,persistent learning,adaptation,automated machine learning (AutoML),autonomous machine learning (AutonoML),deep neural networks,deep learning
AI Read Science
Must-Reading Tree
Example
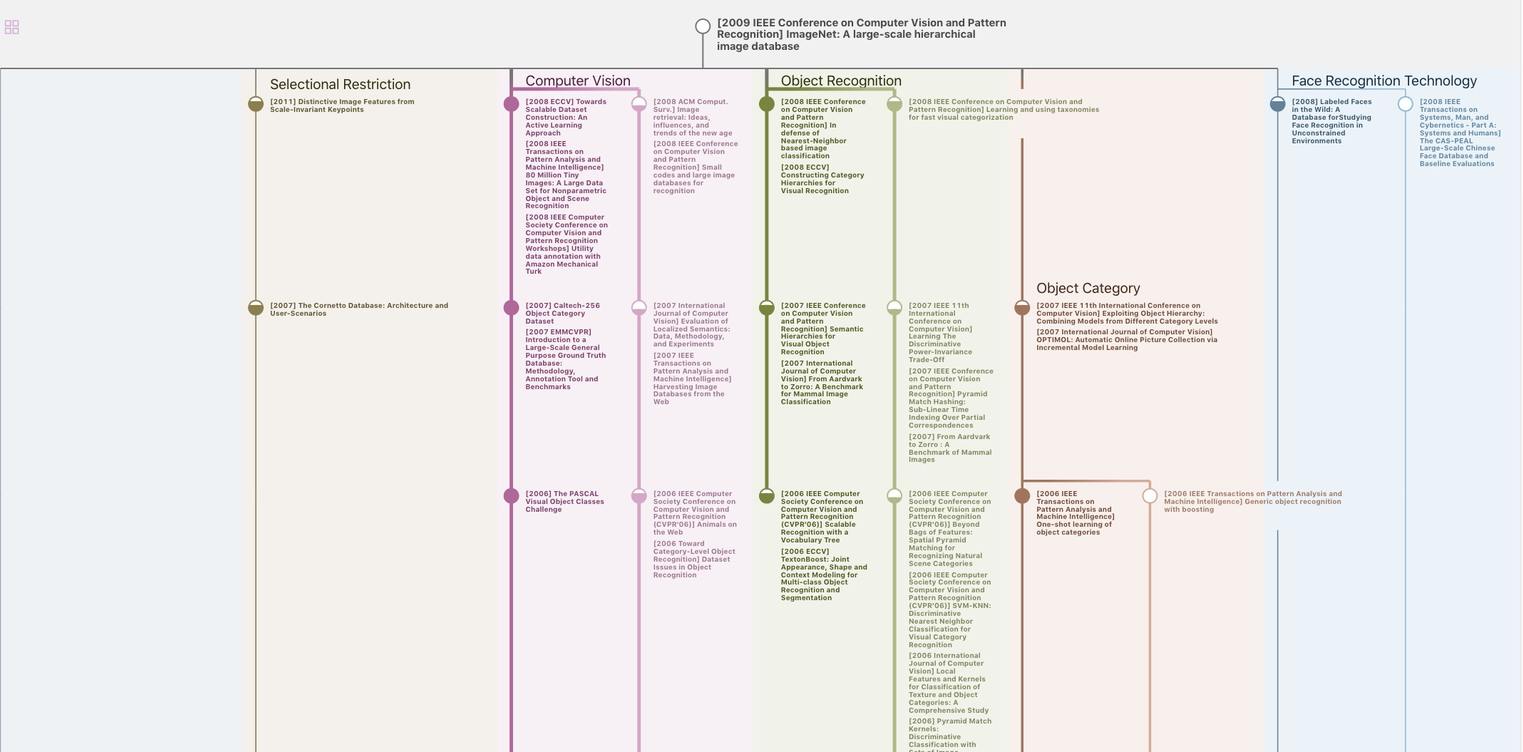
Generate MRT to find the research sequence of this paper
Chat Paper
Summary is being generated by the instructions you defined