Two-view Graph Neural Networks for Knowledge Graph Completion
arxiv(2023)
摘要
We present an effective graph neural network (GNN)-based knowledge graph embedding model, which we name WGE, to capture entity- and relation-focused graph structures. Given a knowledge graph, WGE builds a single undirected entity-focused graph that views entities as nodes. WGE also constructs another single undirected graph from relation-focused constraints, which views entities and relations as nodes. WGE then proposes a GNN-based architecture to better learn vector representations of entities and relations from these two single entity- and relation-focused graphs. WGE feeds the learned entity and relation representations into a weighted score function to return the triple scores for knowledge graph completion. Experimental results show that WGE outperforms strong baselines on seven benchmark datasets for knowledge graph completion.
更多查看译文
关键词
knowledge graph completion,networks,two-view
AI 理解论文
溯源树
样例
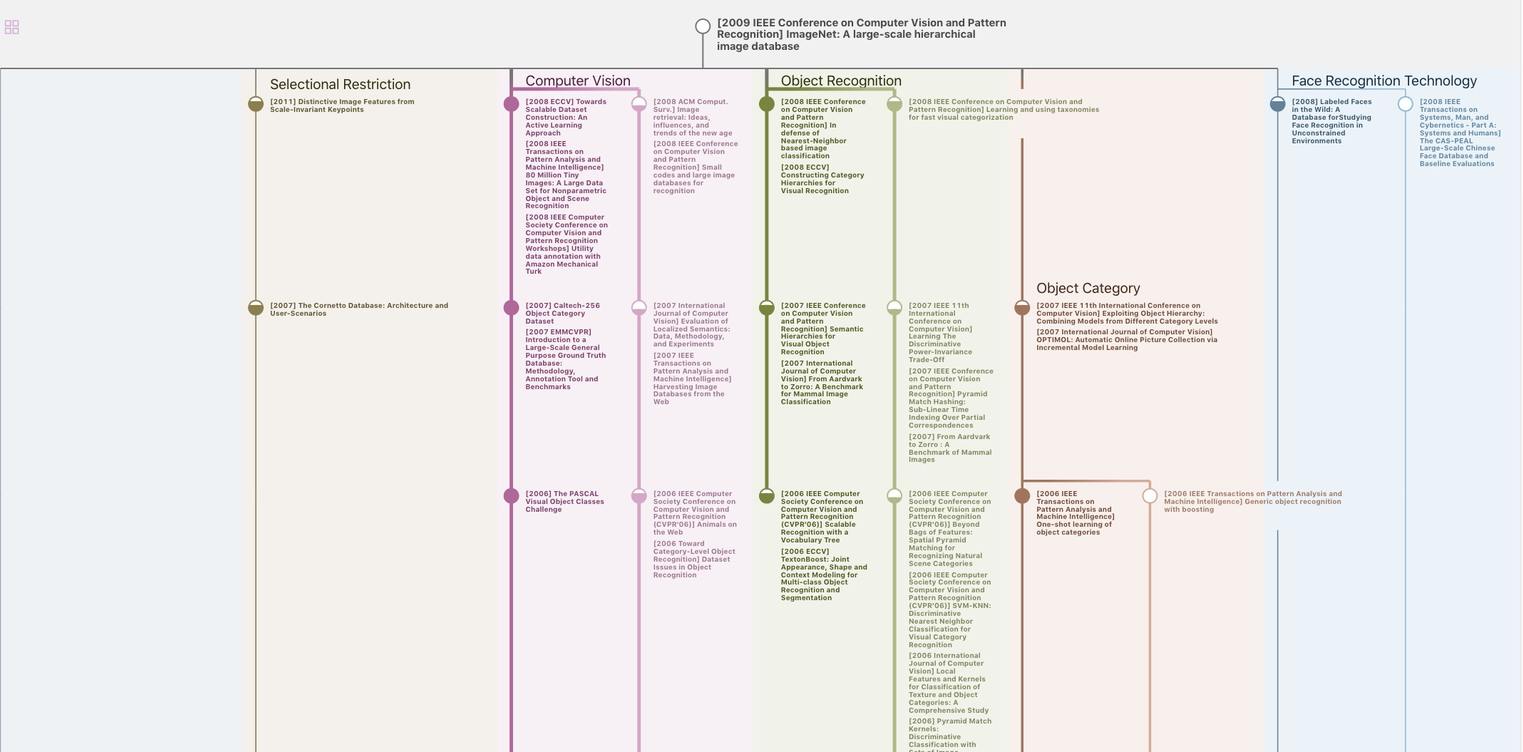
生成溯源树,研究论文发展脉络
Chat Paper
正在生成论文摘要