Targeted proteomics for evaluating risk of venous thrombosis following traumatic lower‐leg injury or knee arthroscopy
Journal of Thrombosis and Haemostasis(2022)
摘要
Introduction Patients with lower-leg cast immobilization and patients undergoing knee arthroscopy have an increased risk of venous thrombosis (VT). Guidelines are ambiguous about thromboprophylaxis use, and individual risk factors for developing VT are often ignored. To assist in VT risk stratification and guide thromboprophylaxis use, various prediction models have been developed. These models depend largely on clinical factors and provide reasonably good C-statistics of around 70%. We explored using protein levels in blood plasma measured by multiplexed quantitative targeted proteomics to predict VT. Our aim was to assess whether a VT risk prediction model based on absolute plasma protein quantification is possible. Methods We used internal standards to quantify proteins in less than 10 mu l plasma. We measured 270 proteins in samples from patients scheduled for knee arthroscopy or with lower-leg cast immobilization. The two prospective POT-(K)CAST trails allow complementary views of VT signature in blood, namely pre and post trauma, respectively. From approximately 3000 patients, 31 patients developed VT who were included and matched with double the number of controls. Results Top discriminating proteins between cases and controls included APOC3, APOC4, APOC2, ATRN, F13B, and F2 in knee arthroscopy patients and APOE, SERPINF2, B2M, F13B, AFM, and C1QC in patients with lower-leg cast. A logistic regression model with cross-validation resulted in C-statistics of 88.1% (95% CI: 85.7-90.6%) and 79.6% (95% CI: 77.2-82.0%) for knee arthroscopy and cast immobilization groups respectively. Conclusions Promising C-statistics merit further exploration of the value of proteomic tests for predicting VT risk upon additional validation.
更多查看译文
关键词
blood proteins,prediction,proteomics,quantitative evaluation,reference standards,venous thrombosis
AI 理解论文
溯源树
样例
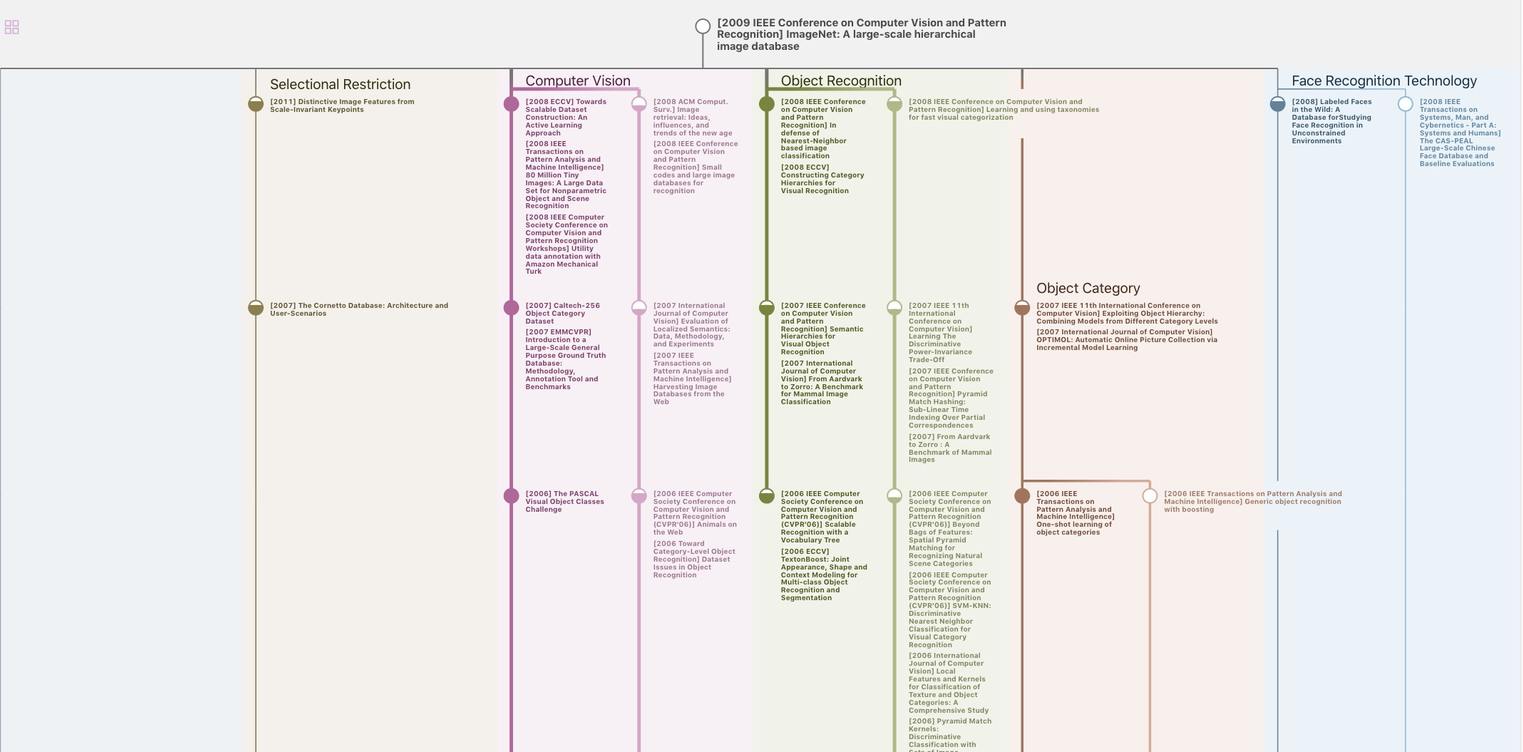
生成溯源树,研究论文发展脉络
Chat Paper
正在生成论文摘要