Machine Learning Identifies Digital Phenotyping Measures Most Relevant to Negative Symptoms in Psychotic Disorders: Implications for Clinical Trials.
SCHIZOPHRENIA BULLETIN(2022)
摘要
BACKGROUND:Digital phenotyping has been proposed as a novel assessment tool for clinical trials targeting negative symptoms in psychotic disorders (PDs). However, it is unclear which digital phenotyping measurements are most appropriate for this purpose.
AIMS:Machine learning was used to address this gap in the literature and determine whether: (1) diagnostic status could be classified from digital phenotyping measures relevant to negative symptoms and (2) the 5 negative symptom domains (anhedonia, avolition, asociality, alogia, and blunted affect) were differentially classified by active and passive digital phenotyping variables.
METHODS:Participants included 52 outpatients with a PD and 55 healthy controls (CN) who completed 6 days of active (ecological momentary assessment surveys) and passive (geolocation, accelerometry) digital phenotyping data along with clinical ratings of negative symptoms.
RESULTS:Machine learning algorithms classifying the presence of a PD diagnosis yielded 80% accuracy for cross-validation in H2O AutoML and 79% test accuracy in the Recursive Feature Elimination with Cross Validation feature selection model. Models classifying the presence vs absence of clinically significant elevations on each of the 5 negative symptom domains ranged in test accuracy from 73% to 91%. A few active and passive features were highly predictive of all 5 negative symptom domains; however, there were also unique predictors for each domain.
CONCLUSIONS:These findings suggest that negative symptoms can be modeled from digital phenotyping data recorded in situ. Implications for selecting the most appropriate digital phenotyping variables for use as outcome measures in clinical trials targeting negative symptoms are discussed.
更多查看译文
关键词
ecological momentary assessment, schizophrenia, negative symptoms, machine learning, classification, feature selection, digital phenotyping
AI 理解论文
溯源树
样例
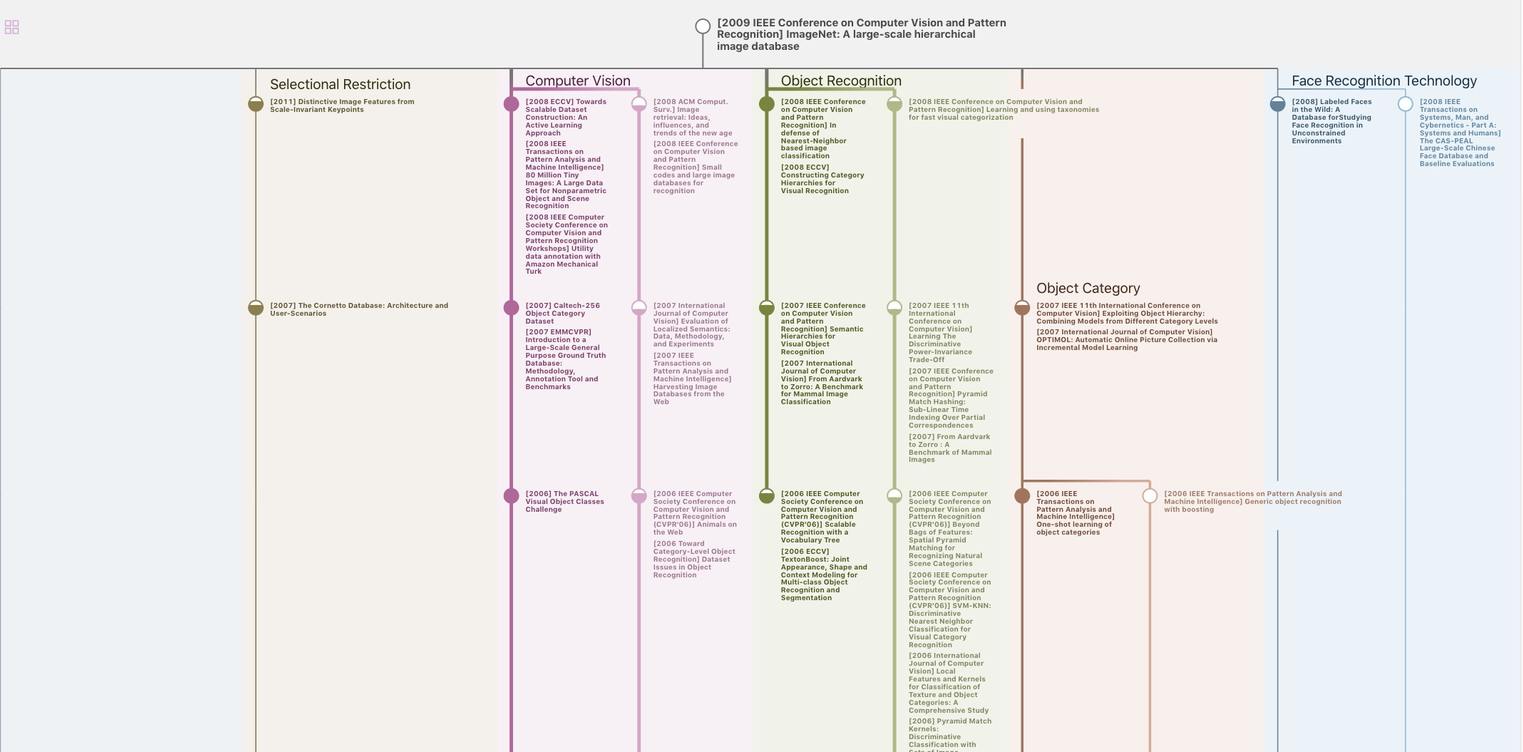
生成溯源树,研究论文发展脉络
Chat Paper
正在生成论文摘要