seGMM: a new tool to infer sex from massively parallel sequencing data
biorxiv(2021)
摘要
Inspecting concordance between self-reported sex and genotype-inferred sex from genomic data is a significant quality control measure in clinical genetic testing. Numerous tools have been developed to infer sex for genotyping array, whole-exome sequencing, and whole-genome sequencing data. However, improvements in sex inference from targeted gene sequencing panels are warranted. Here, we propose a new tool, seGMM, which applies unsupervised clustering (Gaussian Mixture Model) to determine the gender of a sample from the called genotype data integrated aligned reads. seGMM consistently demonstrated >99% sex inference accuracy in publicly available (1000 Genomes) and our in-house panel dataset, which achieved obviously better sex classification than existing popular tools. Compared to including features only in the X chromosome, our results show that adding additional features from Y chromosomes (e.g. reads mapped to the Y chromosome) can increase sex classification accuracy. Notably, for WES and WGS data, seGMM also has an extremely high degree of accuracy. Finally, we proved the ability of seGMM to infer sex in single patient or trio samples by combining with reference data and pinpointing potential sex chromosome abnormality samples. In general, seGMM provides a reproducible framework to infer sex from massively parallel sequencing data and has great promise in clinical genetics.
### Competing Interest Statement
The authors have declared no competing interest.
更多查看译文
关键词
parallel,data
AI 理解论文
溯源树
样例
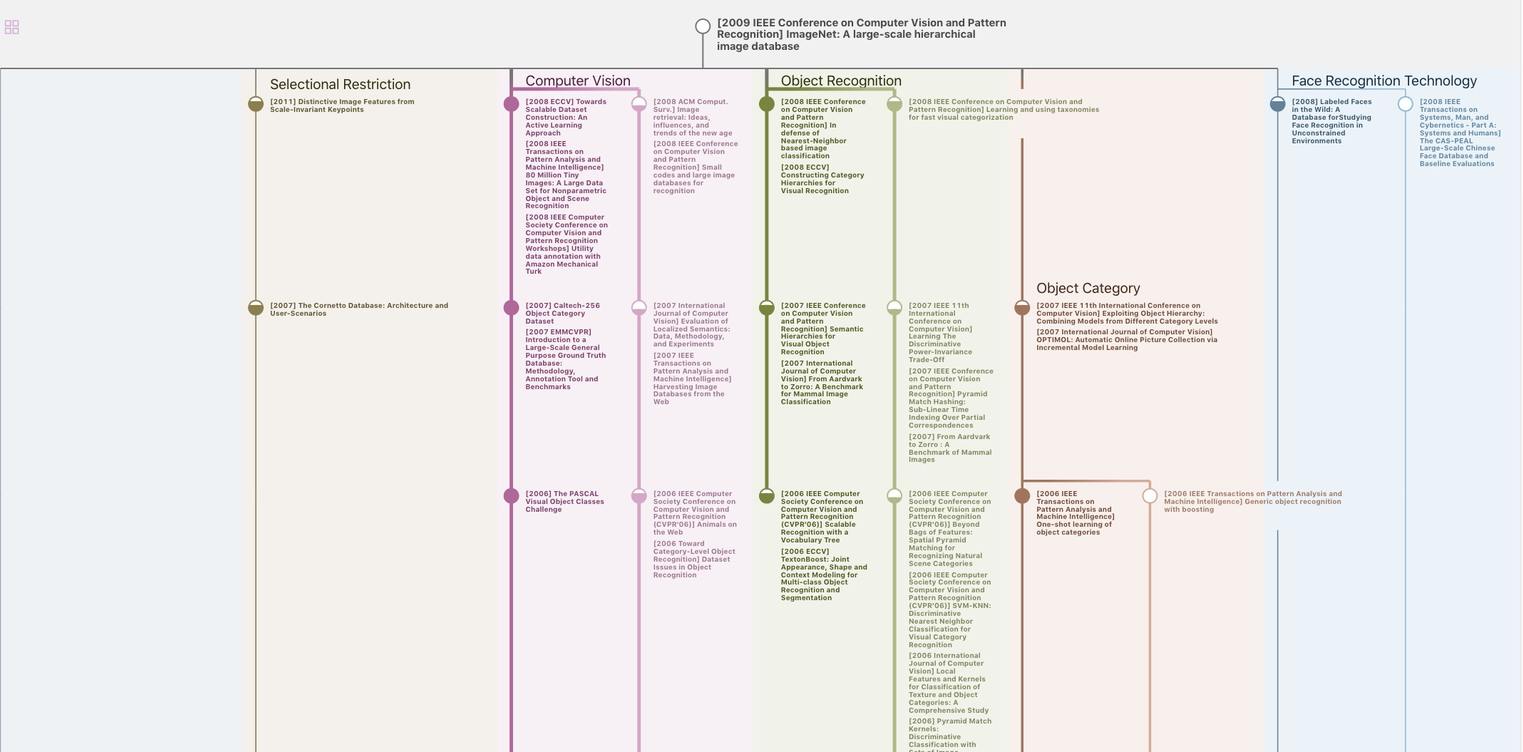
生成溯源树,研究论文发展脉络
Chat Paper
正在生成论文摘要