A Static Analyzer for Detecting Tensor Shape Errors in Deep Neural Network Training Code
2022 IEEE/ACM 44th International Conference on Software Engineering: Companion Proceedings (ICSE-Companion)(2022)
摘要
We present an automatic static analyzer PyTea that detects tensorshape errors in PyTorch code. The tensor-shape error is critical in the deep neural net code; much of the training cost and intermediate results are to be lost once a tensor shape mismatch occurs in the midst of the training phase. Given the input PyTorch source, PyTea statically traces every possible execution path, collects tensor shape constraints required by the tensor operation sequence of the path, and decides if the constraints are unsatisfiable (hence a shape error can occur). PyTea’s scalability and precision hinges on the characteristics of real-world PyTorch applications: the number of execution paths after PyTea’s conservative pruning rarely explodes and loops are simple enough to be circumscribed by our symbolic abstraction. We tested PyTea against the projects in the official PyTorch repository and some tensor-error code questioned in the StackOverflow. PyTea successfully detects tensor shape errors in these codes, each within a few seconds.
更多查看译文
关键词
Software and its engineering → Software testing and debugging
AI 理解论文
溯源树
样例
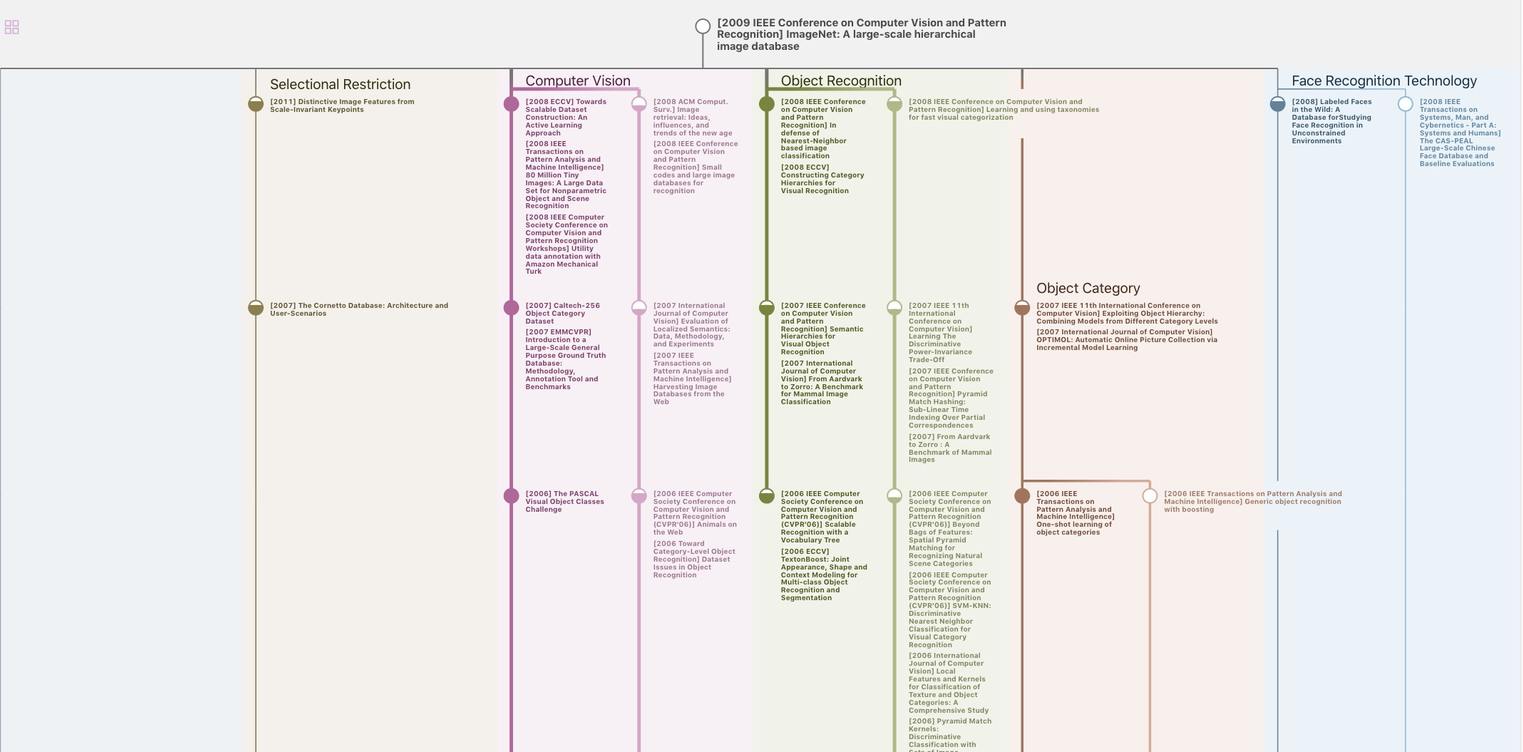
生成溯源树,研究论文发展脉络
Chat Paper
正在生成论文摘要