Prompt Tuning GPT-2 language model for parameter-efficient domain adaptation of ASR systems
arxiv(2022)
摘要
Automatic Speech Recognition (ASR) systems have found their use in numerous industrial applications in very diverse domains creating a need to adapt to new domains with small memory and deployment overhead. In this work, we introduce domain-prompts, a methodology that involves training a small number of domain embedding parameters to prime a Transformer-based Language Model (LM) to a particular domain. Using this domain-adapted LM for rescoring ASR hypotheses can achieve 7-13% WER reduction for a new domain with just 1000 unlabeled textual domain-specific sentences. This improvement is comparable or even better than fully fine-tuned models even though just 0.02% of the parameters of the base LM are updated. Additionally, our method is deployment-friendly as the learnt domain embeddings are prefixed to the input to the model rather than changing the base model architecture. Therefore, our method is an ideal choice for on-the-fly adaptation of LMs used in ASR systems to progressively scale it to new domains.
更多查看译文
关键词
adaptation,tuning,parameter-efficient
AI 理解论文
溯源树
样例
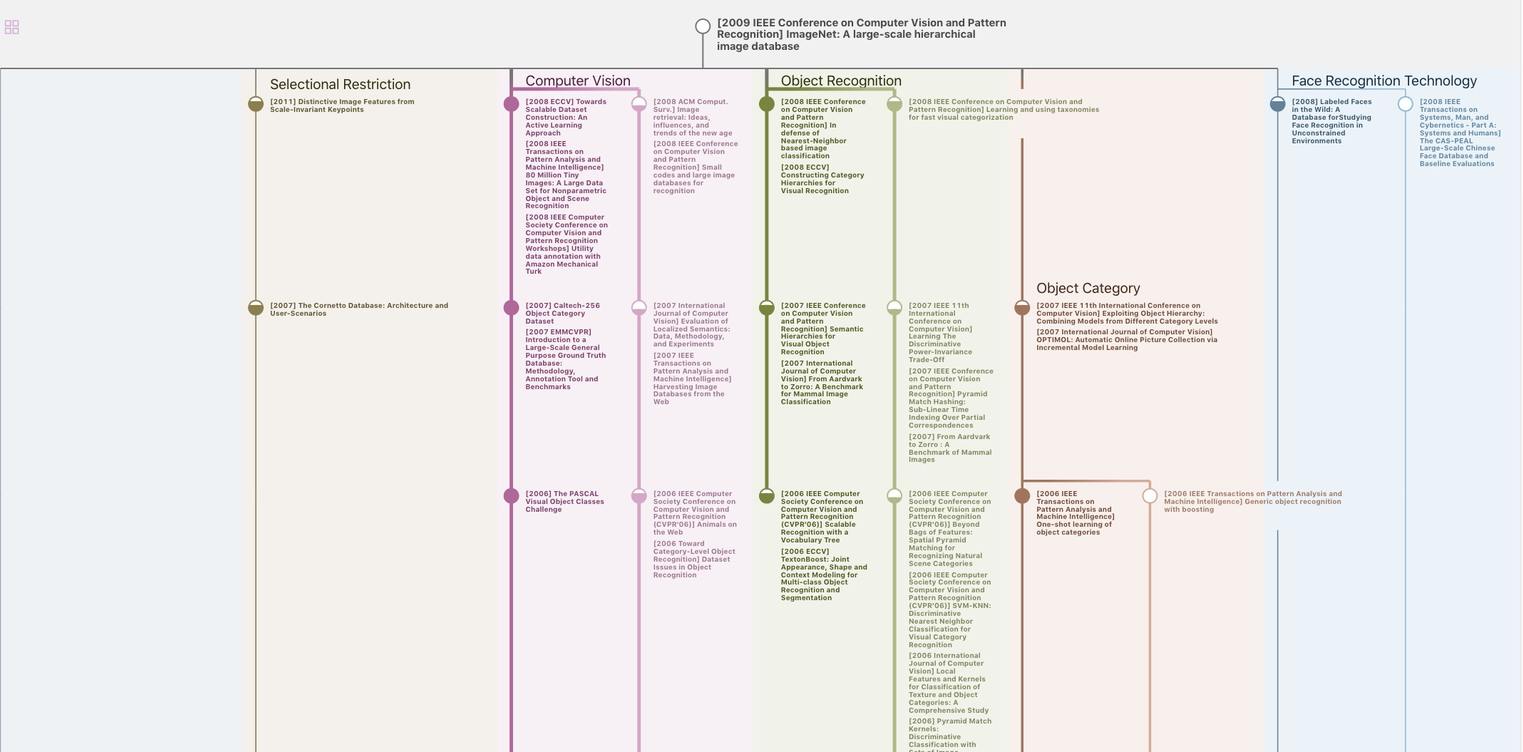
生成溯源树,研究论文发展脉络
Chat Paper
正在生成论文摘要