Learning Interpretable Models Through Multi-Objective Neural Architecture Search
CoRR(2021)
Key words
Machine Learning Interpretability,Interpretable Models,Model Interpretability,Robust Learning,Deep Learning
AI Read Science
Must-Reading Tree
Example
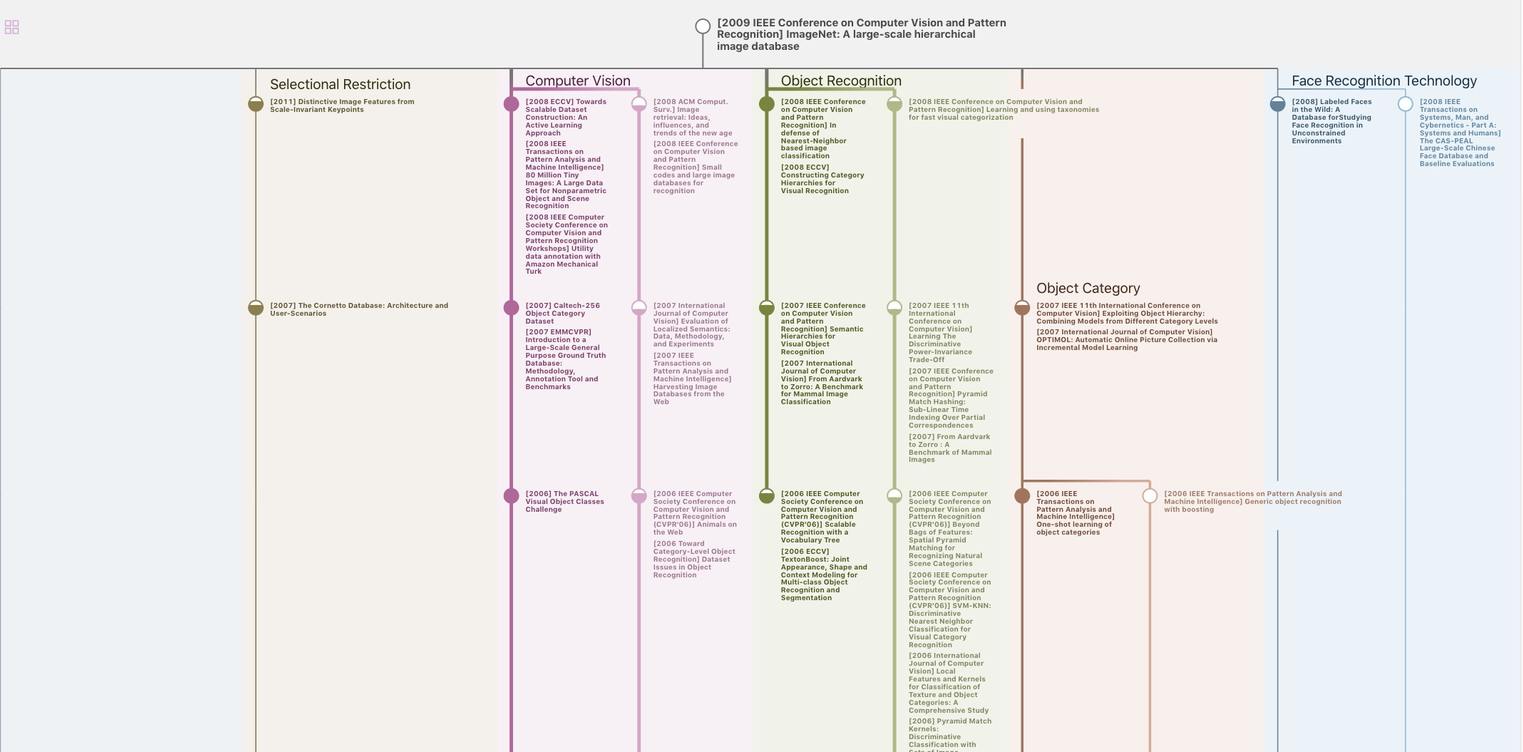
Generate MRT to find the research sequence of this paper
Chat Paper
Summary is being generated by the instructions you defined