Implicit Neural Representations for Deconvolving SAS Images
arxiv(2021)
摘要
Synthetic aperture sonar (SAS) image resolution is constrained by waveform bandwidth and array geometry. Specifically, the waveform bandwidth determines a point spread function (PSF) that blurs the locations of point scatterers in the scene. In theory, deconvolving the reconstructed SAS image with the scene PSF restores the original distribution of scatterers and yields sharper reconstructions. However, deconvolution is an ill-posed operation that is highly sensitive to noise. In this work, we leverage implicit neural representations (INRs), shown to be strong priors for the natural image space, to deconvolve SAS images. Importantly, our method does not require training data, as we perform our deconvolution through an analysis-bysynthesis optimization in a self-supervised fashion. We validate our method on simulated SAS data created with a point scattering model and real data captured with an in-air circular SAS. This work is an important first step towards applying neural networks for SAS image deconvolution.
更多查看译文
关键词
SAS images,synthetic aperture sonar image resolution,waveform bandwidth,array geometry,point spread function,point scatterers,reconstructed SAS image,scene PSF,original distribution,yields sharper reconstructions,leverage implicit neural representations,strong priors,natural image space,training data,analysis-by-synthesis optimization,point scattering model,neural networks,SAS image deconvolution
AI 理解论文
溯源树
样例
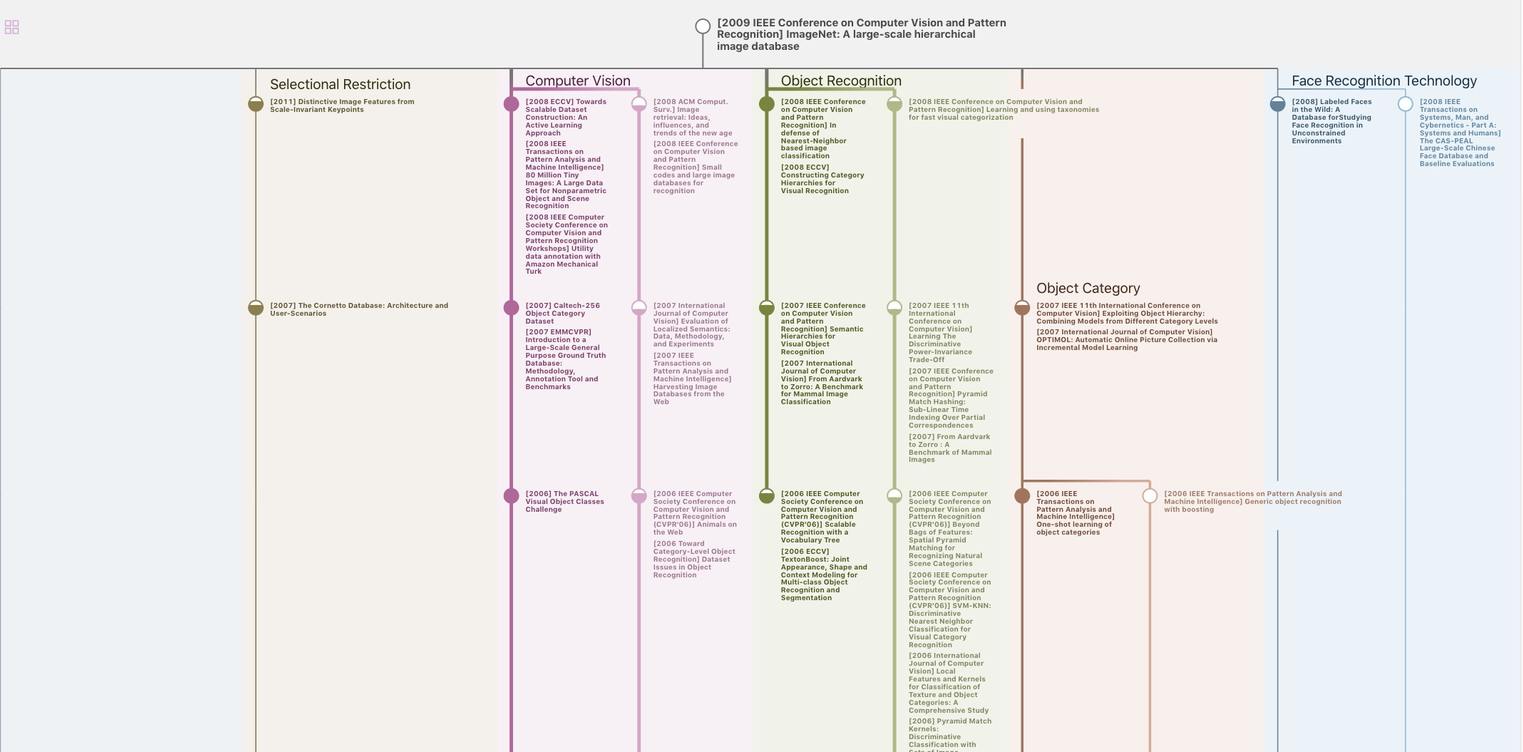
生成溯源树,研究论文发展脉络
Chat Paper
正在生成论文摘要